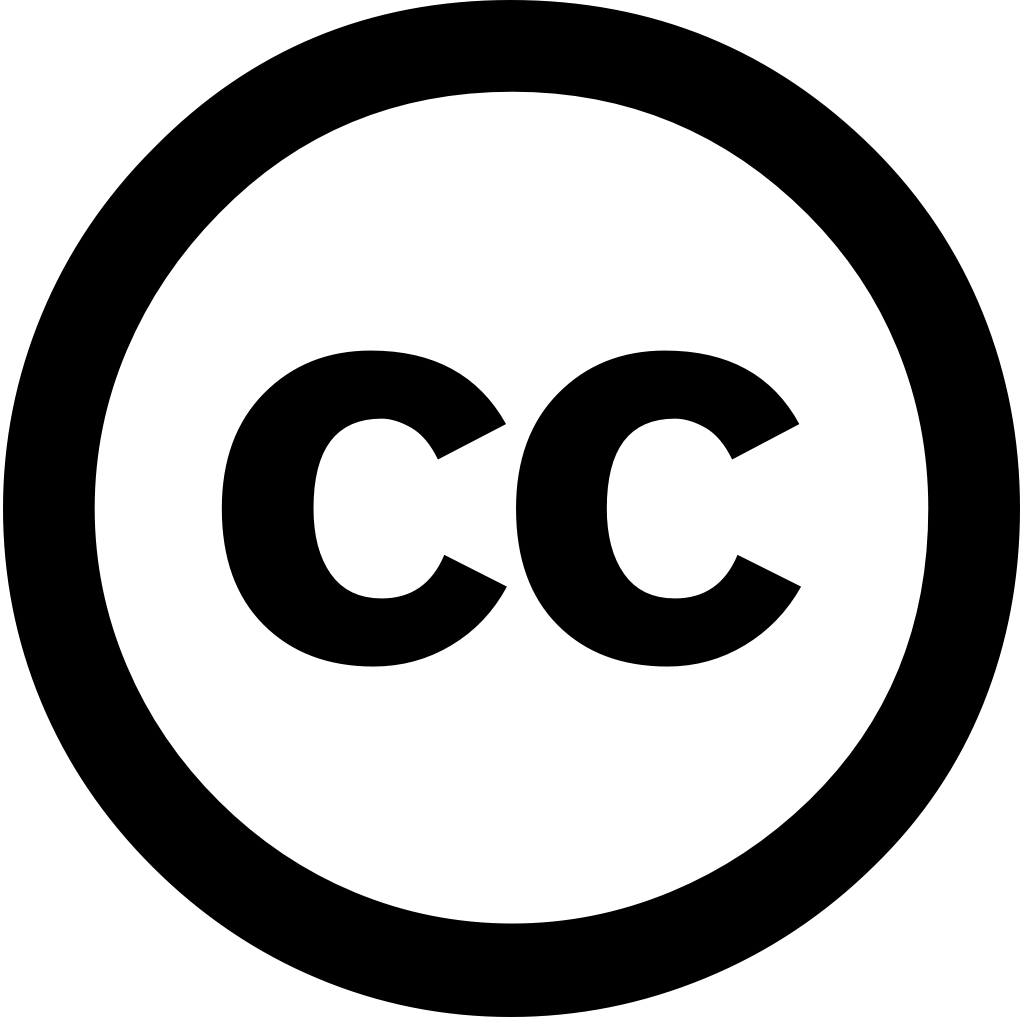
International Journal of Mechanical Sciences, Journal Year: 2024, Volume and Issue: 286, P. 109824 - 109824
Published: Nov. 12, 2024
Language: Английский
International Journal of Mechanical Sciences, Journal Year: 2024, Volume and Issue: 286, P. 109824 - 109824
Published: Nov. 12, 2024
Language: Английский
Thin-Walled Structures, Journal Year: 2024, Volume and Issue: 205, P. 112495 - 112495
Published: Sept. 24, 2024
Language: Английский
Citations
35International Journal for Numerical Methods in Engineering, Journal Year: 2023, Volume and Issue: 125(4)
Published: Nov. 8, 2023
Abstract Physics‐informed neural networks (PINNs) are a new tool for solving boundary value problems by defining loss functions of based on governing equations, conditions, and initial conditions. Recent investigations have shown that when designing many engineering problems, using first‐order derivatives combining equations from both strong weak forms can lead to much better accuracy, especially there heterogeneity variable jumps in the domain. This approach is called mixed formulation PINNs, which takes ideas finite element method. In this method, PDE reformulated as system where primary unknowns fluxes or gradients solution, secondary solution itself. work, we propose applying solve multi‐physical specifically stationary thermo‐mechanically coupled equations. Additionally, discuss sequential fully unsupervised training compare their accuracy computational cost. To improve network, incorporate hard constraints ensure valid predictions. We then investigate how different optimizers architectures affect efficiency. Finally, introduce simple parametric learning similar transfer learning. combines data physics address limitations PINNs regarding cost improves network's ability predict response unseen cases. The outcomes work will be useful other applications deep employed multiple systems fast reliable computations.
Language: Английский
Citations
37Computational Mechanics, Journal Year: 2024, Volume and Issue: 74(6), P. 1279 - 1301
Published: May 6, 2024
Abstract We present an approach for the data-driven modeling of nonlinear viscoelastic materials at small strains which is based on physics-augmented neural networks (NNs) and requires only stress strain paths training. The model built concept generalized standard therefore thermodynamically consistent by construction. It consists a free energy dissipation potential, can be either expressed components their tensor arguments or suitable set invariants. two potentials are described fully/partially input convex networks. For training NN strain, efficient flexible method long short-term memory cell developed to automatically generate internal variable(s) during process. proposed benchmarked thoroughly compared with existing approaches. Different databases ideal noisy data generated using conventional reference model. coordinate-based invariant-based formulation advantages latter demonstrated. Afterwards, calibrated applying three methods data. All yield good results, but differ in computation time usability large sets. presented recurrent turns out particularly robust widely applicable. show that together complete accurate 3D constitutive models even sparse bi- uniaxial
Language: Английский
Citations
14International Journal of Mechanical Sciences, Journal Year: 2024, Volume and Issue: 280, P. 109525 - 109525
Published: July 6, 2024
In this paper, we make the first attempt to adopt boundary integrated neural networks (BINNs) for numerical solution of two-dimensional (2D) elastostatic and piezoelectric problems. The proposed BINNs combine artificial with exact integral equations (BIEs) effectively solve value problems based on corresponding partial differential (PDEs). BIEs are utilized localize all unknown physical quantities boundary, which approximated by using resolved via a training process. contrast many traditional network methods domain discretization, present offer several distinct advantages. Firstly, embedding analytical into learning procedure, only need discretize problem domain, reduces number unknowns can lead faster more stable Secondly, operators in original PDEs substituted operators, eliminate additional differentiations (high-order derivatives may instabilities process). Thirdly, loss function contains residuals BIEs, as conditions have been inherently incorporated within formulation. Therefore, there is no necessity employing any weighting functions, commonly used most balance gradients among different objective functions. Extensive experiments show that much easier train usually provide accurate solutions compared methods.
Language: Английский
Citations
12Results in Engineering, Journal Year: 2025, Volume and Issue: unknown, P. 103976 - 103976
Published: Jan. 1, 2025
Language: Английский
Citations
1Computers and Geotechnics, Journal Year: 2025, Volume and Issue: 180, P. 107091 - 107091
Published: Jan. 27, 2025
Language: Английский
Citations
1Mechanics of Advanced Materials and Structures, Journal Year: 2024, Volume and Issue: unknown, P. 1 - 19
Published: Dec. 17, 2024
Machine learning (ML) models are widely used across numerous scientific and engineering disciplines due to their exceptional performance, flexibility, prediction quality, ability handle highly complex problems if appropriate data available. One example of such areas which has attracted a lot attentions in the last couple years is integration data-driven approaches material modeling. There been several successful researches implementing ML-based constitutive instead classical phenomenological for various materials, particularly those with non-linear mechanical behaviors. This review paper aims systematically investigate literature on materials classify these based suitability non-linearity including Non-linear elasticity (hyperelasticity), plasticity, visco-elasticity, visco-plasticity. Furthermore, we also reviewed compared that have applied architectured as groups designed represent specific behaviors might not exist conventional categories. The other goal this provide initial steps understanding modeling, artificial neural networks (ANN), Gaussian processes, random forests (RF), generated adversarial (GANs), support vector machines (SVM), different regression physics-informed (PINN). outlines collection methods, types data, processing approaches, theoretical background ML models, advantage limitations potential future research directions. comprehensive will researchers knowledge necessary develop high-fidelity, robust, adaptable, flexible, accurate advanced materials.
Language: Английский
Citations
5Journal of the Mechanics and Physics of Solids, Journal Year: 2025, Volume and Issue: unknown, P. 106049 - 106049
Published: Jan. 1, 2025
Language: Английский
Citations
0Mechanical Systems and Signal Processing, Journal Year: 2025, Volume and Issue: 229, P. 112561 - 112561
Published: March 11, 2025
Language: Английский
Citations
0Computers and Geotechnics, Journal Year: 2025, Volume and Issue: 181, P. 107110 - 107110
Published: Feb. 5, 2025
Language: Английский
Citations
0