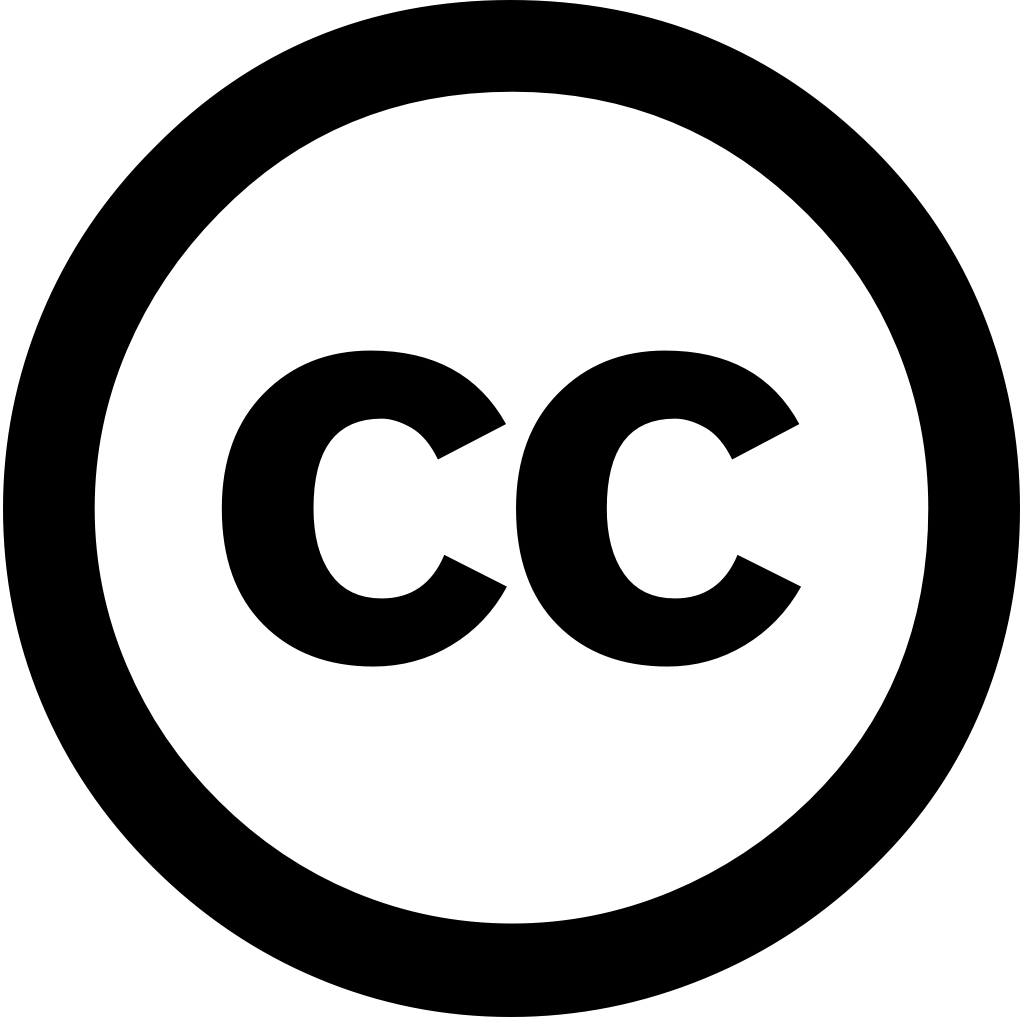
Neural Computing and Applications, Journal Year: 2025, Volume and Issue: unknown
Published: April 10, 2025
Language: Английский
Neural Computing and Applications, Journal Year: 2025, Volume and Issue: unknown
Published: April 10, 2025
Language: Английский
Artificial Intelligence Review, Journal Year: 2025, Volume and Issue: 58(3)
Published: Jan. 17, 2025
Edge deep learning, a paradigm change reconciling edge computing and facilitates real-time decision making attuned to environmental factors through the close integration of computational resources data sources. Here we provide comprehensive review current state art in focusing on computer vision applications, particular medical diagnostics. An overview foundational principles technical advantages learning is presented, emphasising capacity this technology revolutionise wide range domains. Furthermore, present novel categorisation hardware platforms based performance usage scenarios, facilitating platform selection operational effectiveness. Following this, dive into approaches effectively implement neural networks devices, encompassing methods such as lightweight design model compression. Reviewing practical applications fields general diagnostics particular, demonstrate profound impact edge-deployed models can have real-life situations. Finally, an analysis potential future directions obstacles adoption with intention stimulate further investigations advancements intelligent solutions. This survey provides researchers practitioners reference shedding light critical role plays advancement applications.
Language: Английский
Citations
1Scientific Reports, Journal Year: 2025, Volume and Issue: 15(1)
Published: Feb. 11, 2025
Skin cancer can be prevalent in people of any age group who are exposed to ultraviolet (UV) radiation. Among all other types, melanoma is a notable severe kind skin cancer, which fatal. Melanoma malignant arising from melanocytes, requiring early detection. Typically, lesions classified either as benign or malignant. However, some do exist that don't show clear signs, making them suspicious. If unnoticed, these suspicious develop into melanoma, invasive treatments later on. These intermediate completely curable if it diagnosed at their stages. To tackle this, few researchers intended improve the image quality infected obtained dermoscopy through reconstruction techniques. Analyzing reconstructed super-resolution (SR) images allows detection, fine feature extraction, and treatment plans. Despite advancements machine learning, deep complex neural networks enhancing lesion quality, key challenge remains unresolved: how intricate textures while performing significant up scaling medical reconstruction? Thus, an artificial intelligence (AI) based algorithm proposed obtain features dermoscopic for diagnosis. This serves non-invasive approach. In this research, novel information improvised generative adversarial network (MELIIGAN) framework expedited diagnosis lesions. Also, designed stacked residual block handles larger factors fine-grained details. Finally, hybrid loss function with total variation (TV) regularization term switches Charbonnier function, robust substitute mean square error function. The benchmark dataset results structural index similarity (SSIM) 0.946 peak signal-to-noise ratio (PSNR) 40.12 dB highest texture information, evidently compared state-of-the-art methods.
Language: Английский
Citations
1Scientific Reports, Journal Year: 2024, Volume and Issue: 14(1)
Published: July 3, 2024
Abstract Early detection of the adenocarcinoma cancer in colon tissue by means explainable deep learning, classifying histological images and providing visual explainability on model prediction. Considering that recent years, learning techniques have emerged as powerful medical image analysis, offering unprecedented accuracy efficiency, this paper we propose a method to automatically detect presence cancerous cells images. Various architectures are considered, with aim considering best one terms quantitative qualitative results. As matter fact, consider results taking into account so-called prediction explainability, way highlight areas from point view related cancer. The experimental performed 10,000 issue images, showed effectiveness proposed obtaining an equal 0.99. analysis shows can be successfully exploited for localisation
Language: Английский
Citations
8Image and Vision Computing, Journal Year: 2024, Volume and Issue: 147, P. 105064 - 105064
Published: May 3, 2024
Language: Английский
Citations
6IEEE Access, Journal Year: 2024, Volume and Issue: 12, P. 53396 - 53407
Published: Jan. 1, 2024
Accurate classification of moss species is essential for progress in ecology and biology. However, traditional methods classifying require significant expertise, current deep learning techniques struggle due to limited dataset diversity poor performance multi-class tasks. To overcome these challenges, we proposed the Swin Routiformer, a new algorithm image that enhances Transformer with bi-level routing attention. Addressing issue data, constructed images 110 different types. Additionally, propose Crop-Similar data augmentation algorithm, specifically designed images, reduce background noise interference prevent information loss feature scaling. Adopting model its multi-level hierarchical architecture visual extraction, introduce Routiformer Block, which network's interaction capabilities, reduces computational complexity, improves accuracy processing speed species. Our experimental results show achieves top-1 82.19% an f1-score 82.79% on test set, outperforming most mainstream models by 4.53% 1.81% respectively compared baseline model. These findings establish as valuable tool precise identification species, offering contributions related fields.
Language: Английский
Citations
4Image and Vision Computing, Journal Year: 2024, Volume and Issue: 147, P. 105061 - 105061
Published: May 8, 2024
Language: Английский
Citations
4Deleted Journal, Journal Year: 2025, Volume and Issue: unknown
Published: Jan. 17, 2025
Gastrointestinal (GI) disease examination presents significant challenges to doctors due the intricate structure of human digestive system. Colonoscopy and wireless capsule endoscopy are most commonly used tools for GI examination. However, large amount data generated by these technologies requires expertise intervention identification, making manual analysis a very time-consuming task. Thus, development computer-assisted system is highly desirable assist clinical professionals in decisions low-cost effective way. In this paper, we introduce novel framework called InCoLoTransNet, designed polyp segmentation. The study based on transformer convolution-involution neural network, following encoder-decoder architecture. We employed vision encoder section focus global context, while decoder involves collaboration resampling features. Involution enhances model's ability adaptively capture spatial contextual information, convolution focuses local leading more accurate feature extraction. essential features captured passed through two skip connection pathways. CBAM module refines passes them block, leveraging attention mechanisms emphasize relevant information. Meanwhile, locality self-attention pass involution reinforcing regions. Experiments were conducted five public datasets: CVC-ClinicDB, CVC-ColonDB, Kvasir-SEG, Etis-LaribPolypDB, CVC-300. results obtained InCoLoTransNet optimal when compared with 15 state-of-the-art methods segmentation, achieving highest mean dice score 93% CVC-ColonDB 90% intersection over union, outperforming methods. Additionally, distinguishes itself terms segmentation generalization performance. It achieved high scores coefficient union unseen datasets as follows: 85% 79% 91% 87% CVC-300, 70% respectively.
Language: Английский
Citations
0Neurocomputing, Journal Year: 2025, Volume and Issue: unknown, P. 129450 - 129450
Published: Jan. 1, 2025
Language: Английский
Citations
0Deleted Journal, Journal Year: 2025, Volume and Issue: unknown
Published: Jan. 27, 2025
This study explores a transfer learning approach with vision transformers (ViTs) and convolutional neural networks (CNNs) for classifying retinal diseases, specifically diabetic retinopathy, glaucoma, cataracts, from ophthalmoscopy images. Using balanced subset of 4217 images ophthalmology-specific pretrained ViT backbones, this method demonstrates significant improvements in classification accuracy, offering potential broader applications medical imaging. Glaucoma, cataracts are common eye diseases that can cause loss if not treated. These must be identified the early stages to prevent damage progression. paper focuses on accurate identification analysis disparate including using Deep (DL) has been widely used image recognition detection treatment diseases. In study, ResNet50, DenseNet121, Inception-ResNetV2, six variations employed, their performance diagnosing such as retinopathy is evaluated. particular, article uses transformer model an automated diagnose highlighting accuracy pre-trained deep (DTL) structures. The updated ViT#5 augmented-regularized (AugReg ViT-L/16_224) rate 0.00002 outperforms state-of-the-art techniques, obtaining data-based score 98.1% publicly accessible dataset, which includes most categories, other convolutional-based models terms precision, recall, F1 score. research contributes significantly analysis, demonstrating AI enhancing precision disease diagnoses advocating integration artificial intelligence diagnostics.
Language: Английский
Citations
0Biomedical Signal Processing and Control, Journal Year: 2025, Volume and Issue: 105, P. 107542 - 107542
Published: Feb. 3, 2025
Citations
0