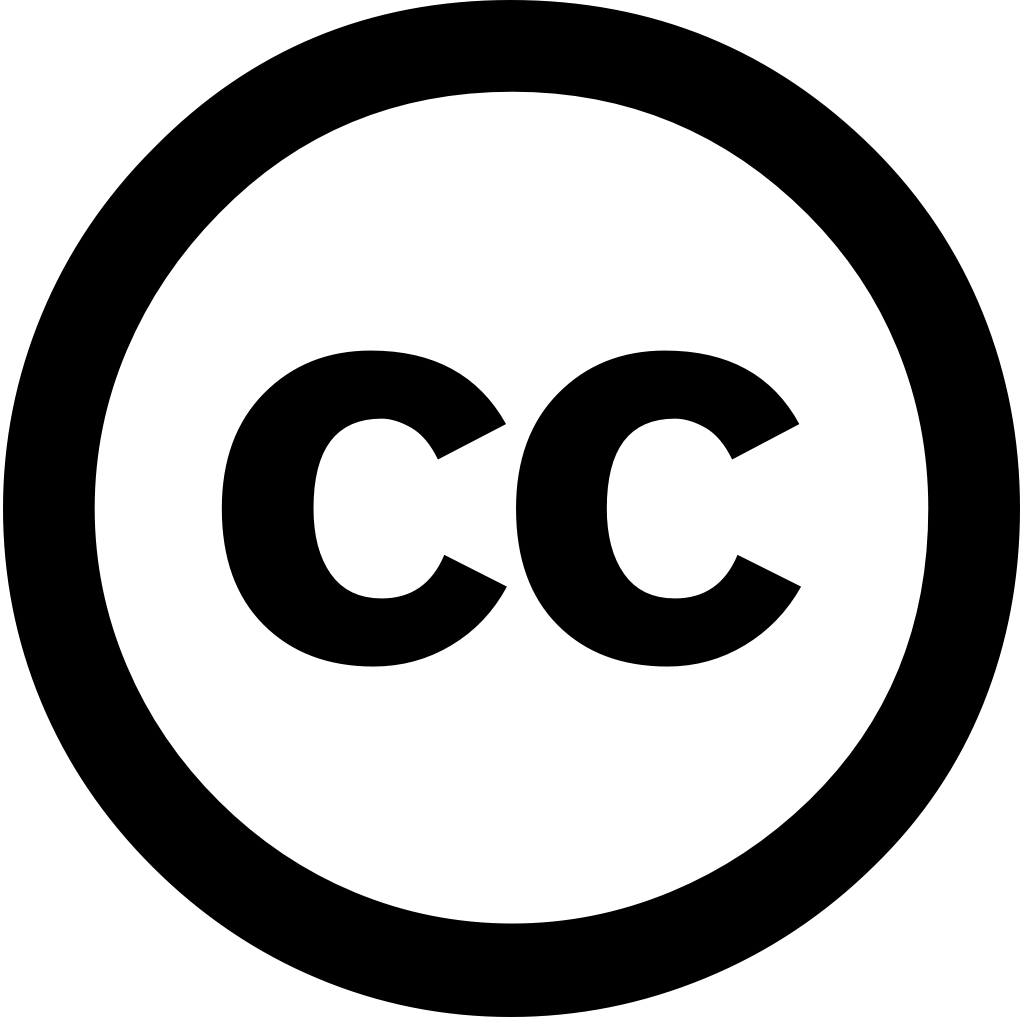
Research Square (Research Square), Journal Year: 2024, Volume and Issue: unknown
Published: Oct. 30, 2024
Language: Английский
Research Square (Research Square), Journal Year: 2024, Volume and Issue: unknown
Published: Oct. 30, 2024
Language: Английский
Computer Modeling in Engineering & Sciences, Journal Year: 2024, Volume and Issue: 139(3), P. 3513 - 3534
Published: Jan. 1, 2024
Chronic kidney disease (CKD) is a major health concern today, requiring early and accurate diagnosis.Machine learning has emerged as powerful tool for detection, medical professionals are increasingly using ML classifier algorithms to identify CKD early.This study explores the application of advanced machine techniques on dataset obtained from University California, UC Irvine Machine Learning repository.The research introduces TrioNet, an ensemble model combining extreme gradient boosting, random forest, extra tree classifier, which excels in providing highly predictions CKD.Furthermore, K nearest neighbor (KNN) imputer utilized deal with missing values while synthetic minority oversampling (SMOTE) used class-imbalance problems.To ascertain efficacy proposed model, comprehensive comparative analysis conducted various models.The TrioNet KNN SMOTE outperformed other models 98.97% accuracy detecting CKD.This in-depth demonstrates model's capabilities underscores its potential valuable diagnosis CKD.
Language: Английский
Citations
10Scientific Reports, Journal Year: 2025, Volume and Issue: 15(1)
Published: Feb. 5, 2025
Abstract Chronic kidney disease (CKD) is a global health concern with early detection playing pivotal role in effective management. Machine learning models demonstrate promise CKD detection, yet the impact on and classification using different sets of clinical features remains under-explored. In this study, we focus creatinine prediction three features: at-home, monitoring, laboratory. We employ artificial neural networks (ANNs) random forests (RFs) dataset 400 patients 25 input features, which divide into feature sets. Using 10-fold cross-validation, calculate metrics such as accuracy, true positive rate (TPR), negative (TNR), mean squared error. Our results reveal RF achieves superior accuracy (92.5%) at-home over ANNs (82.9%). achieve higher TPR (92.0%), but lower TNR (67.9%) compared RFs (90.0% 95.8%, respectively). For monitoring laboratory both methods accuracies exceeding 98%. The R2 score for regression approximately 0.3 than features. Feature importance analysis identifies key variables hemoglobin blood urea, comorbidities hypertension diabetes mellitus, agreement previous studies. models, particularly RFs, exhibit diagnosis highlight significant detection. Moreover, may assist screening general population features—potentially increasing CKD, thus improving patient care offering hope more approach to managing prevalent condition.
Language: Английский
Citations
1Genes, Journal Year: 2024, Volume and Issue: 15(1), P. 123 - 123
Published: Jan. 19, 2024
MicroRNAs (miRNAs) and long non-coding RNAs (lncRNAs) are two crucial classes of transcripts that belong to the major group (ncRNAs). These RNA molecules have significant influence over diverse molecular processes due their role as regulators gene expression. However, dysregulated expression these ncRNAs constitutes a fundamental factor in etiology progression wide variety multifaceted human diseases, including kidney diseases. In this context, past years, compelling evidence has shown miRNAs lncRNAs could be prospective targets for development next-generation drugs against diseases they participate number disease-associated processes, such podocyte nephron death, renal fibrosis, inflammation, transition from acute injury chronic disease, vascular changes, sepsis, pyroptosis, apoptosis. Hence, current review, we critically analyze recent findings concerning therapeutic inferences pathophysiological context Additionally, with aim driving advances formulation ncRNA-based tailored management discuss some key challenges future prospects should addressed forthcoming investigations.
Language: Английский
Citations
8Communications in computer and information science, Journal Year: 2025, Volume and Issue: unknown, P. 313 - 326
Published: Jan. 1, 2025
Language: Английский
Citations
0Computer Methods and Programs in Biomedicine Update, Journal Year: 2025, Volume and Issue: unknown, P. 100173 - 100173
Published: Jan. 1, 2025
Language: Английский
Citations
0Scientific Reports, Journal Year: 2025, Volume and Issue: 15(1)
Published: April 25, 2025
Language: Английский
Citations
0Measurement Sensors, Journal Year: 2023, Volume and Issue: 27, P. 100715 - 100715
Published: Feb. 27, 2023
CKD (chronic kidney disease) have been identified as a serious public health concern globally. Machine learning models can successfully enable physicians to reach this aim because of their rapid and accurate identification performance. In paper, KNN (K Nearest Neighbor) imputations, which choose multiple full samples with the most comparable values for replacing missing utilized in work. Additionally, conventional MLT (Machine Learning Technique) need produce better outcomes than DLT (Deep technique). The prediction step may be sluggish, sensitive size data, filled irrelevant information when there is lot data. Tensor factorization ANFIS (Adaptive Neuro-Fuzzy Inference System) are added data imputations address issue. technique addressing feature selections called AWDBOA Weight Dynamic Butterfly Optimization Algorithm), inspired by nature. At same time, adjustable weights selection from dataset added. A modified form NN NWCNN (Novel Convolution Neural Network) classifier uses convolution rather standard matrix multiplication at least one its layers. layers hidden NWCNN, kernel functions improve or fine-tune classifier's parameters. was imperturbable UCI (University California, Irvine) ML repository had large number misplaced values. This work's proposed evaluated terms precision, recall, F1-score, sensitivitiy, specificity, accuracy 99.17%, 98.71%, 98.94%, 99.10%, 99.04% were obtained higher other models.
Language: Английский
Citations
4Network Modeling Analysis in Health Informatics and Bioinformatics, Journal Year: 2024, Volume and Issue: 13(1)
Published: April 25, 2024
Language: Английский
Citations
1Optical and Quantum Electronics, Journal Year: 2023, Volume and Issue: 55(13)
Published: Oct. 7, 2023
Language: Английский
Citations
2Computers & Electrical Engineering, Journal Year: 2024, Volume and Issue: 122, P. 109933 - 109933
Published: Dec. 2, 2024
Language: Английский
Citations
0