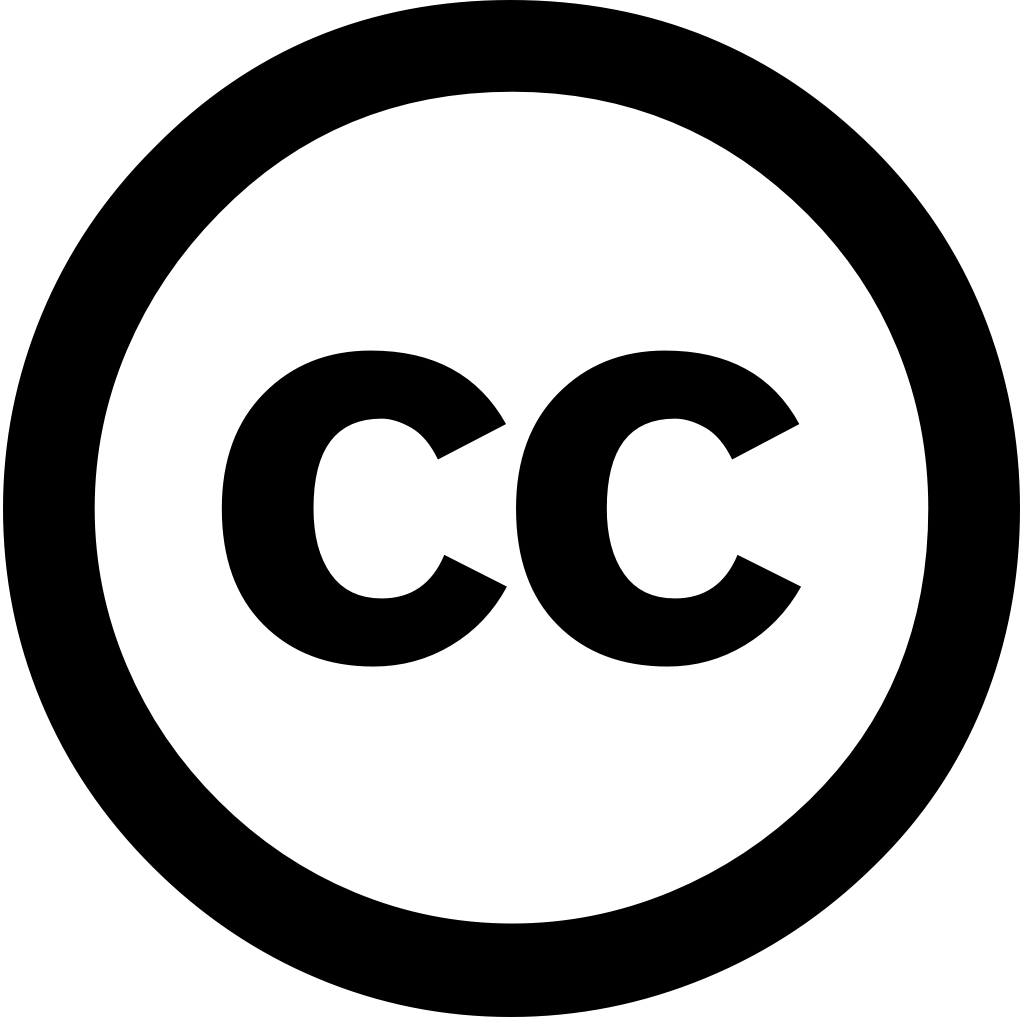
Research Square (Research Square), Journal Year: 2024, Volume and Issue: unknown
Published: Nov. 11, 2024
Language: Английский
Research Square (Research Square), Journal Year: 2024, Volume and Issue: unknown
Published: Nov. 11, 2024
Language: Английский
Journal of Hydrology, Journal Year: 2023, Volume and Issue: 624, P. 129969 - 129969
Published: July 20, 2023
Language: Английский
Citations
120Journal of Hydrology, Journal Year: 2023, Volume and Issue: 625, P. 129956 - 129956
Published: July 19, 2023
Language: Английский
Citations
64Water Resources Management, Journal Year: 2025, Volume and Issue: unknown
Published: March 11, 2025
Language: Английский
Citations
1Journal of Hydroinformatics, Journal Year: 2023, Volume and Issue: 26(1), P. 255 - 283
Published: Dec. 11, 2023
Abstract Accurate runoff prediction is vital in efficiently managing water resources. In this paper, a hybrid model combining complete ensemble empirical mode decomposition with adaptive noise, variational decomposition, CABES, and long short-term memory network (CEEMDAN-VMD-CABES-LSTM) proposed. Firstly, CEEMDAN used to decompose the original data, high-frequency component decomposed using VMD. Then, each input into LSTM optimized by CABES for prediction. Finally, results of individual predictions are combined reconstructed produce monthly predictions. The employed predict at Xiajiang hydrological station Yingluoxia station. A comprehensive comparison conducted other models including back propagation (BP), LSTM, etc. assessment model's performance uses four evaluation indexes. Results reveal that CEEMDAN-VMD-CABES-LSTM showcased highest forecast accuracy among all evaluated. Compared single root mean square error (RMSE) absolute percentage (MAPE) decreased 71.09 65.26%, respectively, RMSE MAPE 65.13 40.42%, respectively. R NSEC both sites near 1.
Language: Английский
Citations
20Journal of Hydrology Regional Studies, Journal Year: 2023, Volume and Issue: 49, P. 101492 - 101492
Published: Aug. 1, 2023
Kaidu River catchment in the Tianshan Mountain, northwestern China. This paper compared applicability and accuracy of four machine learning models two hydrological ones to simulate daily streamflow extreme catchment. The are Support Vector Regression (SVR), eXtreme Gradient Boosting (XGBoost), Random Forests (RF), Long Short-Term Memory (LSTM), while Soil Water Assessment Tool (SWAT) extended SWAT with a glacier dynamic module (SWAT-Glacier). LSTM achieved better model performance simulating than SWAT-Glacier, Kling-Gupta efficiency 0.92, 0.82, 0.80, respectively. Meanwhile, SVR, XGBoost, RF showed satisfactory performance, KGE 0.67, 0.71, 0.70, LSTM, SWAT-Glacier could well annual peak flow (i.e., maximum 1-day 5-day average streamflow) but failed mimic minimum 7-day streamflow, PBIAS exceeding 28%. Furthermore, all reproduce dates extremes. Nevertheless, using quantile loss function resulted significantly improved low indices, that mean squared error as function. Overall, be good alternative for data-scarce catchments.
Language: Английский
Citations
19Water Resources Management, Journal Year: 2024, Volume and Issue: 38(7), P. 2471 - 2488
Published: Feb. 10, 2024
Language: Английский
Citations
7Water Resources Management, Journal Year: 2023, Volume and Issue: 38(1), P. 269 - 286
Published: Dec. 1, 2023
Language: Английский
Citations
15Hydrological Sciences Journal, Journal Year: 2023, Volume and Issue: 68(3), P. 488 - 506
Published: Jan. 27, 2023
ABSTRACTA novel smoothing-based long short-term memory (Smooth-LSTM) framework for flood forecasting up to five days ahead is proposed, and compared with the benchmark LSTM (LSTM) model, an Artificial Neural Network (ANN) conceptual Nedbør Afstrømnings Model (MIKE11 NAM)-Hydrodynamic (HD) (MIKE) hydrological model. This was tested in typical middle Mahanadi River basin (India), which has a tropical monsoon-type climate. Variation of training loss indicated network higher learning ability at smaller batch sizes. The Smooth-LSTM model could predict streamflow Nash-Sutcliffe efficiency 0.82–0.87 lead time better reproduction observed crucial high peak floods, whereas corresponding MIKE, ANN model-based forecasts were acceptable only four-, three- one-day times, respectively. Overall, found be robust operational forecasting, lower uncertainty least sensitivity redundant input information.KEYWORDS: ANNflood forecastinglong (LSTM)MIKE 11 NAM-HDsmoothing windows Editor A. Castellarin Associate O. KisiEditor KisiAcknowledgementsThe authors sincerely thank Hirakud Dam Circle (HDC), Department Water Resources (Prachi Division), Odisha, Central Commission (CWC) India Meteorological (IMD) providing necessary datasets carry out study.Disclosure statementNo potential conflict interest reported by authors.Data availability statementAll data, models, code generated or used during study appear submitted article.Supplementary materialSupplemental data this article can accessed online https://doi.org/10.1080/02626667.2023.2173012
Language: Английский
Citations
11Water, Journal Year: 2024, Volume and Issue: 16(2), P. 364 - 364
Published: Jan. 22, 2024
River flood routing computes changes in the shape of a wave over time as it travels downstream along river. Conventional models, especially hydrodynamic require high quality and quantity input data, such measured hydrologic series, geometric hydraulic structures, hydrological parameters. Unlike physically based machine learning algorithms, which are data-driven do not much knowledge about underlying physical processes can identify complex nonlinearity between inputs outputs. Due to their higher performance, lower complexity, low computation cost, researchers introduced novel methods single application or hybrid achieve more accurate efficient routing. This paper reviews recent river
Language: Английский
Citations
4IEEE Access, Journal Year: 2025, Volume and Issue: 13, P. 10252 - 10264
Published: Jan. 1, 2025
Language: Английский
Citations
0