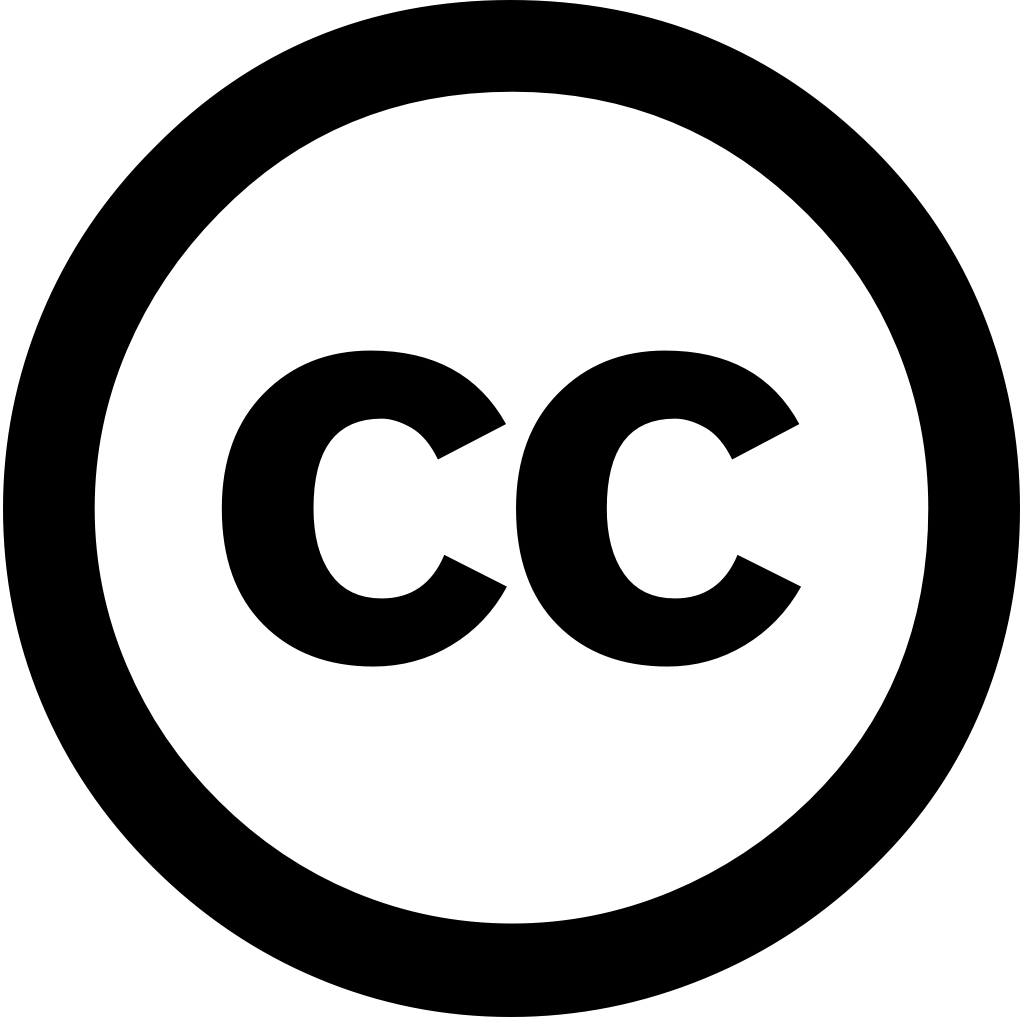
PeerJ, Journal Year: 2024, Volume and Issue: 12, P. e18414 - e18414
Published: Nov. 29, 2024
Ecological niche modeling (ENM) is a valuable tool for inferring suitable environmental conditions and estimating species’ geographic distributions. ENM widely used to assess the potential effects of climate change on species distributions; however, choice algorithm introduces substantial uncertainty, especially since future projections cannot be properly validated. In this study, we evaluated performance seven popular algorithms—Bioclim, generalized additive models (GAM), linear (GLM), boosted regression trees (BRT), Maxent, random forest (RF), support vector machine (SVM)—in transferring across time, using Mexican endemic rodents as model system. We retrospective approach, from near past (1950–1979) more recent (1980–2009) vice versa, evaluate their in both forecasting hindcasting. Consistent with previous studies, our results highlight that input data quality significantly impact accuracy, but most importantly, found varied between While no single outperformed others temporal directions, RF generally showed better forecasting, while Maxent performed hindcasting, though it was sensitive small sample sizes. Bioclim consistently lowest performance. These findings underscore not all or algorithms are suited projections. Therefore, strongly recommend conducting thorough evaluation quality—in terms quantity biases—of interest. Based assessment, appropriate algorithm(s) should carefully selected rigorously tested before proceeding transfers.
Language: Английский