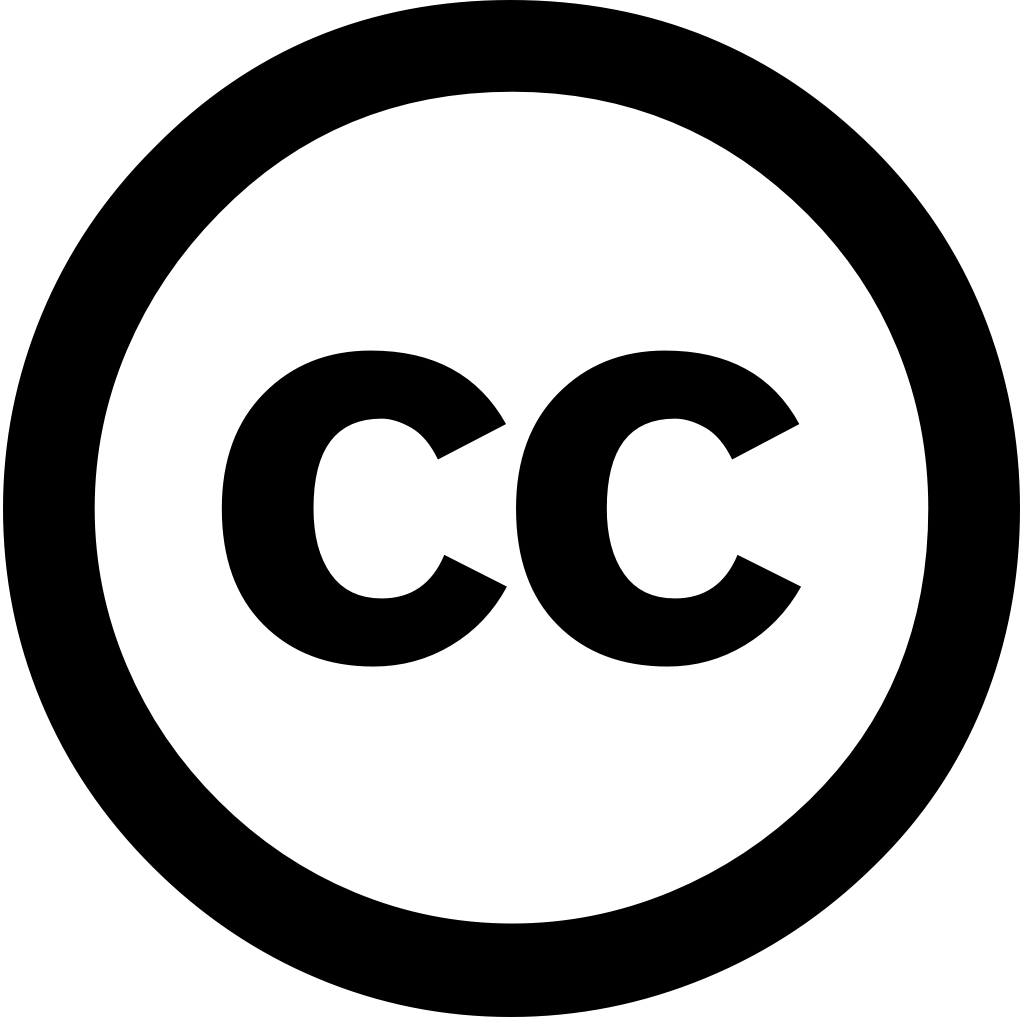
PeerJ Computer Science, Journal Year: 2025, Volume and Issue: 11, P. e2800 - e2800
Published: April 8, 2025
Kidney diseases are becoming an alarming concern around the globe. Premature diagnosis of kidney disease can save precious human lives by taking preventive measures. Deep learning demonstrates a substantial performance in various medical disciplines. Numerous deep approaches suggested literature for accurate chronic classification compromising on architectural complexity, speed, and resource constraints. In this study, transfer is exploited incorporating unexplored yet effective variants ConvNeXt EfficientNetV2 efficient diseases. The benchmark computed tomography (CT)-based database containing 12,446 CT scans tumor, stone cysts, normal patients utilized to train designed fine-tuned networks. However, due highly imbalanced distribution images among classes, operation data trimming balancing number each class, which essential designing unbiased predictive network. By utilizing pre-trained models our specific task, training time reduced leading computationally inexpensive solution. After comprehensive hyperparameters tuning with respect changes rates, batch sizes, optimizers, it depicted that EfficientNetV2B0 network 23.8 MB size only 6.2 million parameters shows diagnostic achieving generalized test accuracy 99.75% balanced database. Furthermore, attains high precision, recall, F1-score 99.75%, 99.63%, respectively. Moreover, final ensures its scalability impressive 99.73% set original dataset as well. Through extensive evaluation proposed strategy, concluded design outperforms counterparts terms computational efficiency tasks. serves accurate, efficient, solution tailored real-time deployment or mobile edge devices.
Language: Английский