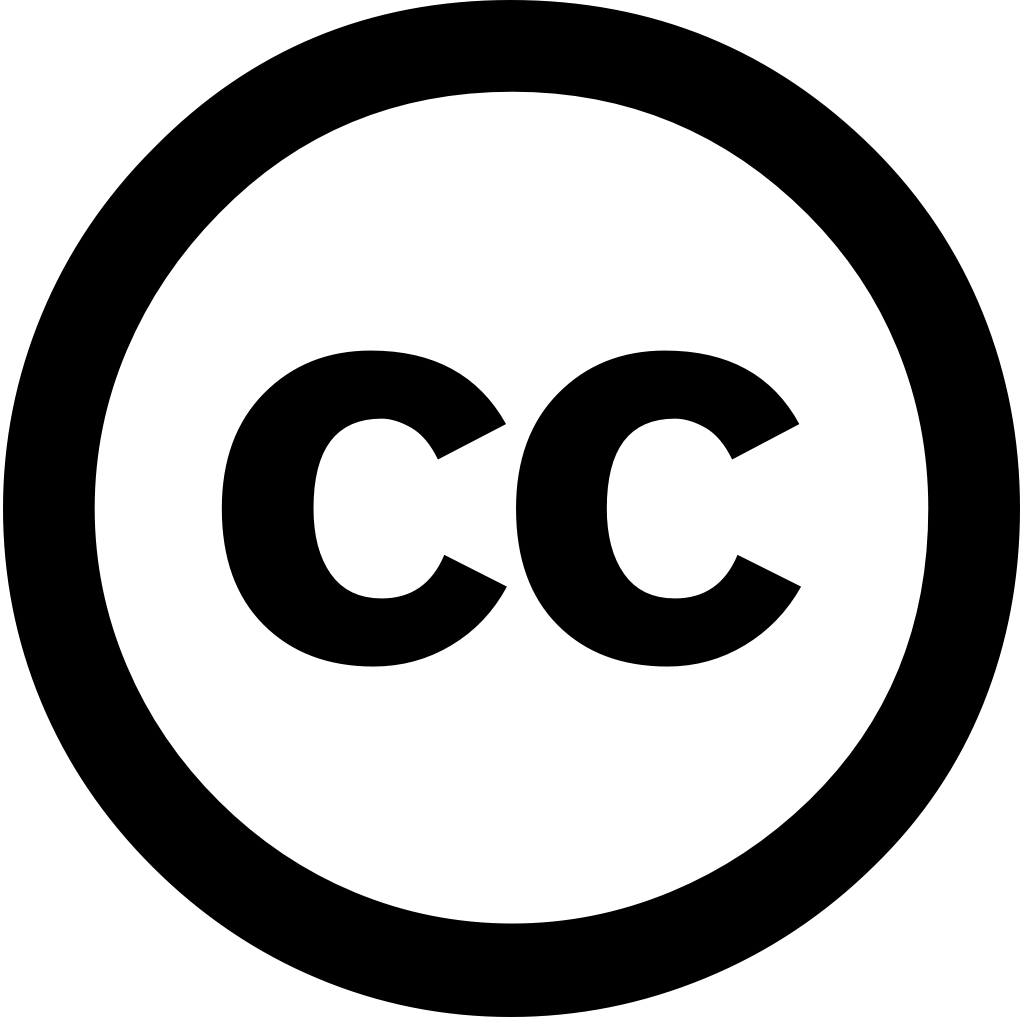
Artificial Intelligence Review, Journal Year: 2025, Volume and Issue: 58(8)
Published: May 13, 2025
Language: Английский
Artificial Intelligence Review, Journal Year: 2025, Volume and Issue: 58(8)
Published: May 13, 2025
Language: Английский
Information, Journal Year: 2025, Volume and Issue: 16(3), P. 244 - 244
Published: March 18, 2025
Federated learning (FL) is a machine technique where clients exchange only local model updates with central server that combines them to create global after training. While FL offers privacy benefits through training, privacy-preserving strategies are needed since can leak training data information due various attacks. To enhance and attack robustness, techniques like homomorphic encryption (HE), Secure Multi-Party Computation (SMPC), the Private Aggregation of Teacher Ensembles (PATE) be combined FL. Currently, no study has more than two or comparatively analyzed their combinations. We conducted comparative in FL, analyzing performance security. implemented using an artificial neural network (ANN) Malware Dataset from Kaggle for malware detection. privacy, we proposed models combining PATE, SMPC, HE. All were evaluated against poisoning attacks (targeted untargeted), backdoor attack, inversion man middle attack. The maintained while improving robustness. FL_SMPC, FL_CKKS, FL_CKKS_SMPC improved both resistance. outperformed base FL_PATE_CKKS_SMPC achieved lowest success rate (0.0920). best resisted untargeted (0.0010 rate). FL_CKKS defended targeted (0.0020 FL_PATE_SMPC (19.267 MSE). degradation accuracy (1.68%), precision (1.94%), recall F1-score (1.64%).
Language: Английский
Citations
0Scientific Reports, Journal Year: 2025, Volume and Issue: 15(1)
Published: April 16, 2025
Abstract In the digital age, privacy preservation is of paramount importance while processing health-related sensitive information. This paper explores integration Federated Learning (FL) and Differential Privacy (DP) for breast cancer detection, leveraging FL’s decentralized architecture to enable collaborative model training across healthcare organizations without exposing raw patient data. To enhance privacy, DP injects statistical noise into updates made by model. mitigates adversarial attacks prevents data leakage. The proposed work uses Breast Cancer Wisconsin Diagnostic dataset address critical challenges such as heterogeneity, privacy-accuracy trade-offs, computational overhead. From experimental results, FL combined with achieves 96.1% accuracy a budget ε = 1.9, ensuring strong minimal performance trade-offs. comparison, traditional non-FL achieved 96.0% accuracy, but at cost requiring centralized storage, which poses significant risks. These findings validate feasibility privacy-preserving artificial intelligence models in real-world clinical applications, effectively balancing protection reliable medical predictions.
Language: Английский
Citations
0Artificial Intelligence Review, Journal Year: 2025, Volume and Issue: 58(8)
Published: May 13, 2025
Language: Английский
Citations
0