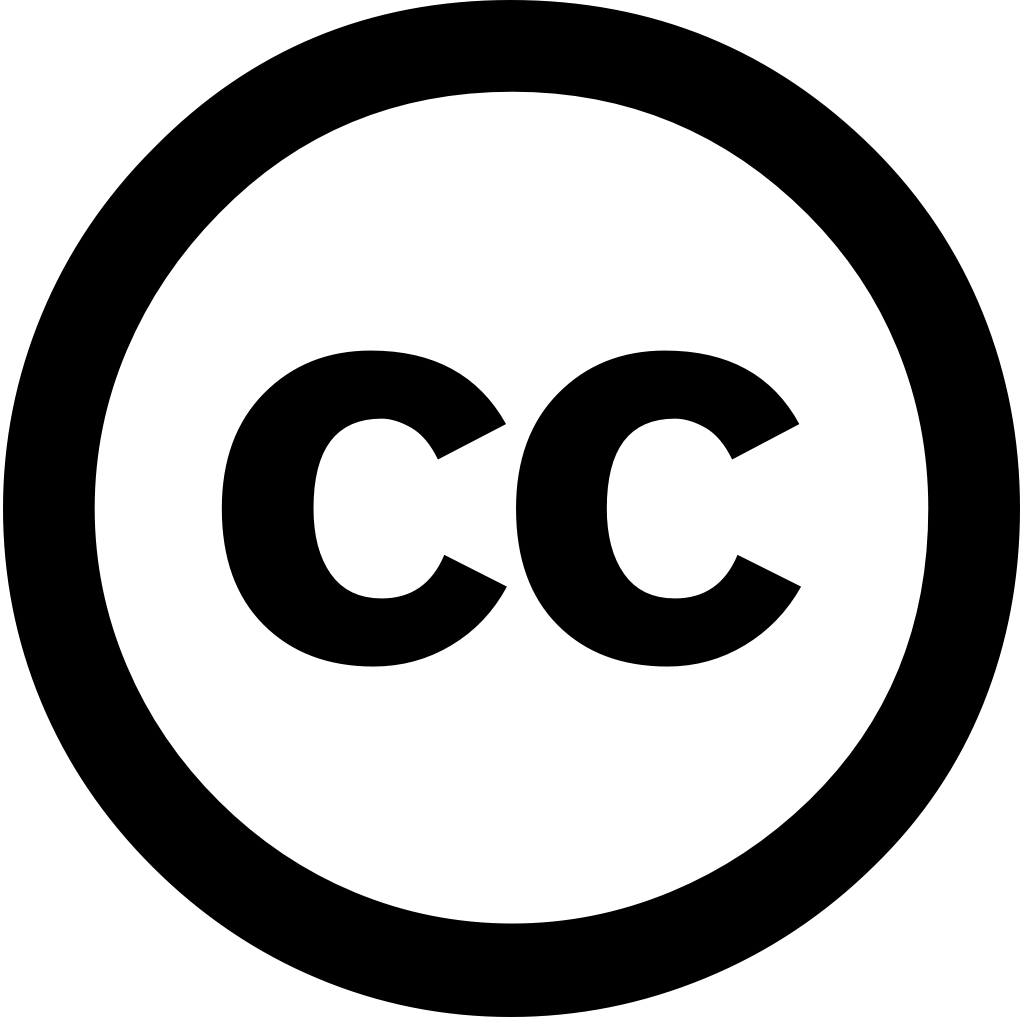
Mathematics, Journal Year: 2025, Volume and Issue: 13(9), P. 1473 - 1473
Published: April 30, 2025
Under the rapid evolution of financial technology, traditional credit risk management paradigms relying on expert experience and singular algorithmic architectures have proven inadequate in addressing complex decision-making demands arising from dynamically correlated multidimensional factors heterogeneous data fusion. This manuscript proposes an enhanced rating model based improved TabNet framework. First, Kaggle “Give Me Some Credit” dataset undergoes preprocessing, including balancing partitioning into training, testing, validation sets. Subsequently, architecture is refined through integration a multi-head attention mechanism to extract both global local feature representations. Bayesian optimization then employed accelerate hyperparameter selection automate parameter search for TabNet. To further enhance classification predictive performance, stacked ensemble learning approach implemented: serves as extractor, while XGBoost (Extreme Gradient Boosting), LightGBM (Light Boosting Machine), CatBoost (Categorical KNN (K-Nearest Neighbors), SVM (Support Vector Machine) are selected base learners first layer, with acting meta-learner second layer. The experimental results demonstrate that proposed TabNet-based outperforms benchmark models across multiple metrics, accuracy, precision, recall, F1-score, AUC (Area Curve), KS (Kolmogorov–Smirnov statistic).
Language: Английский