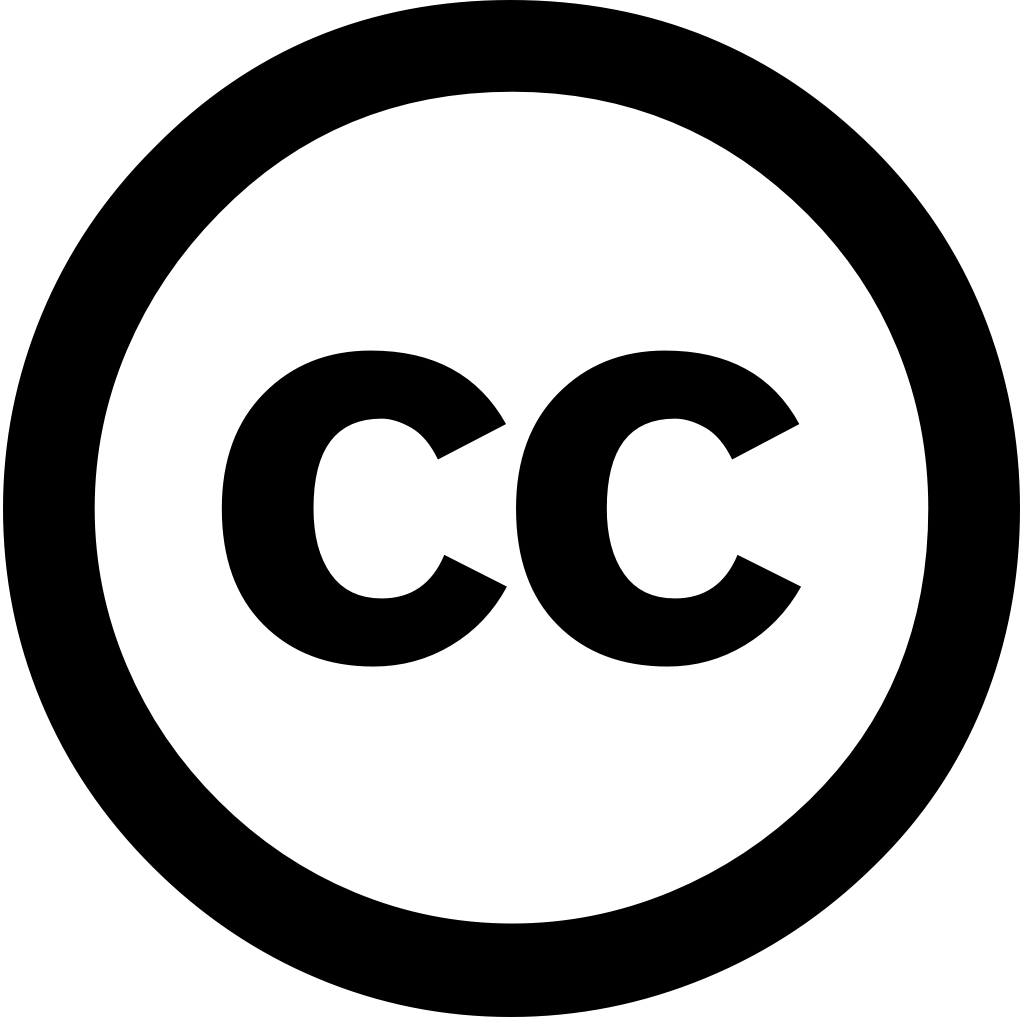
Actuators, Journal Year: 2024, Volume and Issue: 13(10), P. 413 - 413
Published: Oct. 13, 2024
In fields such as manufacturing and aerospace, remaining useful life (RUL) prediction estimates the failure time of high-value assets like industrial equipment aircraft engines by analyzing series data collected from various sensors, enabling more effective predictive maintenance. However, significant temporal diversity operational complexity during operation make it difficult for traditional single-scale, single-dimensional feature extraction methods to effectively capture complex dependencies multi-dimensional interactions. To address this issue, we propose a Dual-Path Interaction Network, integrating Multiscale Temporal-Feature Convolution Fusion Module (MTF-CFM) Dynamic Weight Adaptation (DWAM). This approach adaptively extracts information across different scales, interaction information. Using Commercial Modular Aero-Propulsion System Simulation (C-MAPSS) dataset comprehensive performance evaluation, our method achieved RMSE values 0.0969, 0.1316, 0.086, 0.1148; MAPE 9.72%, 14.51%, 8.04%, 11.27%; Score results 59.93, 209.39, 67.56, 215.35 four categories. Furthermore, MTF-CFM module demonstrated an average improvement 7.12%, 10.62%, 7.21% in RMSE, MAPE, multiple baseline models. These validate effectiveness potential proposed model improving accuracy robustness RUL prediction.
Language: Английский