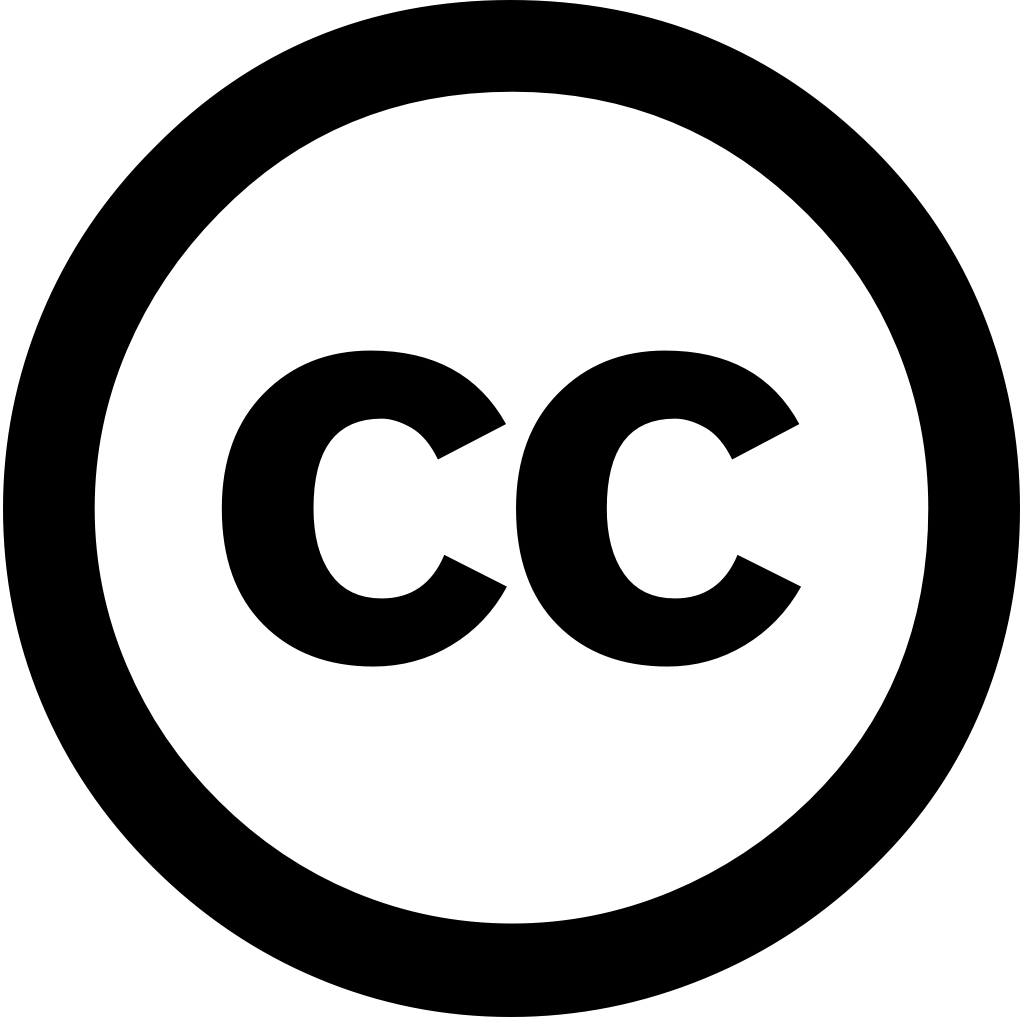
Journal Of Big Data, Journal Year: 2024, Volume and Issue: 11(1)
Published: Dec. 20, 2024
Language: Английский
Journal Of Big Data, Journal Year: 2024, Volume and Issue: 11(1)
Published: Dec. 20, 2024
Language: Английский
Journal of Hazardous Materials, Journal Year: 2024, Volume and Issue: 474, P. 134865 - 134865
Published: June 12, 2024
Language: Английский
Citations
18Journal of Business Logistics, Journal Year: 2025, Volume and Issue: 46(1)
Published: Jan. 1, 2025
ABSTRACT The Journal of Business Logistics has been the top location for publishing logistics and supply chain‐related technological research over forty years. With digital transformation, reshoring manufacturing, labor shortages, decreasing birth rates, aging workforces, companies are increasingly adopting artificial intelligence‐supported robotics to increase ability chains react quickly effectively changes in customer demand, market conditions, or disruptions. This paper analyzes use hardware robots across fulfillment process. study addresses evolution robotic training from explicit programming machine learning continues with a detailed discussion generative learning. We then provide an overview key driven by models that used examines challenges robot adoption presents organizations concludes directions further using Theory Resource Orchestration.
Language: Английский
Citations
2Scientific Reports, Journal Year: 2025, Volume and Issue: 15(1)
Published: Jan. 27, 2025
Language: Английский
Citations
2Advances in logistics, operations, and management science book series, Journal Year: 2024, Volume and Issue: unknown, P. 33 - 47
Published: June 30, 2024
This chapter explores the application of quantum machine learning (QML) techniques for demand prediction in supply chain networks. Traditional forecasting methods often struggle to capture intricate dynamics and uncertainties present modern chains. By leveraging computational power probabilistic nature computing, coupled with flexibility adaptability algorithms, organizations can enhance accuracy efficiency their processes. provides an overview QML methodologies tailored specifically networks, highlighting advantages over classical approaches. Through case studies practical examples, demonstrates how enable make more informed decisions, optimize inventory levels, improve overall performance.
Language: Английский
Citations
4Platforms, Journal Year: 2025, Volume and Issue: 3(2), P. 6 - 6
Published: April 9, 2025
Efficient supply chain management (SCM) is essential for enterprises seeking to enhance operational efficiency, reduce costs, and mitigate risks while ensuring product quality customer satisfaction. Addressing concerns within the proactively helps minimize rework, recalls, returns, leading significant cost savings improved profitability. This study presents a machine learning (ML)-driven predictive analytics framework designed forecast defect rates optimize control processes. The research leverages dataset sourced from real-world fashion beauty startup, hosted in public repository. employs advanced ML algorithms, including extreme gradient boosting (XGBoost), support vector machines (SVMs), random forests (RFs), accurately predict derive actionable insights optimization. Results demonstrate effectiveness of improving management, enabling rates, return on investment (ROI). proposed be scalable transferable, adaptability across various industries, fashion, e-commerce, manufacturing. These findings underscore economic benefits integrating into control, offering data-driven, proactive approach achieving high-efficiency, high-quality operations.
Language: Английский
Citations
0Cureus Journal of Computer Science., Journal Year: 2025, Volume and Issue: unknown
Published: April 16, 2025
Language: Английский
Citations
0Journal of improved oil and gas recovery technology., Journal Year: 2024, Volume and Issue: 7(5), P. 47 - 57
Published: Sept. 20, 2024
Our study presents a data-driven framework designed to simultaneously enhance supply chain resilience and optimize operational efficiency. By addressing key gaps in existing research, particularly the integration of risk management resource optimization across entire chain, this work offers comprehensive approach improving robustness. The was empirically tested within context Company A's global product operations, where we quantified economic impact underutilized production capacities assessed benefits strategic reallocation. analysis demonstrated that by optimizing idle lines, utilization could be improved 18%, resulting annual cost savings approximately $1.2 million. Additionally, enhanced overall 25%, as evidenced reduced recovery times continuity during disruptions. These findings not only provide empirical support for framework's effectiveness but also offer practical insights businesses seeking strengthen their chains face increasing uncertainties. research contributes theoretical advancement efficiency while offering actionable strategies industry practitioners. proposed serves scalable model adaptable various contexts, thereby enhancing competitiveness enterprises an increasingly volatile market environment.
Language: Английский
Citations
12022 International Congress on Human-Computer Interaction, Optimization and Robotic Applications (HORA), Journal Year: 2024, Volume and Issue: 1, P. 1 - 9
Published: May 23, 2024
Supply chain management (SCM) poses significant challenges in optimizing operations and enhancing efficiency. While previous research has explored the application of machine learning (ML) techniques SCM, there remains a gap understanding impact feature engineering on model performance. In this study, we address by investigating various ML algorithms, including linear regression, decision trees, random forests, support vector machines (SVM), deep neural networks, predicting key SCM metrics. Leveraging dataset from Fashion Beauty startup, our approach emphasizes selection to improve prediction accuracy. Our findings indicate notable enhancements performance post-feature engineering, with networks exhibiting highest accuracy, achieving mean absolute error (MAE) 40 root square (RMSE) 50. This contributes advancing ML-driven underscores importance predictive models for real-world applications.
Language: Английский
Citations
0Journal Of Big Data, Journal Year: 2024, Volume and Issue: 11(1)
Published: Dec. 20, 2024
Language: Английский
Citations
0