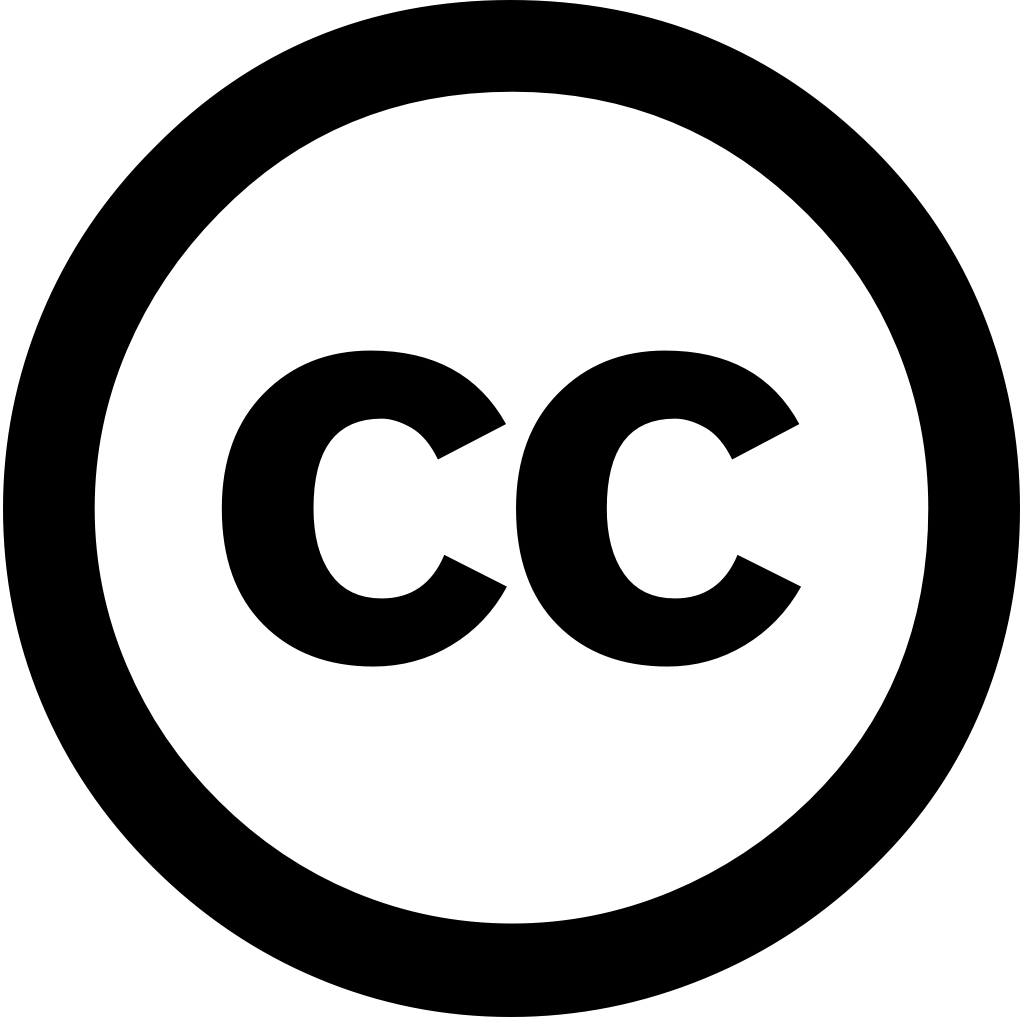
BMJ evidence-based medicine, Journal Year: 2024, Volume and Issue: unknown, P. bmjebm - 113199
Published: Dec. 20, 2024
Language: Английский
BMJ evidence-based medicine, Journal Year: 2024, Volume and Issue: unknown, P. bmjebm - 113199
Published: Dec. 20, 2024
Language: Английский
Journal of the Medical Library Association JMLA, Journal Year: 2025, Volume and Issue: 113(1), P. 31 - 38
Published: Jan. 14, 2025
Sexual and gender minority (SGM) populations experience health disparities compared to heterosexual cisgender populations. The development of accurate, comprehensive sexual orientation identity (SOGI) measures is fundamental quantify address SGM disparities, which first requires identifying SOGI-related research. As part a larger project reviewing synthesizing how SOGI has been assessed within the literature, we provide an example application automated tools for systematic reviews area measurement. In collaboration with research librarians, three-phase approach was used prioritize screening set 11,441 measurement studies published since 2012. Phase 1, search results were stratified into two groups (title vs. without measurement-related terms); titles terms manually screened. 2, supervised clustering using DoCTER software sort remaining based on relevance. 3, machine learning further identify deemed low relevance in 2 should be prioritized manual screening. 1,607 identified 1. Across Phases team excluded 5,056 9,834 DoCTER. review, percentage relevant screened low, ranging from 0.1 7.8 percent. Automated librarians have potential save hundreds hours human labor large-scale
Language: Английский
Citations
2Journal of Medical Internet Research, Journal Year: 2024, Volume and Issue: 26, P. e52758 - e52758
Published: Aug. 16, 2024
Background The screening process for systematic reviews is resource-intensive. Although previous machine learning solutions have reported reductions in workload, they risked excluding relevant papers. Objective We evaluated the performance of a 3-layer method using GPT-3.5 and GPT-4 to streamline title abstract-screening reviews. Our goal develop that maximizes sensitivity identifying records. Methods conducted screenings on 2 our related treatment bipolar disorder, with 1381 records from first review 3146 second. Screenings were (gpt-3.5-turbo-0125) (gpt-4-0125-preview) across three layers: (1) research design, (2) target patients, (3) interventions controls. was prompts tailored each study. During this process, information extraction according study’s inclusion criteria optimization carried out GPT-4–based flow without manual adjustments. Records at layer, those meeting all layers subsequently judged as included. Results On both able about 110 per minute, total time required second studies approximately 1 hour hours, respectively. In study, sensitivities/specificities 0.900/0.709 0.806/0.996, Both by 6 used meta-analysis 0.958/0.116 0.875/0.855, sensitivities align human evaluators: 0.867-1.000 study 0.776-0.979 9 After accounting justifiably excluded GPT-4, 0.962/0.996 0.943/0.855 Further investigation indicated cases incorrectly due lack domain knowledge, while misinterpretations criteria. Conclusions demonstrated acceptable level specificity supports its practical application screenings. Future should aim generalize approach explore effectiveness diverse settings, medical nonmedical, fully establish use operational feasibility.
Language: Английский
Citations
10PLoS ONE, Journal Year: 2025, Volume and Issue: 20(1), P. e0313401 - e0313401
Published: Jan. 7, 2025
Background Systematic reviews provide clarity of a bulk evidence and support the transfer knowledge from clinical trials to guidelines. Yet, they are time-consuming. Artificial intelligence (AI), like ChatGPT-4o, may streamline processes data extraction, but its efficacy requires validation. Objective This study aims (1) evaluate validity ChatGPT-4o for extraction compared human reviewers, (2) test reproducibility ChatGPT-4o’s extraction. Methods We conducted comparative using papers an ongoing systematic review on exercise reduce fall risk. Data extracted by were reference standard: two independent reviewers. The was assessed categorizing into five categories ranging completely correct false data. Reproducibility evaluated comparing in separate sessions different accounts. Results total 484 points across 11 papers. AI’s 92.4% accurate (95% CI: 89.5% 94.5%) produced 5.2% cases 3.4% 7.4%). between high, with overall agreement 94.1%. decreased when information not reported papers, 77.2%. Conclusion Validity high reviews. qualified as second reviewer showed potential future advancements summarizing
Language: Английский
Citations
1Annals of Internal Medicine, Journal Year: 2025, Volume and Issue: unknown
Published: Feb. 24, 2025
Systematic reviews (SRs) are hindered by the initial rigorous article screen, which delays access to reliable information synthesis. To develop generic prompt templates for large language model (LLM)-driven abstract and full-text screening that can be adapted different reviews. Diagnostic test accuracy. 48 425 citations were tested across 10 SRs. Full-text evaluated all 12 690 freely available articles from original search. Prompt development used GPT4-0125-preview (OpenAI). None. Large models prompted include or exclude based on SR eligibility criteria. Model outputs compared with author decisions after evaluate performance (accuracy, sensitivity, specificity). Optimized prompts using achieved a weighted sensitivity of 97.7% (range, 86.7% 100%) specificity 85.2% 68.3% 95.9%) in 96.5% 89.7% 100.0%) 91.2% 80.7% In contrast, zero-shot had poor (49.0% abstract, 49.1% full-text). Across LLMs, Claude-3.5 (Anthropic) GPT4 variants similar performance, whereas Gemini Pro (Google) GPT3.5 (OpenAI) underperformed. Direct costs 000 differed substantially: Where single human was estimated require more than 83 hours $1666.67 USD, our LLM-based approach completed under 1 day $157.02 USD. Further optimizations may exist. Retrospective study. Convenience sample evaluations limited free PubMed Central articles. A achieving high other SRs LLMs developed. Our prompting innovations have value investigators researchers conducting criteria-based tasks medical sciences.
Language: Английский
Citations
1BMJ evidence-based medicine, Journal Year: 2025, Volume and Issue: unknown, P. bmjebm - 113320
Published: Jan. 9, 2025
Language: Английский
Citations
0JMIR Medical Informatics, Journal Year: 2025, Volume and Issue: 13, P. e64682 - e64682
Published: March 12, 2025
Abstract This study demonstrated that while GPT-4 Turbo had superior specificity when compared to GPT-3.5 (0.98 vs 0.51), as well comparable sensitivity (0.85 0.83), processed 100 studies faster (0.9 min 1.6 min) in citation screening for systematic reviews, suggesting may be more suitable due its higher and highlighting the potential of large language models optimizing literature selection.
Language: Английский
Citations
0Research Synthesis Methods, Journal Year: 2025, Volume and Issue: unknown, P. 1 - 11
Published: March 24, 2025
Abstract With the increasing volume of scientific literature, there is a need to streamline screening process for titles and abstracts in systematic reviews, reduce workload reviewers, minimize errors. This study validated artificial intelligence (AI) tools, specifically Llama 3 70B via Groq’s application programming interface (API) ChatGPT-4o mini OpenAI’s API, automating this biomedical research. It compared these AI tools with human reviewers using 1,081 articles after duplicate removal. Each model was tested three configurations assess sensitivity, specificity, predictive values, likelihood ratios. The model’s LLA_2 configuration achieved 77.5% sensitivity 91.4% 90.2% accuracy, positive value (PPV) 44.3%, negative (NPV) 97.9%. CHAT_2 showed 56.2% 95.1% 92.0% PPV 50.6%, an NPV 96.1%. Both models demonstrated strong having higher overall accuracy. Despite promising results, manual validation remains necessary address false positives negatives, ensuring that no important studies are overlooked. suggests can significantly enhance efficiency accuracy potentially revolutionizing not only research but also other fields requiring extensive literature reviews.
Language: Английский
Citations
0Deleted Journal, Journal Year: 2025, Volume and Issue: 73(2), P. 202990 - 202990
Published: April 1, 2025
Mental health disorders have a high disability-adjusted life years in the Middle East and North Africa. This rise has led to surge related publications, prompting researchers use AI tools like ChatGPT reduce time spent on routine tasks. Our study aimed validate an AI-assisted critical appraisal (CA) tool by comparing it with human raters. We developed customized GPT models using ChatGPT-4. These were tailored evaluate studies Newcastle-Ottawa Scale (NOS) or Jadad one model, while another model evaluated STROBE CONSORT guidelines. results showed moderate good agreement between CA our GPTs for NOS cohort, case control cross-sectional scale, ICC of 0.68 [95 %CI: 0.24-0.82], 0.69 0.31-0.88], 0.76 0.47-0.90] 0.84 0.57-0.94] respectively. There was also substantial two methods cross sectional, studies, trial design, K 0.63 0.56-0.70], 0.57 0.47-0.66], 0.48 0.38-0.50] 0.70 0.63-0.77] custom produced hallucinations 6.5 % 4.9 cases, Human raters took average 19.6 ± 4.3 min per article, whereas only 1.4. could be useful handling repetitive tasks yet its effective application relies expertise researchers.
Language: Английский
Citations
0Research Synthesis Methods, Journal Year: 2025, Volume and Issue: unknown, P. 1 - 19
Published: April 24, 2025
Abstract Introduction With the increasing accessibility of tools such as ChatGPT, Copilot, DeepSeek, Dall-E, and Gemini, generative artificial intelligence (GenAI) has been poised a potential, research timesaving tool, especially for synthesising evidence. Our objective was to determine whether GenAI can assist with evidence synthesis by assessing its performance using accuracy, error rates, time savings compared traditional expert-driven approach. Methods To systematically review evidence, we searched five databases on 17 January 2025, synthesised outcomes reporting or taken, appraised risk-of-bias modified version QUADAS-2. Results We identified 3,071 unique records, 19 which were included in our review. Most studies had high unclear Domain 1A: selection, 2A: conduct, 1B: applicability results. When used (1) searching missed 68% 96% (median = 91%) studies, (2) screening made incorrect inclusion decisions ranging from 0% 29% 10%); exclusion 1% 83% 28%), (3) data extractions 4% 31% 14%), (4) assessments 10% 56% 27%). Conclusion shows that current does not support use without human involvement oversight. However, most tasks other than searching, may have role assisting humans synthesis.
Language: Английский
Citations
0PeerJ Computer Science, Journal Year: 2025, Volume and Issue: 11, P. e2822 - e2822
Published: April 30, 2025
Background Large language models (LLMs) offer a potential solution to the labor-intensive nature of systematic reviews. This study evaluated ability GPT model identify articles that discuss perioperative risk factors for esophagectomy complications. To test performance model, we tested GPT-4 on narrower inclusion criterion and by assessing its discriminate relevant solely identified preoperative esophagectomy. Methods A literature search was run trained librarian studies ( n = 1,967) discussing The underwent title abstract screening three independent human reviewers GPT-4. Python script used analysis made Application Programming Interface (API) calls with criteria in natural language. GPT-4’s exclusion decision were compared those decided reviewers. Results agreement between 85.58% 78.75% factors. AUC value 0.87 0.75 query, respectively. In evaluation factors, demonstrated high recall included at 89%, positive predictive 74%, negative 84%, low false rate 6% macro-F1 score 0.81. For showed 67% studies, 65%, 85%, 15% 0.66. interobserver reliability substantial, kappa 0.69 0.61 Despite lower accuracy under more stringent criteria, proved valuable streamlining review workflow. Preliminary justification provided reported have been useful screeners, especially resolving discrepancies during screening. Conclusion demonstrates promising use LLMs streamline workflow integration reviews could lead significant time cost savings, however caution must be taken involving criterion. Future research is needed should explore integrating other steps review, such as full text or data extraction, compare different their effectiveness various types
Language: Английский
Citations
0