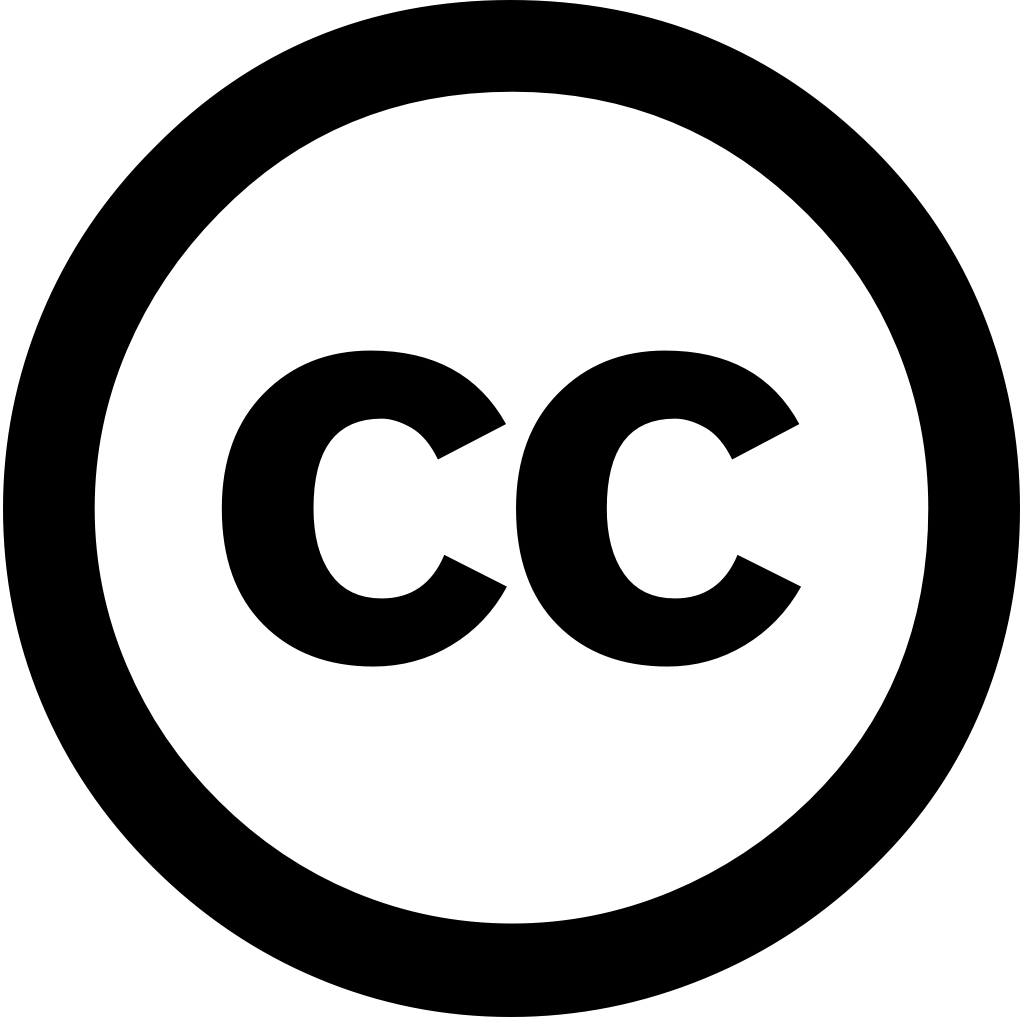
IEEE Access, Journal Year: 2024, Volume and Issue: 12, P. 128602 - 128617
Published: Jan. 1, 2024
Language: Английский
IEEE Access, Journal Year: 2024, Volume and Issue: 12, P. 128602 - 128617
Published: Jan. 1, 2024
Language: Английский
Journal of Statistical Theory and Practice, Journal Year: 2025, Volume and Issue: 19(1)
Published: Jan. 23, 2025
Language: Английский
Citations
2Journal of Economy and Technology, Journal Year: 2025, Volume and Issue: unknown
Published: Feb. 1, 2025
Language: Английский
Citations
0Published: Jan. 1, 2025
Language: Английский
Citations
0Scientific Reports, Journal Year: 2025, Volume and Issue: 15(1)
Published: March 7, 2025
Skin lesion segmentation is crucial for identifying and diagnosing skin diseases. Accurate aids in localizing diseases, monitoring morphological changes, extracting features further diagnosis, especially the early detection of cancer. This task challenging due to irregularity lesions dermatoscopic images, significant color variations, boundary blurring, other complexities. Artifacts like hairs, blood vessels, air bubbles complicate automatic segmentation. Inspired by U-Net its variants, this paper proposes a Multiscale Input Fusion Residual Attention Pyramid Convolution Network (MRP-UNet) dermoscopic image MRP-UNet includes three modules: Module (MIF), Res2-SE Module, Dilated (PDC). The MIF module processes different sizes morphologies fusing input information from various scales. integrates Res2Net SE mechanisms enhance multi-scale feature extraction. PDC captures at receptive fields through pyramid dilated convolution, improving accuracy. Experiments on ISIC 2016, 2017, 2018, PH2, HAM10000 datasets show that outperforms methods. Ablation studies confirm effectiveness main modules. Both quantitative qualitative analyses demonstrate MRP-UNet's superiority over state-of-the-art enhances combining multiscale fusion, residual attention, convolution. It achieves higher accuracy across multiple datasets, showing promise disease diagnosis improved patient outcomes.
Language: Английский
Citations
0Biomedical Signal Processing and Control, Journal Year: 2025, Volume and Issue: 108, P. 107961 - 107961
Published: April 27, 2025
Language: Английский
Citations
0Exploration of Medicine, Journal Year: 2024, Volume and Issue: unknown, P. 694 - 708
Published: Oct. 25, 2024
Aim: Skin lesion segmentation is critical for early skin cancer detection. Challenges in automatic from dermoscopic images include variations color, texture, and artifacts of indistinct boundaries. This study aims to develop evaluate MUCM-Net, a lightweight efficient model segmentation, leveraging Mamba state-space models integrated with UCM-Net architecture optimized mobile deployment Methods: MUCM-Net combines Convolutional Neural Networks (CNNs), multi-layer perceptions (MLPs), elements into hybrid feature learning module. Results: The was trained tested on the International Imaging Collaboration (ISIC) 2017 ISIC2018 datasets, consisting 2,000 2,594 images, respectively. Critical metrics evaluation included Dice Similarity Coefficient (DSC), sensitivity (SE), specificity (SP), accuracy (ACC). model’s computational efficiency also assessed by measuring Giga Floating-point Operations Per Second (GFLOPS) number parameters. demonstrated superior performance an average DSC 0.91 ISIC2017 dataset 0.89 dataset, outperforming existing models. It achieved high SE (0.93), SP (0.95), ACC (0.92) low demands (0.055–0.064 GFLOPS). Conclusions: innovative Mamba-UCM layer significantly enhanced while maintaining that suitable devices. establishes new standard balancing exceptional performance. Its ability perform well devices makes it scalable tool detection resource-limited settings. open-source availability supports further research collaboration, promoting advances health diagnostics fight against cancer. source code will be posted https://github.com/chunyuyuan/MUCM-Net.
Language: Английский
Citations
2Applied Sciences, Journal Year: 2024, Volume and Issue: 14(24), P. 11474 - 11474
Published: Dec. 10, 2024
Image classification usually requires connectivity and access to the cloud, which is often limited in many parts of world, including hard-to-reach rural areas. Tiny machine learning (tinyML) aims solve this problem by hosting artificial intelligence (AI) assistants on constrained devices, eliminating issues processing data within device itself, without Internet or cloud access. This study explores use tinyML provide healthcare support with low-spec devices low-connectivity environments, focusing diagnosis skin diseases ethical AI a setting. To investigate this, images lesions were used train model for classifying visually detectable (VDDs). The weights then offloaded Raspberry Pi webcam attached, be It was found that developed prototype achieved test accuracy 78% when trained HAM10000 dataset, 85% ISIC 2020 Challenge dataset.
Language: Английский
Citations
1IEEE Access, Journal Year: 2024, Volume and Issue: 12, P. 128602 - 128617
Published: Jan. 1, 2024
Language: Английский
Citations
0