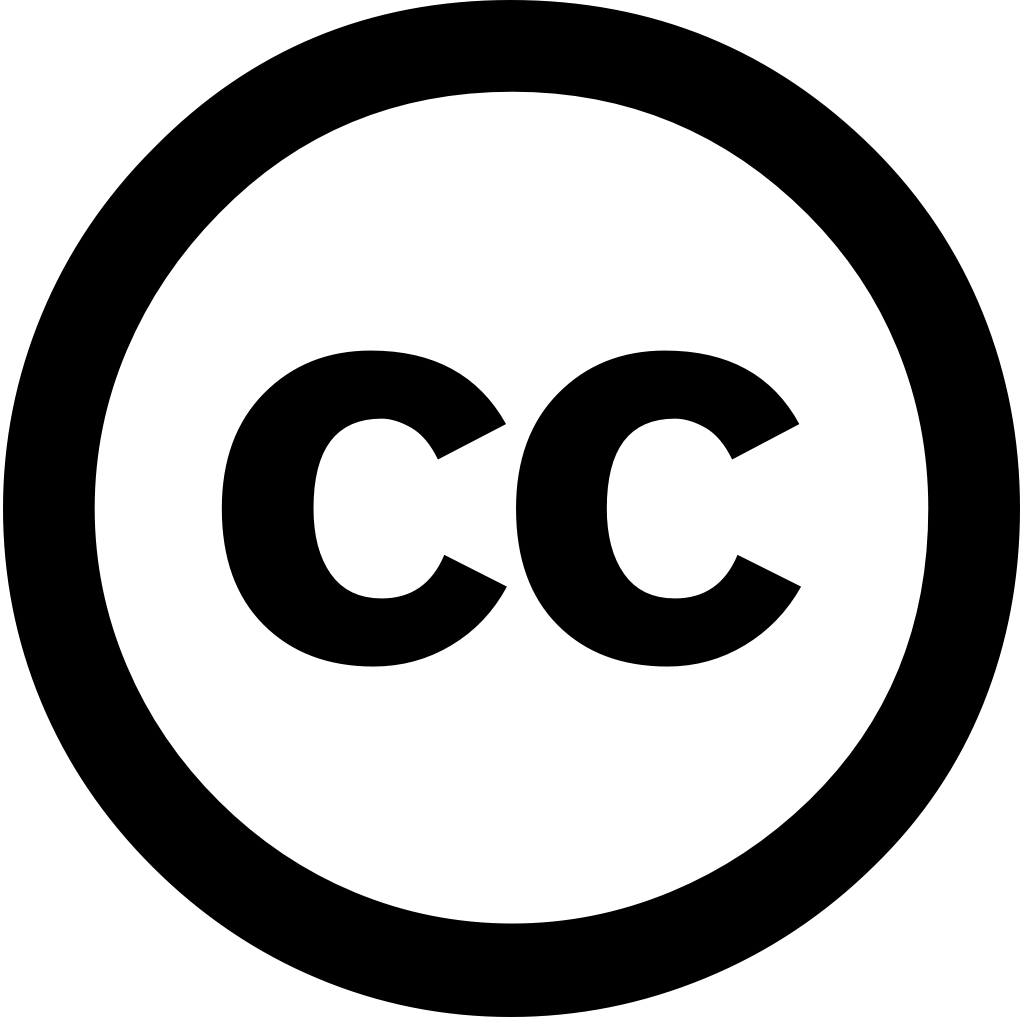
Applied Computing and Geosciences, Journal Year: 2024, Volume and Issue: unknown, P. 100208 - 100208
Published: Nov. 1, 2024
Language: Английский
Applied Computing and Geosciences, Journal Year: 2024, Volume and Issue: unknown, P. 100208 - 100208
Published: Nov. 1, 2024
Language: Английский
Earth Science Informatics, Journal Year: 2024, Volume and Issue: unknown
Published: June 12, 2024
Abstract
Climate
projections
at
fine
spatial
resolutions
are
required
to
conduct
accurate
risk
assessment
for
critical
infrastructure
and
design
adaptation
planning.
Generating
these
using
advanced
Earth
system
models
(ESM)
requires
significant
computational
resources.
To
address
this
issue,
various
statistical
downscaling
techniques
have
been
introduced
generate
fine-resolution
data
from
coarse-resolution
simulations.
In
study,
we
evaluate
compare
five
deep
learning-based
techniques,
namely,
super-resolution
convolutional
neural
networks,
fast
network
ESM,
efficient
sub-pixel
network,
enhanced
residual
(EDRN),
generative
adversarial
(SRGAN).
These
applied
a
dataset
generated
by
the
Energy
Exascale
System
Model
(E3SM),
focusing
on
key
surface
variables
such
as
temperature,
shortwave
heat
flux,
longwave
flux.
Models
trained
validated
paired
(0.25
$$^{\circ
}$$
Language: Английский
Citations
1Intelligent Marine Technology and Systems, Journal Year: 2024, Volume and Issue: 2(1)
Published: Sept. 9, 2024
Language: Английский
Citations
1Frontiers in Earth Science, Journal Year: 2024, Volume and Issue: 12
Published: Nov. 20, 2024
Identifying lithology is crucial for geological exploration, and the adoption of artificial intelligence progressively becoming a refined approach to automate this process. A key feature strategy leveraging population search algorithms fine-tune hyperparameters, thus boosting prediction accuracy. Notably, Bayesian optimization has been applied first time select most effective learning parameters neural network classifiers used identification. This technique utilizes capability utilize past classification outcomes enhance models performance based on physical calculated from well log data. In comparison architectures, Bayesian-optimized (BOANN) demonstrably achieved superior accuracy in validation significantly outperformed non-optimized wide, bilayer, tri-layer configurations, indicating that incorporating can advance lithofacies recognition, offering more accurate intelligent solution identifying lithology.
Language: Английский
Citations
1Energy Geoscience, Journal Year: 2024, Volume and Issue: unknown, P. 100364 - 100364
Published: Dec. 1, 2024
Language: Английский
Citations
1International Journal of Multiphase Flow, Journal Year: 2024, Volume and Issue: 180, P. 104952 - 104952
Published: Aug. 5, 2024
Fluid Dynamics is a key scientific field to multitudes of engineering applications. Experimental work in this requires careful set-up and expensive image-capturing equipment, particularly when considering the finer details complex phenomena. In work, we study application super-resolution Generative Adversarial Networks (GANs) achieve high-resolution results by upscaling lower-resolution experimental images. We train GANs proposed for natural images on bubbly flow dataset compare common evaluation metrics domain expert assessments upscaled find that these models promising results, as evaluated experts, transfer learning from translates better performance overall. Attention mechanisms are found be useful recreating sharper details. On other hand, traditional align poorly with perception quality, signaling need systematic methodologies domain.
Language: Английский
Citations
0Applied Computing and Geosciences, Journal Year: 2024, Volume and Issue: 23, P. 100193 - 100193
Published: Sept. 1, 2024
Language: Английский
Citations
0Frontiers in Earth Science, Journal Year: 2024, Volume and Issue: 12
Published: Dec. 12, 2024
Experimental variogram modelling is an essential process in geostatistics. The use of artificial intelligence (AI) a new and advanced way automating experimental modelling. One part this AI approach the population search algorithms to fine-tune hyperparameters for better prediction performing. We Bayesian optimization first time find optimal learning parameters more precise neural network regressor goal leverage capability consider previous regression results improve output using three variograms as inputs one training, calculated from ore grades four orebodies, characterised by same genetic aspect. In comparison architectures, Bayesian-optimized demonstrably achieved superior Coefficient determination validation 78.36%. This significantly outperformed non-optimized wide, bilayer, tri-layer configurations, which yielded 32.94%, 14.00%, −46.03% determination, respectively. improved reliability demonstrates its superiority over traditional, regressors, indicating that incorporating can advance modelling, thus offering accurate intelligent solution, combining geostatistics specifically machine
Language: Английский
Citations
02021 IEEE International Conference on Big Data (Big Data), Journal Year: 2024, Volume and Issue: unknown, P. 6011 - 6020
Published: Dec. 15, 2024
Language: Английский
Citations
0Published: Jan. 1, 2024
Download This Paper Open PDF in Browser Add to My Library Share: Permalink Using these links will ensure access this page indefinitely Copy URL DOI
Language: Английский
Citations
0Published: Jan. 1, 2024
Download This Paper Open PDF in Browser Add to My Library Share: Permalink Using these links will ensure access this page indefinitely Copy URL DOI
Language: Английский
Citations
0