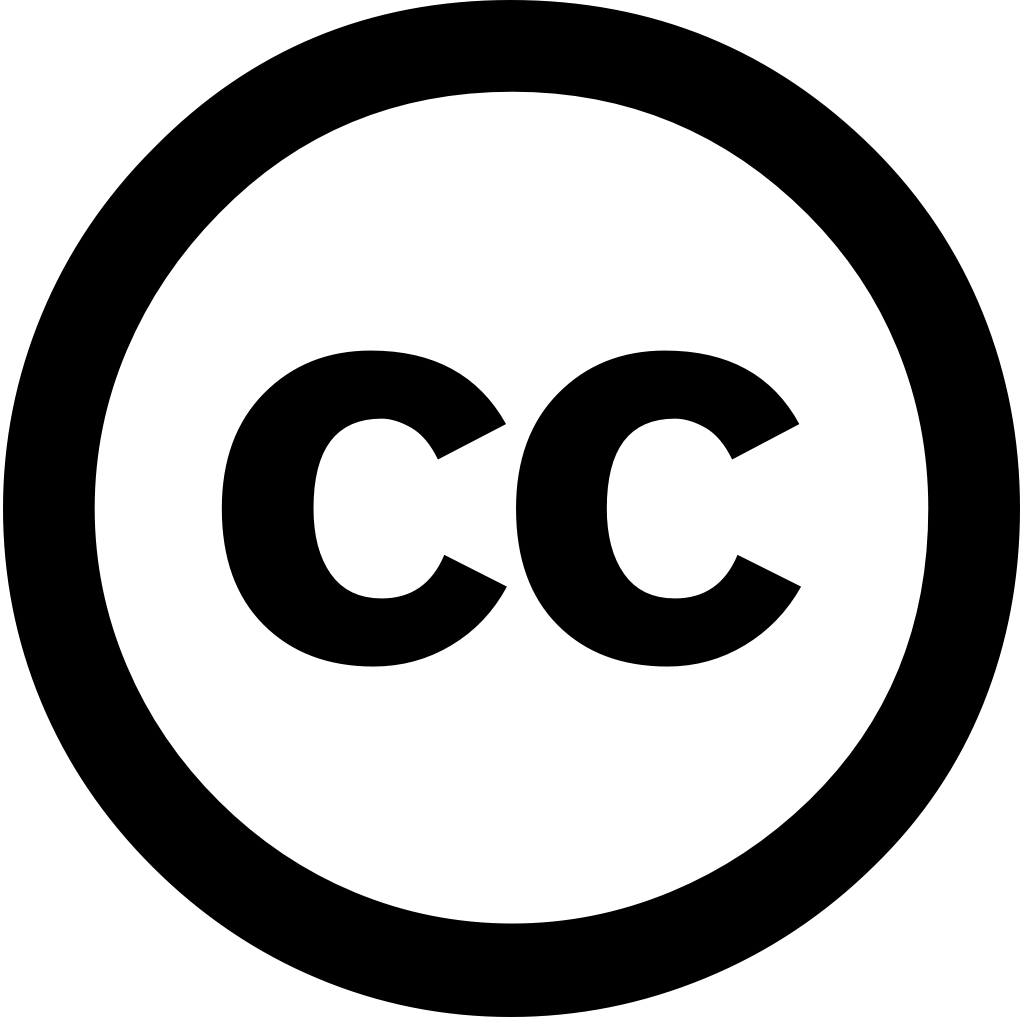
Iranian Journal of Medical Microbiology, Journal Year: 2024, Volume and Issue: 18(5), P. 287 - 300
Published: Nov. 30, 2024
Language: Английский
Iranian Journal of Medical Microbiology, Journal Year: 2024, Volume and Issue: 18(5), P. 287 - 300
Published: Nov. 30, 2024
Language: Английский
Diagnostics, Journal Year: 2024, Volume and Issue: 14(17), P. 1974 - 1974
Published: Sept. 6, 2024
(1) Background: Urinary tract infection (UTI) is a leading cause of emergency department visits and hospital admissions. Despite many studies identifying UTI-related risk factors for bacteremia or sepsis, significant gap remains in developing predictive models in-hospital mortality the necessity emergent intensive care unit admission department. This study aimed to construct interpretable machine learning capable patients at high critical outcomes. (2) Methods: was retrospective adult with urinary (UTI), extracted from Medical Information Mart Intensive Care IV Emergency Department (MIMIC-IV-ED) database. The outcome defined as either transfer an within 12 h. ED were randomly partitioned into 70%/30% split training validation. extreme gradient boosting (XGBoost), random forest (RF), support vector (SVM) algorithms constructed using variables selected stepwise logistic regression model. XGBoost model then compared traditional clinical decision rules (CDRs) on validation data area under curve (AUC). (3) Results: There 3622 among 3235 unique diagnosed UTI. Of 2535 group, 836 (33%) experienced outcomes, 1087 358 (32.9%) did. AUCs different follows: XGBoost, 0.833; RF, 0.814; SVM, 0.799. performed better than others. (4) Conclusions: Machine outperformed existing CDRs predicting outcomes Future research should prospectively evaluate effectiveness this approach integrate it practice.
Language: Английский
Citations
1BioMedInformatics, Journal Year: 2024, Volume and Issue: 4(1), P. 733 - 753
Published: March 2, 2024
Background: Antibiotics can play a pivotal role in the treatment of colorectal cancer (CRC) at various stages disease, both directly and indirectly. Identifying novel patterns antibiotic effects or responses CRC within extensive medical data poses significant challenge that be addressed through algorithmic approaches. Machine Learning (ML) emerges as promising solution for predicting clinical outcomes using heterogeneous data. In pursuit our objective, we employed ML techniques mortality influence. Methods: We utilized dataset to examine accuracy death prediction metastatic cancer. addition, analyzed association between exposure The comprised 147 patients, nineteen independent variables, one dependent variable. Our analysis involved testing different classification-supervised ML, including an oversampling pool classification models, Logistic Regression, Decision Trees, Naive Bayes, Support Vector Machine, Random Forest, XGBboost Classifier, consensus all top models (meta models). Results: models’ classifier exhibited highest among algorithms tested (93%). This model met standards good accuracy, surpassing 90% threshold considered useful applications. Consistent with results, other metrics are also good, precision (0.96), recall (0.93), F-Beta (0.94), AUC (0.93). Hazard ratio suggests there is no discernible difference patients who received antibiotics those did not. Conclusions: modelling approach provides alternative analyzing relationship treated bevacizumab, complementing classic statistical methods. methodology lays groundwork future use datasets research highlights advantages meta models.
Language: Английский
Citations
1Expert Review of Medical Devices, Journal Year: 2024, Volume and Issue: unknown
Published: Nov. 25, 2024
Diagnosing oral cancer is crucial in healthcare, with technological advancements enhancing early detection and outcomes. This review examines the impact of handheld AI-based tools, focusing on Convolutional Neural Networks (CNNs) their advanced architectures diagnosis.
Language: Английский
Citations
1Iranian Journal of Medical Microbiology, Journal Year: 2024, Volume and Issue: 18(2), P. 66 - 79
Published: May 25, 2024
Artificial intelligence (AI), described as computer algorithms that exhibit cognitive characteristics like learning abilities, now affecting our lives in many areas.In the medical field, AI-supported image analysis has already taken on a central role pathology, radiology and dermatology.The policy of this review consisted peer-reviewed literature annotated Web Science, Scopus, PubMed Google Scholar databases.Articles were reviewed describe use AI to analyze images diagnose infectious diseases.Digitization healthcare is having profound impact patients.It expected development started will continue gain momentum.Machine fundamentally changing way we interact with health-related data, including clinical microbiology disease data.We likely transition from Internet Things environment Bodies devices providing detailed health data even disease-free times.The focus study was current views attempts apply methods daily practice, well search for promising diseases most efficient way.
Language: Английский
Citations
0Computation, Journal Year: 2024, Volume and Issue: 12(8), P. 167 - 167
Published: Aug. 18, 2024
In the contemporary era, dizziness is a prevalent ailment among patients. It can be caused by either vestibular neuritis or stroke. Given lack of diagnostic utility instrumental methods in acute isolated vertigo, differentiation and stroke primarily clinical. As part initial differential diagnosis, physician focuses on characteristics nystagmus results video head impulse test (vHIT). Instruments for accurate vHIT are costly often utilized exclusively healthcare settings. The objective this paper to review methodologies accurately detecting position pupil centers both eyes patient precisely extracting their coordinates. Additionally, describes determining rotation angle under diverse imaging lighting conditions. Furthermore, suitability these being evaluated. We assume maximum allowable error 0.005 radians per frame detect pupils’ coordinates 0.3 degrees while position. found that such conditions, most suitable approaches posture detection deep learning (including LSTM networks), search template matching, linear regression EMG sensor data, optical fiber usage. relevant localization our medical tasks learning, geometric transformations, decision trees, RASNAC. This study might assist identification number employed future construct high-accuracy system based smartphone home computer, with subsequent signal processing diagnosis.
Language: Английский
Citations
0Technologies, Journal Year: 2024, Volume and Issue: 12(9), P. 142 - 142
Published: Aug. 27, 2024
In recent years, COVID-19 and skin cancer have become two prevalent illnesses with severe consequences if untreated. This research represents a significant step toward leveraging machine learning (ML) ensemble techniques to improve the accuracy efficiency of medical image diagnosis for critical diseases such as (grayscale images) (RGB images). this paper, stacked approach is proposed enhance precision effectiveness both cancer. The method combines pretrained models convolutional neural networks (CNNs) including ResNet101, DenseNet121, VGG16 feature extraction grayscale (COVID-19) RGB (skin cancer) images. performance model evaluated using individual CNNs combination vectors generated from architectures. obtained through transfer are then fed into base-learner consisting five different ML algorithms. final step, predictions models, validation dataset, extracted assembled applied input meta-learner obtain predictions. metrics show high intermediate
Language: Английский
Citations
0BMC Cancer, Journal Year: 2024, Volume and Issue: 24(1)
Published: Aug. 29, 2024
Disulfidptosis is an emerging form of cellular death resulting from the binding intracellular disulfide bonds to actin cytoskeleton proteins. This study aimed investigate expression and prognostic significance hub disulfidptosis-related lncRNAs (DRLRs) in R0 resected hepatocellular carcinoma (HCC) as well their impact on malignant behaviour HCC cells.
Language: Английский
Citations
0Health Informatics Journal, Journal Year: 2024, Volume and Issue: 30(4)
Published: Oct. 1, 2024
Objective: Integrating artificial intelligence (AI) solutions into clinical practice, particularly in the field of video capsule endoscopy (VCE), necessitates execution rigorous studies. Methods: This work introduces a novel software platform tailored to facilitate conduct multi–reader multi–case studies VCE. The platform, developed as web application, prioritizes remote accessibility accommodate multi–center Notably, considerable attention was devoted user interface and experience design elements ensure seamless engaging interface. To evaluate usability pilot study is conducted. Results: results indicate high level acceptance among users, providing valuable insights expectations preferences gastroenterologists navigating AI–driven VCE solutions. Conclusion: research lays foundation for future advancements AI integration within practice.
Language: Английский
Citations
0Health Systems, Journal Year: 2024, Volume and Issue: unknown, P. 1 - 15
Published: Dec. 9, 2024
Preeclampsia, a life-threatening condition in late pregnancy, has unclear causes and risk factors. Machine learning (ML) offers promising approach for early prediction. This systematic review analyzes state-of-the-art studies on preeclampsia prediction using ML approaches. We reviewed articles published between January 1 2013 December 31 2023, from Google Scholar PubMed. Of 183 identified studies, 35 were selected based inclusion exclusion criteria. Our findings reveal that key predictive features commonly used machine models include age, number of pregnancies, body mass index, diabetes, hypertension, blood pressure. In contrast, factors such as medications, genetic data, clinical imaging considered less frequently. Random Forest, Support Vector Machine, Logistic Regression, Decision Tree, Naïve Bayes the most algorithms. Most conducted China USA, indicating geographic concentration. The field seen notable rise research, especially past two years, though many rely small datasets single hospitals. highlights need more diverse comprehensive research to enhance detection management preeclampsia.
Language: Английский
Citations
0Applied Sciences, Journal Year: 2024, Volume and Issue: 14(2), P. 607 - 607
Published: Jan. 10, 2024
Protozoa detection and classification from freshwaters microscopic imaging are critical components in environmental monitoring, parasitology, science, biological processes, scientific research. Bacterial parasitic contamination of water plays an important role society health. Conventional methods often rely on manual identification, resulting time-consuming analyses limited scalability. In this study, we propose a real-time protozoa framework using the YOLOv4 algorithm, state-of-the-art deep learning model known for its exceptional speed accuracy. Our dataset consists objects species, such as Bdelloid Rotifera, Stylonychia Pustulata, Paramecium, Hypotrich Ciliate, Colpoda, Lepocinclis Acus, Clathrulina Elegans, which have different shapes, sizes, movements. One major properties our work is to create by forming cultures various sources like rainwater puddles. network architecture carefully tailored optimize protozoa, ensuring precise localization individual organisms. To validate approach, extensive experiments conducted real-world image datasets. The results demonstrate that YOLOv4-based achieves outstanding accuracy significantly outperforms traditional terms precision. capabilities enable rapid analysis large-scale datasets, making it highly suitable dynamic environments time-sensitive applications. Furthermore, introduce user-friendly interface allows researchers professionals effortlessly deploy tool. We f1-score 0.95, precision 0.92, sensitivity 0.98, mAP 0.9752 evaluating metrics. proposed achieved 97% After reaching high efficiency, desktop application was developed provide testing model. framework’s significant implications fields, ranging support tool paramesiology/parasitology studies quality assessments, offering powerful enhance understanding preservation ecosystems.
Language: Английский
Citations
0