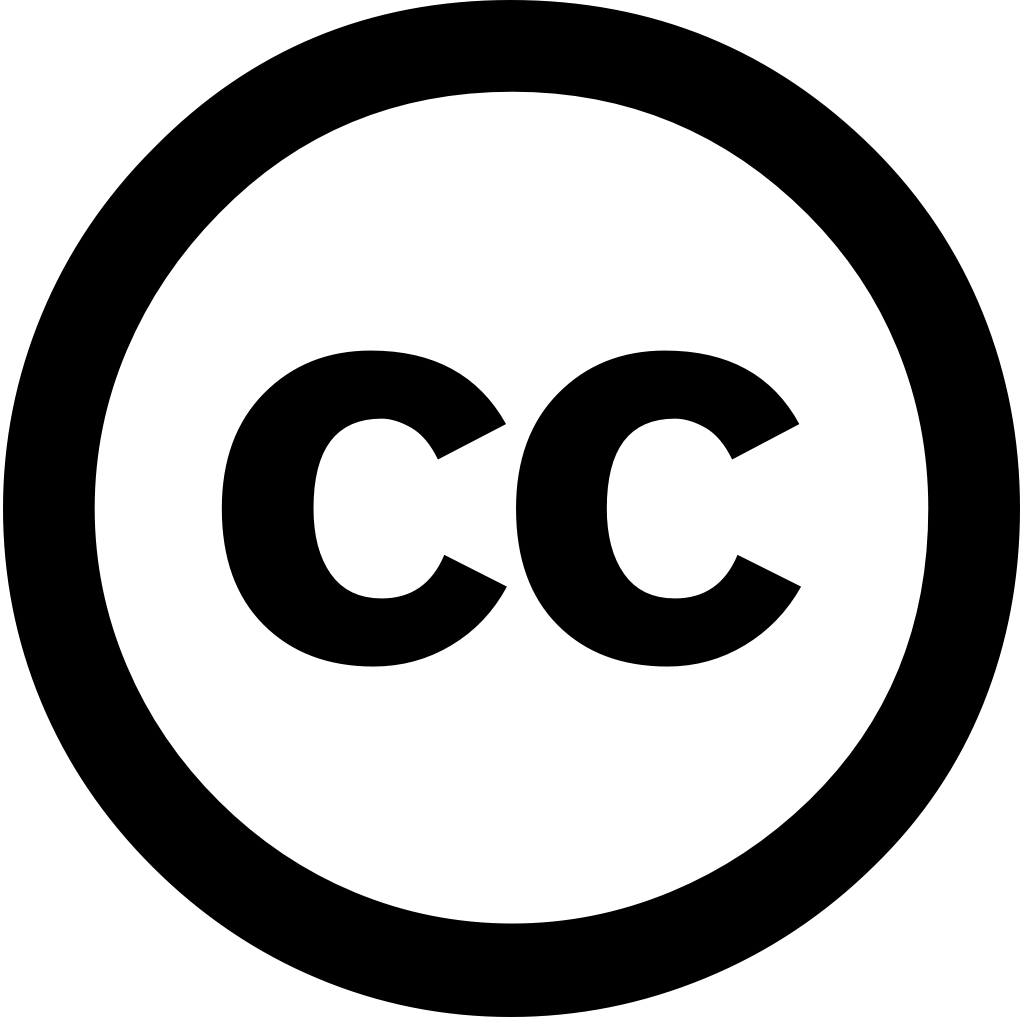
Scientific Reports, Journal Year: 2025, Volume and Issue: 15(1)
Published: May 7, 2025
Language: Английский
Scientific Reports, Journal Year: 2025, Volume and Issue: 15(1)
Published: May 7, 2025
Language: Английский
Future Internet, Journal Year: 2025, Volume and Issue: 17(1), P. 30 - 30
Published: Jan. 11, 2025
The proliferation of the Internet Things (IoT) has transformed digital landscape, enabling a vast array interconnected devices to communicate and share data seamlessly. However, rapid expansion IoT networks also introduced significant cybersecurity challenges. This paper presents comprehensive survey in ecosystem, examining current state research, identifying critical security vulnerabilities, exploring advanced strategies for mitigating threats. covers various facets security, including device authentication, integrity, privacy, network emerging role artificial intelligence (AI) bolstering defenses. By synthesizing existing research highlighting ongoing challenges, this aims provide holistic understanding guide future endeavors.
Language: Английский
Citations
4International Journal of Computational and Experimental Science and Engineering, Journal Year: 2025, Volume and Issue: 11(1)
Published: Feb. 28, 2025
Internet of Things (IoT) applications have made inroads into different domains, providing unique solutions—Internet technology offers seamless integration physical and digital worlds. However, the broad nature technologies protocols used in IoT has increased vulnerability from malicious attackers. Hence, protecting cyber-attacks is imperative. Researchers implemented intrusion detection systems to overcome this issue improve cybersecurity scenarios. With new threats cybercrime emerging, a continuous effort required enhance security applications. To address pressing need, we present our study that proposes deep learning-based framework bolster at use cases level by exploiting power transfer learning ensembling it models pre-trained larger datasets. Deep attain high performance with help hyperparameter tuning, achieve through PSO proposed system. Our ensemble system shows how individual can outperform using best-performing as constituents approach. We introduce an algorithm called — Optimized Ensemble Learning-Based Intrusion Detection (OEL-ID). This leverages corresponding optimization strategies boost for improved cyber Using UNSW-NB15 benchmark dataset, empirical demonstrates method, compared some existing models, obtained accuracy 98.89%, which, turn, provided highest comparative accuracy. Therefore, be allows significant system's underlying
Language: Английский
Citations
4IEEE Access, Journal Year: 2023, Volume and Issue: 11, P. 115047 - 115061
Published: Jan. 1, 2023
Ensuring the security of critical Industrial Internet Things (IIoT) systems is utmost importance, with a primary focus on identifying cyber-attacks using Intrusion Detection Systems (IDS). Deep learning (DL) techniques are frequently utilized in anomaly detection components IDSs. However, these models often generate high false-positive rates, and their decision-making rationale remains opaque, even to experts. Gaining insights into reasons behind an IDS's decision block specific packet can aid cybersecurity professionals assessing system's effectiveness creating more cyber-resilient solutions. In this paper, we offer explainable ensemble DL-based IDS improve transparency robustness IDSs IIoT networks. The framework incorporates Shapley additive explanations (SHAP) Local comprehensible-independent Clarifications (LIME) methods elucidate decisions made by IDSs, providing valuable experts responsible for maintaining network developing systems. ToN_IoT dataset was used evaluate efficacy suggested framework. As baseline intrusion system, extreme machines (ELM) model implemented compared other models. Experiments show results.
Language: Английский
Citations
27Expert Systems with Applications, Journal Year: 2024, Volume and Issue: 251, P. 123919 - 123919
Published: April 5, 2024
Language: Английский
Citations
9ACM Transactions on Privacy and Security, Journal Year: 2025, Volume and Issue: unknown
Published: March 19, 2025
Detecting unknown cyberattacks remains an open research problem and a significant challenge for the community security industry. This paper tackles detection of cybersecurity attacks in Internet Things (IoT) traditional networks by categorizing them into two types: entirely new classes (type-A) within already known (type-B). To address this, we propose novel multi-stage, multi-layer zero trust architecture intrusion system (IDS), uniquely designed to handle these attack types. The employs hybrid methodology that combines supervised one unsupervised learning stages funnel-like design, significantly advancing current capabilities. A key innovation is layered filtering mechanism, leveraging type-A type-B concepts systematically classify traffic as malicious unless proven otherwise. Using four benchmark datasets, proposed demonstrates improvements accuracy, recall, error classification rates attacks, achieving average accuracy recall ranging between 88% 95%. work offers robust, scalable framework enhancing diverse network environments.
Language: Английский
Citations
1Wiley Interdisciplinary Reviews Data Mining and Knowledge Discovery, Journal Year: 2025, Volume and Issue: 15(2)
Published: March 28, 2025
ABSTRACT As the Internet of Things (IoT) continues expanding its footprint across various sectors, robust security systems to mitigate associated risks are more critical than ever. Intrusion Detection Systems (IDS) fundamental in safeguarding IoT infrastructures against malicious activities. This systematic review aims guide future research by addressing six pivotal questions that underscore development advanced IDS tailored for environments. Specifically, concentrates on applying machine learning (ML) and deep (DL) technologies enhance capabilities. It explores feature selection methodologies aimed at developing lightweight solutions both effective efficient scenarios. Additionally, assesses different datasets balancing techniques, which crucial training models perform accurately reliably. Through a comprehensive analysis existing literature, this highlights significant trends, identifies current gaps, suggests studies optimize frameworks ever‐evolving landscape.
Language: Английский
Citations
1Engineering Applications of Artificial Intelligence, Journal Year: 2023, Volume and Issue: 126, P. 106771 - 106771
Published: July 21, 2023
Language: Английский
Citations
18Heliyon, Journal Year: 2024, Volume and Issue: 10(8), P. e29410 - e29410
Published: April 1, 2024
Currently, the Internet of Things (IoT) generates a huge amount traffic data in communication and information technology. The diversification integration IoT applications terminals make vulnerable to intrusion attacks. Therefore, it is necessary develop an efficient Intrusion Detection System (IDS) that guarantees reliability, integrity, security systems. detection considered challenging task because inappropriate features existing input slow training process. In order address these issues, effective meta heuristic based feature selection deep learning techniques are developed for enhancing IDS. Osprey Optimization Algorithm (OOA) proposed selecting highly informative from which leads differentiation among normal attack network. Moreover, traditional sigmoid tangent activation functions replaced with Exponential Linear Unit (ELU) function propose modified Bi-directional Long Short Term Memory (Bi-LSTM). Bi-LSTM used classifying types ELU makes gradients extremely large during back-propagation faster learning. This research analysed three different datasets such as N-BaIoT, Canadian Institute Cybersecurity Dataset 2017 (CICIDS-2017), ToN-IoT datasets. empirical investigation states framework obtains impressive accuracy 99.98 %, 99.97 % 99.88 on CICIDS-2017, datasets, respectively. Compared peer frameworks, this high better interpretability reduced processing time.
Language: Английский
Citations
7Computers & Security, Journal Year: 2024, Volume and Issue: 145, P. 104005 - 104005
Published: July 20, 2024
Language: Английский
Citations
7Decision Analytics Journal, Journal Year: 2023, Volume and Issue: 7, P. 100220 - 100220
Published: April 6, 2023
Artificial neural networks (ANNs) are commonly used to solve nonlinear problems. ANNs composed of parallel interconnected layers that include processing elements called neurons. These neurons use mathematical functions process the data, mimicking human brain. In this study, we for designing deep underground supports. We develop a predictive model ultimate axial capacity foundations drilled shafts, as support highways, bridges and high-rise buildings. Different single hidden layer ANN architectures utilized predict shafts. The data in study load tests collected from extended version Nevada Deep Foundation Load Test Database. This focuses on certain difficulties foundation engineering problems, such variability soil or construction method which, conventional methods might not fully address. can sometimes lead overestimation underestimation capacity. Consequently, project be unsafe expensive. Therefore, objective is improve prediction accuracy which important save design costs time. A total 45 were divided into 85% training, 15% validation. developed achieved good generalization with Root Mean-Squared Error 2807.08 Kilopounds force (kips), Mean Absolute 2380.6 kips, an R-squared (R2) 87% unseen data. results adapted future studies industry first-order estimate architecture nominal
Language: Английский
Citations
14