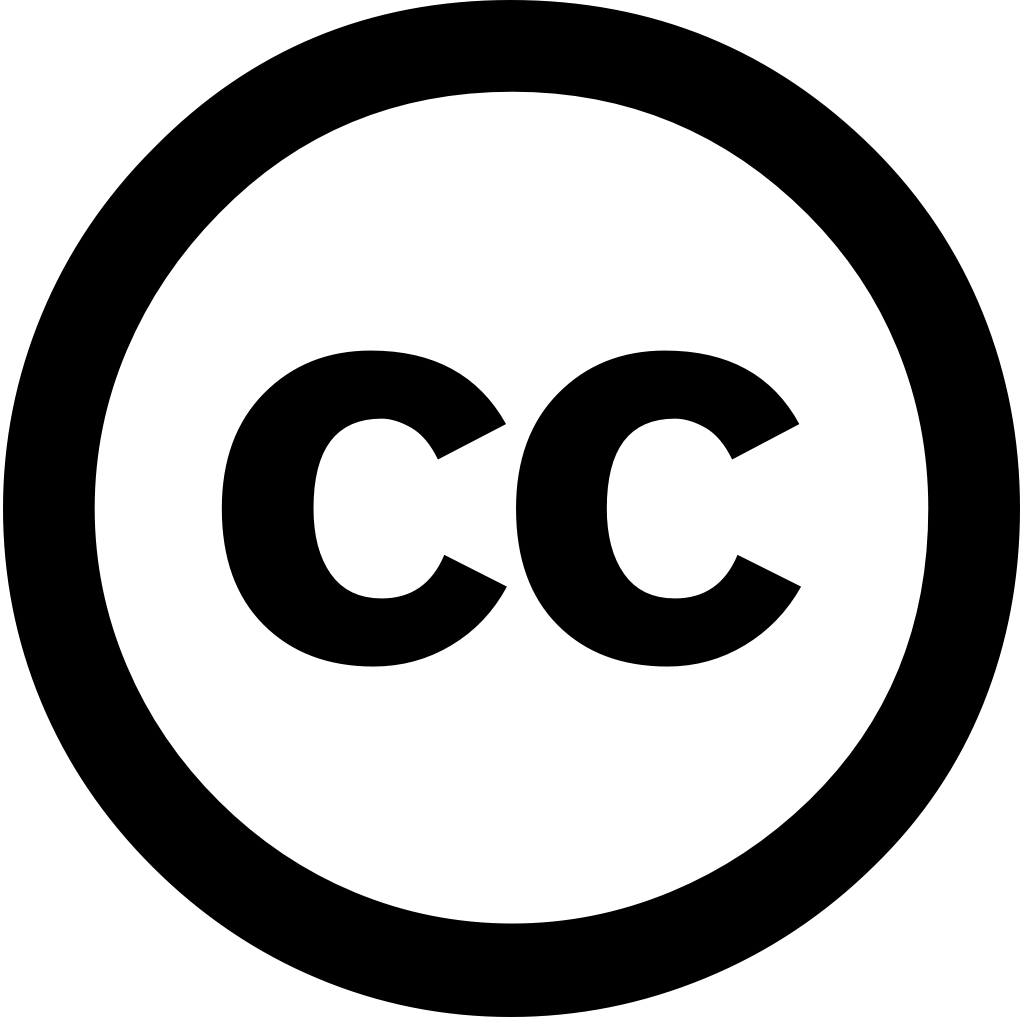
Matter, Journal Year: 2023, Volume and Issue: 6(6), P. 1831 - 1859
Published: June 1, 2023
Language: Английский
Matter, Journal Year: 2023, Volume and Issue: 6(6), P. 1831 - 1859
Published: June 1, 2023
Language: Английский
The Innovation, Journal Year: 2021, Volume and Issue: 2(4), P. 100179 - 100179
Published: Oct. 29, 2021
•"Can machines think?" The goal of artificial intelligence (AI) is to enable mimic human thoughts and behaviors, including learning, reasoning, predicting, so on.•"Can AI do fundamental research?" coupled with machine learning techniques impacting a wide range sciences, mathematics, medical science, physics, etc.•"How does accelerate New research applications are emerging rapidly the support by infrastructure, data storage, computing power, algorithms, frameworks. Artificial promising (ML) well known from computer science broadly affecting many aspects various fields technology, industry, even our day-to-day life. ML have been developed analyze high-throughput view obtaining useful insights, categorizing, making evidence-based decisions in novel ways, which will promote growth fuel sustainable booming AI. This paper undertakes comprehensive survey on development application different information materials geoscience, life chemistry. challenges that each discipline meets, potentials handle these challenges, discussed detail. Moreover, we shed light new trends entailing integration into scientific discipline. aim this provide broad guideline sciences potential infusion AI, help motivate researchers deeply understand state-of-the-art AI-based thereby continuous sciences.
Language: Английский
Citations
943npj Computational Materials, Journal Year: 2022, Volume and Issue: 8(1)
Published: April 5, 2022
Deep learning (DL) is one of the fastest growing topics in materials data science, with rapidly emerging applications spanning atomistic, image-based, spectral, and textual modalities. DL allows analysis unstructured automated identification features. Recent development large databases has fueled application methods atomistic prediction particular. In contrast, advances image spectral have largely leveraged synthetic enabled by high quality forward models as well generative unsupervised methods. this article, we present a high-level overview deep-learning followed detailed discussion recent developments deep simulation, imaging, analysis, natural language processing. For each modality discuss involving both theoretical experimental data, typical modeling approaches their strengths limitations, relevant publicly available software datasets. We conclude review cross-cutting work related to uncertainty quantification field brief perspective on challenges, potential growth areas for science. The science presents an exciting avenue future discovery design.
Language: Английский
Citations
608Advanced Materials, Journal Year: 2021, Volume and Issue: 34(25)
Published: Sept. 7, 2021
Abstract Lithium‐ion batteries (LIBs) are vital energy‐storage devices in modern society. However, the performance and cost still not satisfactory terms of energy density, power cycle life, safety, etc. To further improve batteries, traditional “trial‐and‐error” processes require a vast number tedious experiments. Computational chemistry artificial intelligence (AI) can significantly accelerate research development novel battery systems. Herein, heterogeneous category AI technology for predicting discovering materials estimating state system is reviewed. Successful examples, challenges deploying real‐world scenarios, an integrated framework analyzed outlined. The state‐of‐the‐art about applications ML property prediction discovery, including electrolyte electrode materials, summarized. Meanwhile, states also provided. Finally, various existing to tackle on machine learning rechargeable LIBs proposed.
Language: Английский
Citations
265InfoMat, Journal Year: 2020, Volume and Issue: 2(4), P. 656 - 697
Published: March 2, 2020
Abstract The pioneering exfoliation of monolayer tungsten diselenide has greatly inspired researchers toward semiconducting applications. WSe 2 belongs to a family transition‐metal dichalcogenides. Similar graphene, and analogous dichalcogenides have layered structures with weak van der Waals interactions between two adjacent layers. First, the readers are presented fundamentals 2, such as types, morphologies, properties. Here, we report characterization principles practices microscopy, spectroscopy, diffraction. Second, methods for obtaining high‐quality , exfoliation, hydrothermal chemical vapor deposition, briefly listed. With advantages light weight, flexibility, high quantum efficiency, 2D materials may niche in optoelectronics building blocks p‐n junctions. Therefore, introduce state‐of‐the‐art demonstration heterostructure devices employing p‐type semiconductor. device architectures include field‐effect transistors, photodetectors, gas sensors, photovoltaic solar cells. Due its unique electronic, optical, energy band properties, been increasingly investigated due conductivity charge carrier upon palladium contact. Eventually, dynamic research on heterostructures is summarized arouse passion community. image
Language: Английский
Citations
174Journal of the American Chemical Society, Journal Year: 2023, Volume and Issue: 145(16), P. 8736 - 8750
Published: April 13, 2023
Traditional computational approaches to design chemical species are limited by the need compute properties for a vast number of candidates, e.g., discriminative modeling. Therefore, inverse methods aim start from desired property and optimize corresponding structure. From machine learning viewpoint, problem can be addressed through so-called generative Mathematically, models defined probability distribution function given molecular or material In contrast, model seeks exploit joint with target characteristics. The overarching idea modeling is implement system that produces novel compounds expected have set features, effectively sidestepping issues found in forward process. this contribution, we overview critically analyze popular algorithms like adversarial networks, variational autoencoders, flow, diffusion models. We highlight key differences between each models, provide insights into recent success stories, discuss outstanding challenges realizing discovered solutions applications.
Language: Английский
Citations
169Archives of Computational Methods in Engineering, Journal Year: 2022, Volume and Issue: 30(1), P. 115 - 159
Published: July 24, 2022
Language: Английский
Citations
164Chemical Engineering Journal, Journal Year: 2020, Volume and Issue: 405, P. 126622 - 126622
Published: Aug. 11, 2020
Language: Английский
Citations
158Materials Today Nano, Journal Year: 2020, Volume and Issue: 11, P. 100087 - 100087
Published: June 4, 2020
Language: Английский
Citations
153Energy and AI, Journal Year: 2021, Volume and Issue: 3, P. 100049 - 100049
Published: Jan. 24, 2021
The screening of advanced materials coupled with the modeling their quantitative structural-activity relationships has recently become one hot and trending topics in energy due to diverse challenges, including low success probabilities, high time consumption, computational cost associated traditional methods developing materials. Following this, new research concepts technologies promote development necessary. latest advancements artificial intelligence machine learning have therefore increased expectation that data-driven science would revolutionize scientific discoveries towards providing paradigms for Furthermore, current advances engineering also demonstrate application technology not only significantly facilitate design but enhance discovery deployment. In this article, importance necessity contributing global carbon neutrality are presented. A comprehensive introduction fundamentals is provided, open-source databases, feature engineering, algorithms, analysis model. Afterwards, progress alkaline ion battery materials, photovoltaic catalytic dioxide capture discussed. Finally, relevant clues successful applications remaining challenges highlighted.
Language: Английский
Citations
152Advanced Materials, Journal Year: 2023, Volume and Issue: 36(4)
Published: Oct. 23, 2023
Abstract Microwave absorption materials (MAMs) are originally developed for military purposes, but have since evolved into versatile with promising applications in modern technologies, including household use. Despite significant progress bench‐side research over the past decade, MAMs remain limited their scope and yet to be widely adopted. This review explores history of from first‐generation coatings second‐generation functional absorbers, identifies bottlenecks hindering maturation. It also presents potential solutions such as exploring broader spatial scales, advanced characterization, introducing liquid media, utilizing novel toolbox (machine learning, ML), proximity lab end‐user. Additionally, it meticulously compelling medicine, mechanics, energy, optics, sensing, which go beyond efficiency, along current development status prospects. interdisciplinary direction differs previous primarily focused on meeting traditional requirements (i.e., thin, lightweight, wide, strong), can defined next generation smart absorbers. Ultimately, effective utilization ubiquitous electromagnetic (EM) waves, aided by third‐generation MAMs, should better aligned future expectations.
Language: Английский
Citations
136