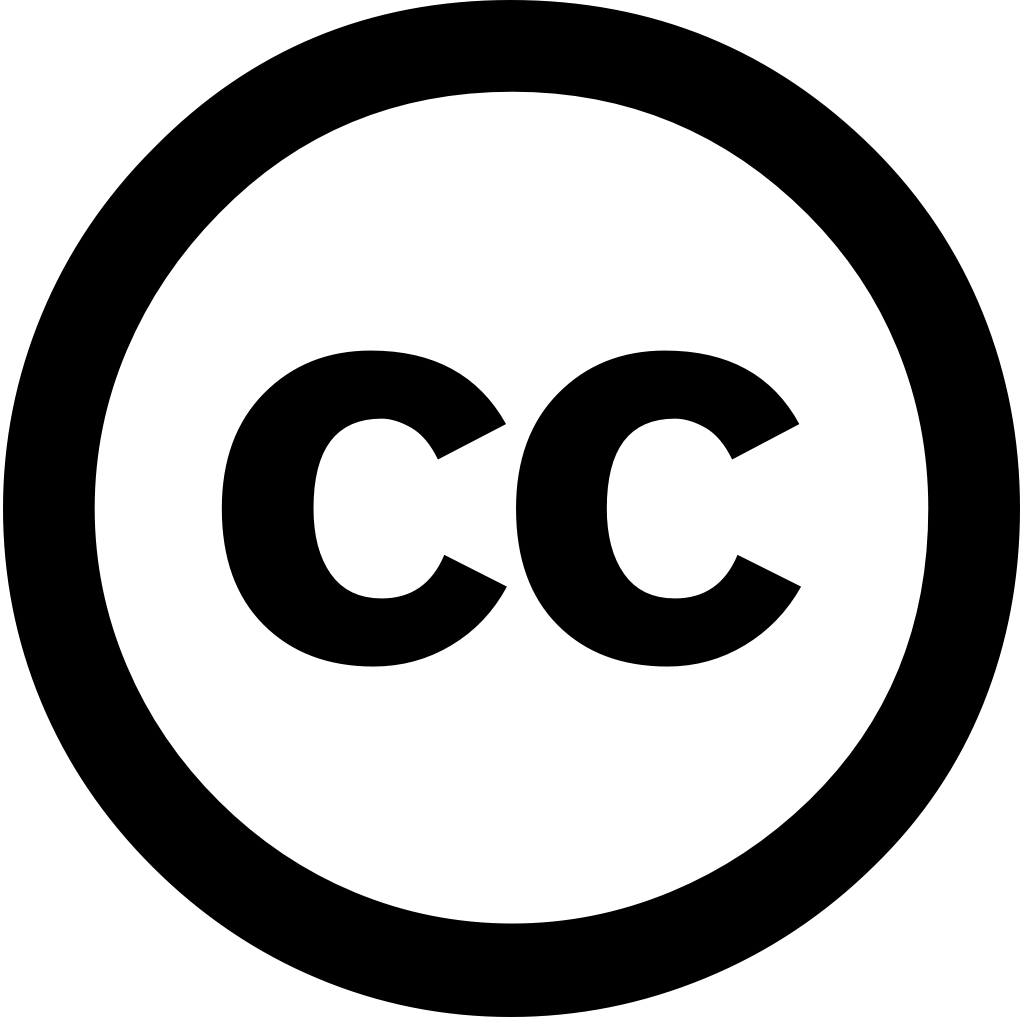
Mehran University Research Journal of Engineering and Technology, Journal Year: 2025, Volume and Issue: 44(2), P. 93 - 116
Published: April 9, 2025
Due to the tremendous value embedded in big educational data, numerous research institutes have collected large volumes of student behavioral data. To fully utilize underlying values, data may be shared with third parties, such as worldwide intelligent experts. However, this pose privacy risks owners, even though collectors usually anonymize before crowdsourcing. demonstrate that anonymization alone is insufficient protect user privacy, we conducted an experimental study using offline and online traces through campus cards smartphones. Our demonstrates a student’s identity can identified high probability based on anonymized behavior payment traces. The analysis results only ten features, i.e., Transmission Control Protocol (TCP), synchronization attempts, content length, downlink traffic, last acknowledgement packet delay, uplink cell ID, base station day, hour (offline payment, time) hour, minute (online time), point sale ID (POS_ID) are sufficient uniquely identify individual. Five supervised standard learning algorithm classifiers been utilized predict Extra Tree, Bagging, Decision Nearest Neighbor (KNN), Random Forest Tree classifiers. evaluation showed achieved accuracy reached 99.99%, 99.95%, 99.02%, 98.84%, 99.56%, respectively.
Language: Английский