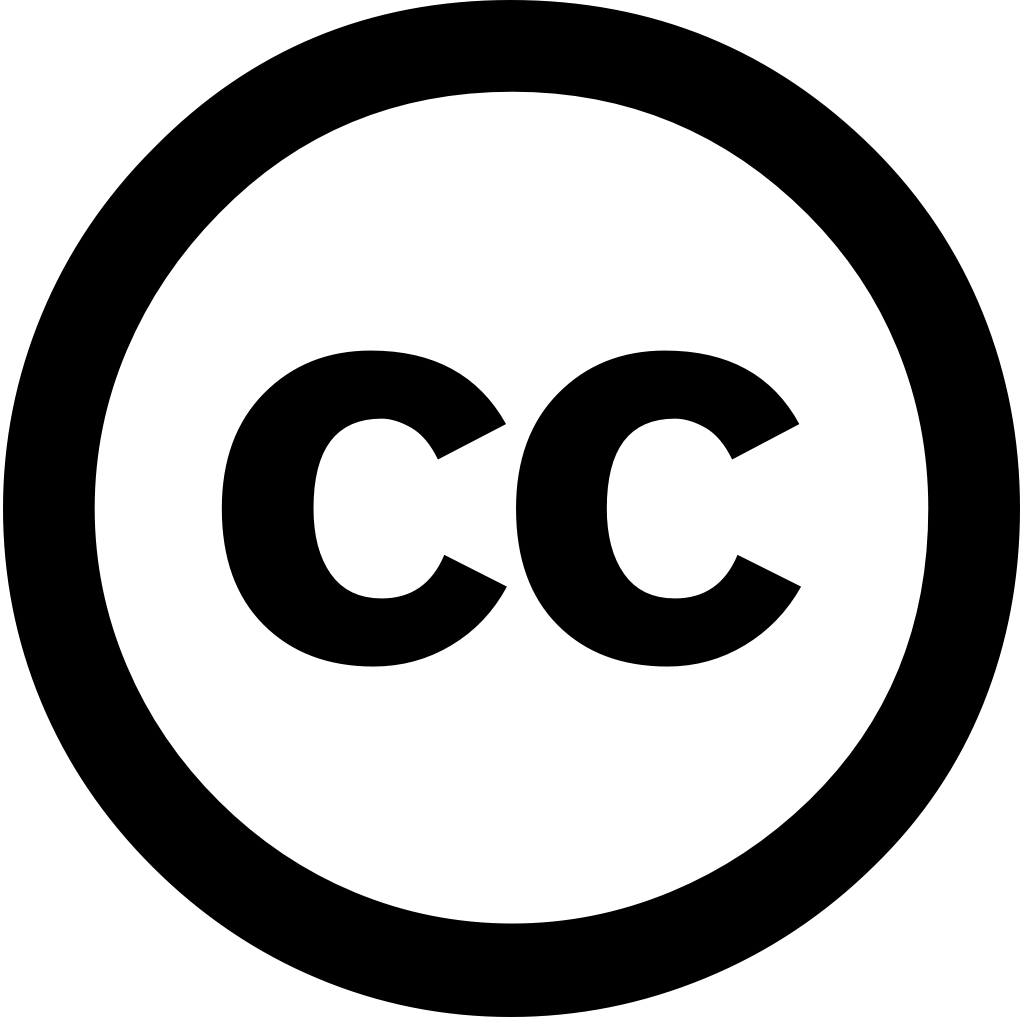
GeoJournal, Journal Year: 2025, Volume and Issue: 90(3)
Published: May 9, 2025
Language: Английский
GeoJournal, Journal Year: 2025, Volume and Issue: 90(3)
Published: May 9, 2025
Language: Английский
Sustainability, Journal Year: 2020, Volume and Issue: 13(1), P. 232 - 232
Published: Dec. 29, 2020
Soil erosion from arable lands removes the top fertile soil layer (comprised of humus/organic matter) and therefore requires fertilizer application which affects overall sustainability. Hence, determination is crucial to planning conservation measures. A modeling approach a suitable alternative estimate loss in ungauged catchments. primarily depends on texture, structure, infiltration, topography, land uses, other erosive forces like water wind. By analyzing these parameters, coupled with geospatial tools, models can storm wise annual average losses. In this study, hilly watershed called Nongpoh was considered objective prioritizing critical hazard areas within micro-catchment based use cover making appropriate management plans for prioritized areas. Two namely Revised Universal Loss Equation (RUSLE) Modified Morgan–Morgan–Finney (MMF) were used input parameters extracted satellite information automatic weather stations. The RUSLE MMF showed similar results estimating loss, except model estimated 7.74% less than watershed. also indicated that study area under severe class, whereas agricultural land, open forest area, scrubland most prone Based prioritization, best developed at catchment scale reducing loss. These findings methodology employed be widely mountainous watersheds around world identifying practices (BMP).
Language: Английский
Citations
36GEOMATICA, Journal Year: 2024, Volume and Issue: 76(2), P. 100017 - 100017
Published: Aug. 10, 2024
Alterations in Land use and cover (LULC) stand out as a key catalyst for shifts global climate patterns, environmental conditions, ecological dynamics. In order to further enhance our comprehension of the effects variability on environment, Remote sensing GIS analytical approaches have been thoroughly explored are reflected an imperative vision. Thus, objective this study is model Uttarakhand's LULC pattern 2032 analyse changes trend between 1992 2022. change mapping was conducted utilizing semi-automated hybrid classification approach high level accuracy which integrates both Maximum likelihood Object based image analysis techniques Landsat datasets. The machine learning Cellular automata Artificial neural networks (CA-ANN) within MOLUSCE plugin QGIS applied future patterns. assessment results showed that overall years 1992, 2002, 2012, 2022 96.94 %, 97.77 98.61 % 98.87 respectively, kappa statistics coefficient 0.92, 0.95, 0.94 0.95 respectively. simulated projected map implies substantially accuracy, with Kappa value 0.77 85.39 correctness. Then, year predicted using CA-ANN. observed alterations significant, characterized by augmentation built-up areas, open land, water bodies, alongside decline snow-covered regions, vegetation cover. Whereas, slight increase seen Forested areas. Planners policy makers aiming accomplish more sustainable efficient management environment will find over prolonged period time be useful asset optimal land planning.
Language: Английский
Citations
4Remote Sensing, Journal Year: 2025, Volume and Issue: 17(3), P. 492 - 492
Published: Jan. 31, 2025
In the past two decades, Islamabad has experienced significant urbanization. As a result of inadequate urban planning and spatial distribution, it significantly influenced land use–land cover (LULC) changes green areas. To assess these changes, there is an increasing need for reliable appropriate information about Landsat imagery categorized into four thematic classes using supervised classification method called support vector machine (SVM): built-up, bareland, vegetation, water. The results change detection post-classification show that city region increased from 6.37% (58.09 km2) in 2000 to 28.18% (256.49 2020, while vegetation decreased 46.97% (428.28 34.77% (316.53 bareland 45.45% (414.37 35.87% (326.49 km2). Utilizing modeler (LCM), forecasts future conditions 2025, 2030, 2035 are predicted. artificial neural network (ANN) model embedded IDRISI software 18.0v based on well-defined backpropagation (BP) algorithm was used simulate sprawl considering historical pattern 2015–2020. Selected landscape morphological measures were quantify analyze structure patterns. According data, area grew at pace 4.84% between 2015 2020 will grow rate 1.47% 2035. This growth metropolitan encroach further bareland. If existing patterns persist over next ten years, drop mean Euclidian Nearest Neighbor Distance (ENN) patches anticipated (from 104.57 m 101.46 2020–2035), indicating accelerated transformation landscape. Future prediction modeling revealed would be huge increase 49% areas until year compared 2000. rapidly urbanizing areas, urgent enhance use laws policies ensure sustainability ecosystem, development, preservation natural resources.
Language: Английский
Citations
0Arabian Journal of Geosciences, Journal Year: 2021, Volume and Issue: 14(6)
Published: March 1, 2021
Language: Английский
Citations
27GeoJournal, Journal Year: 2025, Volume and Issue: 90(3)
Published: May 9, 2025
Language: Английский
Citations
0