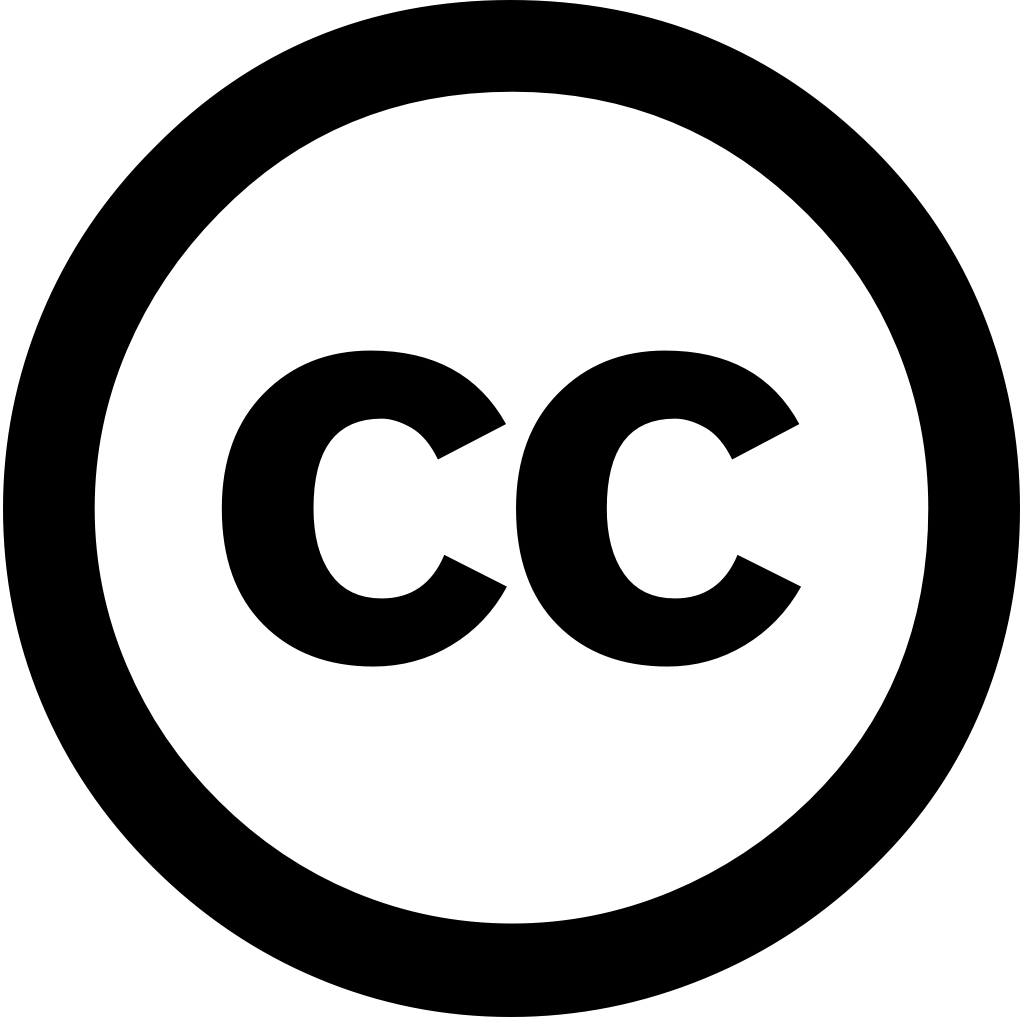
Geomechanics and Geophysics for Geo-Energy and Geo-Resources, Journal Year: 2024, Volume and Issue: 10(1)
Published: Oct. 4, 2024
Language: Английский
Geomechanics and Geophysics for Geo-Energy and Geo-Resources, Journal Year: 2024, Volume and Issue: 10(1)
Published: Oct. 4, 2024
Language: Английский
The Science of The Total Environment, Journal Year: 2024, Volume and Issue: 937, P. 173425 - 173425
Published: May 24, 2024
Language: Английский
Citations
16Energies, Journal Year: 2024, Volume and Issue: 17(15), P. 3768 - 3768
Published: July 31, 2024
Porosity assessment is a vital component for reservoir evaluation in the oil and gas sector, with technological advancement, reliance on conventional methods has decreased. In this regard, research aims to reduce well logging, purposing successive machine learning (ML) techniques precise porosity measurement. So, examines prediction of curves Sui main upper limestone reservoir, utilizing ML approaches such as an artificial neural networks (ANN) fuzzy logic (FL). Thus, input dataset includes gamma ray (GR), neutron (NPHI), density (RHOB), sonic (DT) logs amongst five drilled wells located Qadirpur field. The ANN model was trained using backpropagation algorithm. For FL model, ten bins were utilized, Gaussian-shaped membership functions chosen ideal correspondence geophysical log dataset. closeness fit (C-fit) values ranged from 91% 98%, while exhibited variability 90% 95% throughout wells. addition, similar used evaluate multiple linear regression (MLR) comparative analysis. models achieved robust performance compared MLR, R2 0.955 (FL) 0.988 0.94 (MLR). outcomes indicate that exceed MLR predicting curve. Moreover, significant lowest root mean square error (RMSE) support potency these advanced approaches. This emphasizes authenticity not only enhance natural resource exploitation within region but also hold broader potential worldwide applications assessment.
Language: Английский
Citations
9Sustainability, Journal Year: 2025, Volume and Issue: 17(5), P. 2048 - 2048
Published: Feb. 27, 2025
Natural gas, as a sustainable and cleaner energy source, still holds crucial position in the transition stage. In shale gas exploration, total organic carbon (TOC) content plays role, with log data proving beneficial predicting reservoirs. However, complex coal-bearing layers like marine–continental transitional Shanxi Formation, traditional prediction methods exhibit significant errors. Therefore, this study proposes an advanced, cost- time-saving deep learning approach to predict TOC shale. Five well records from area were used evaluate five machine models: K-Nearest Neighbors (KNNs), Random Forest (RF), Gradient Boosting Decision Tree (GBDT), Extreme (XGB), Deep Neural Network (DNN). The predictive results compared conventional for accurate predictions. Through K-fold cross-validation, ML models showed superior accuracy over models, DNN model displaying lowest root mean square error (RMSE) absolute (MAE). To enhance accuracy, δR was integrated new parameter into models. Comparative analysis revealed that improved DNN-R reduced MAE RMSE by 57.1% 70.6%, respectively, on training set, 59.5% 72.5%, test original model. Williams plot permutation importance confirmed reliability effectiveness of enhanced indicate potential technology valuable tool parameters, especially reservoirs lacking sufficient core samples relying solely basic well-logging data, signifying its effective assessment development.
Language: Английский
Citations
1Canadian Geotechnical Journal, Journal Year: 2024, Volume and Issue: unknown
Published: March 6, 2024
The Mahu sag slope area, which holds significance as an oil and gas resource, still have some underexplored regions because of structural mismatches, presenting a potential challenge to be properly addressed. To resolve, this study conducts comprehensive investigation concerning characteristics, fault combinations, favorable reservoir distribution, control factors, oil-water distribution characteristics within the Triassic Baikouquan Formation, evaluating impact depositional environments sedimentary dynamics on quality. For purpose, constrained sparse spike inversion seismic waveform indication were employed comparatively evaluate reservoirs, further integrating petrophysical geological data with modeling enhance accuracy in complex geology enable high-precision prediction. findings elucidated range Formation location sand bodies, exemplified by well B identified hydrocarbon traps, offering valuable insights into performance. It demonstrated reliable effects considerable predictability power inversion. These outcomes provide strong foundation for future evaluations multi-layer system deployment region serving novel framework subsequent development activities not only but also similar regions.
Language: Английский
Citations
8Geomechanics and Geophysics for Geo-Energy and Geo-Resources, Journal Year: 2024, Volume and Issue: 10(1)
Published: Aug. 1, 2024
Abstract The oil and gas industry relies on accurately predicting profitable clusters in subsurface formations for geophysical reservoir analysis. It is challenging to predict payable complicated geological settings like the Lower Indus Basin, Pakistan. In complex, high-dimensional heterogeneous settings, traditional statistical methods seldom provide correct results. Therefore, this paper introduces a robust unsupervised AI strategy designed identify classify zones using self-organizing maps (SOM) K-means clustering techniques. Results of SOM provided potentials six depositional facies types (MBSD, DCSD, MBSMD, SSiCL, SMDFM, MBSh) based cluster distributions. MBSD DCSD exhibited high similarity achieved maximum effective porosity (PHIE) value ≥ 15%, indicating good rock typing (RRT) features. density-based spatial applications with noise (DBSCAN) showed minimum outliers through meta attributes confirmed reliability generated Shapley Additive Explanations (SHAP) model identified PHIE as most significant parameter was beneficial identifying non-payable zones. Additionally, highlights importance managing distribution across various formations, going beyond simple characterization.
Language: Английский
Citations
7Geo-spatial Information Science, Journal Year: 2024, Volume and Issue: unknown, P. 1 - 21
Published: Oct. 18, 2024
Reservoir characterization is a vital task within the oil and gas industry, with identification of lithofacies in subsurface formations being fundamental aspect this process. However, complex geological environments high dimensions, such as Lower Indus Basin Pakistan, poses notable challenge, especially when dealing limited data. To address issue, we propose four common data-driven machine learning approaches: multi-resolution graph-based clustering (MRGC), artificial neural networks (ANN), K-nearest neighbors (KNN), self-organizing map (SOM). We utilized these proposed approaches to assess their performance scenarios varying core sample availability, specifically evaluating effectiveness identifying Goru formation middle Basin. The study reveals that number samples, MRGC preferred choice, while KNN or more suitable for larger datasets. results demonstrate superior specified environment, SOM following closely behind, ANN exhibiting comparatively lower efficacy. accurate from selected model complemented by application truncated Gaussian simulation method facies modeling. Comparative confirm excellent agreement between well logs electro-facies obtained volume. This highlights crucial role selecting right approach precise modeling environments. comparative analysis provides practitioners petroleum industry insights into strengths limitations each method, enhancing existing knowledge. In conclusion, research emphasizes significance comprehensive selection advancing diverse areas, ultimately benefiting broader field industry.
Language: Английский
Citations
6Journal of Applied Geophysics, Journal Year: 2024, Volume and Issue: 230, P. 105502 - 105502
Published: Aug. 31, 2024
Language: Английский
Citations
5Frontiers in Earth Science, Journal Year: 2025, Volume and Issue: 12
Published: Jan. 15, 2025
Introduction The Yanchang gas field in the southeastern Ordos Basin is an important region for natural exploration due to presence of Upper Paleozoic coal-bearing source rocks. This study systematically evaluates these rocks terms organic matter abundance, type, and thermal maturity assess their hydrocarbon generation potential. Methods Organic geochemical analyses were performed determine total carbon (TOC) content, kerogen Benxi Taiyuan Formations specifically targeted detailed evaluation. Kerogen maceral analysis was used classify types, while assessed through vitrinite reflectance (Ro) values. Results results indicate that exhibit high TOC with showing excellent identified predominantly Type III (humic) some II2 (humic-sapropelic) components, suggesting a strong capacity generation. Thermal revealed have reached overmature dry stage, signifying advanced evolution. Discussion findings highlight central southern parts as promising targets, characterized by favorable type indices, Ro These features collectively demonstrate significant potential Conclusion represent highly prospective target exploration, particularly regions properties.
Language: Английский
Citations
0Natural Resources Research, Journal Year: 2025, Volume and Issue: unknown
Published: April 13, 2025
Language: Английский
Citations
0Journal of Alloys and Compounds, Journal Year: 2025, Volume and Issue: unknown, P. 180709 - 180709
Published: May 1, 2025
Language: Английский
Citations
0