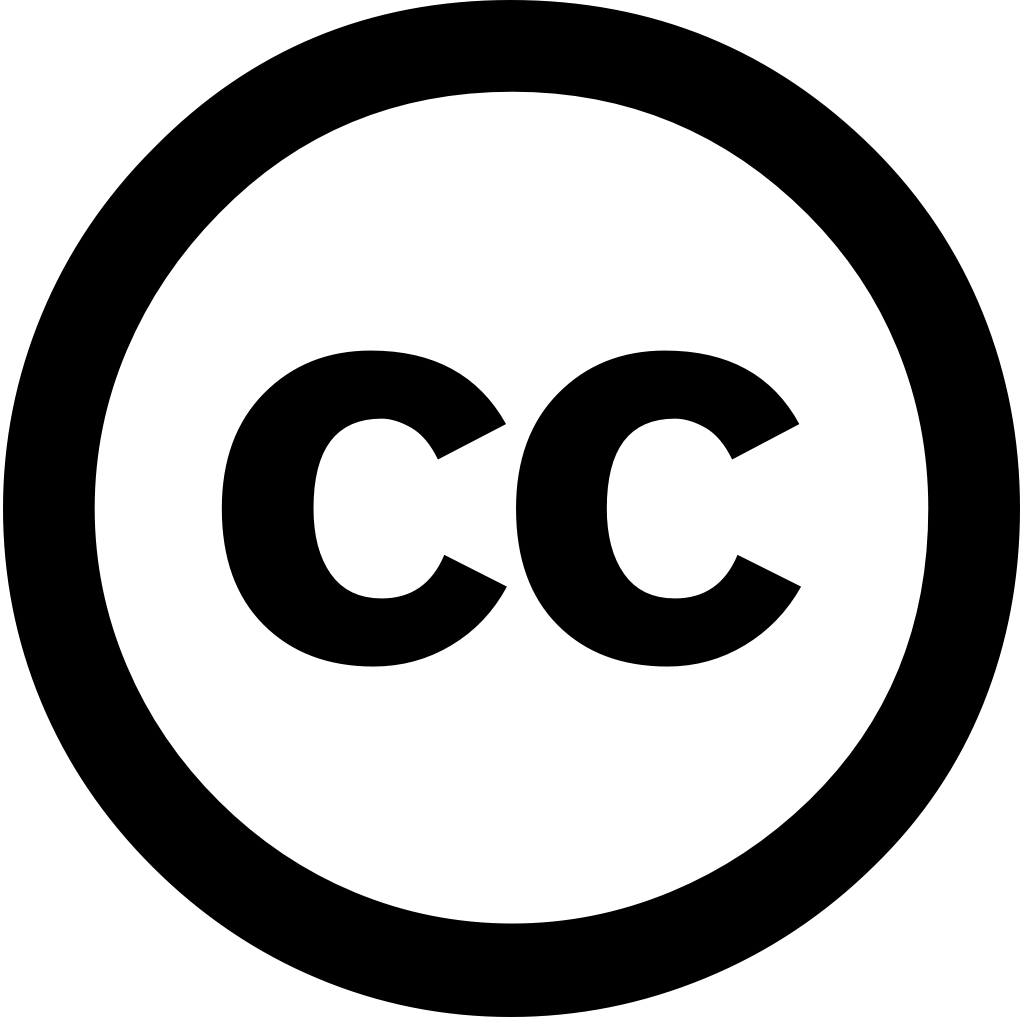
Atmosphere, Journal Year: 2024, Volume and Issue: 15(12), P. 1407 - 1407
Published: Nov. 22, 2024
Predicting streamflow is essential for managing water resources, especially in basins and watersheds where snowmelt plays a major role river discharge. This study evaluates the advanced deep learning models accurate monthly peak forecasting Gilgit River Basin. The utilized were LSTM, BiLSTM, GRU, CNN, their hybrid combinations (CNN-LSTM, CNN-BiLSTM, CNN-GRU, CNN-BiGRU). Our research measured model’s accuracy through root mean square error (RMSE), absolute (MAE), Nash–Sutcliffe efficiency (NSE), coefficient of determination (R2). findings indicated that models, CNN-BiGRU achieved much better performance than traditional like LSTM GRU. For instance, lowest RMSE (71.6 training 95.7 testing) highest R2 (0.962 0.929 testing). A novel aspect this was integration MODIS-derived snow-covered area (SCA) data, which enhanced model substantially. When SCA data included, CNN-BiLSTM improved from 83.6 to 71.6 during 108.6 testing. In prediction, outperformed other with (108.4), followed by (144.1). study’s results reinforce notion combining CNN’s spatial feature extraction capabilities temporal dependencies captured or GRU significantly enhances accuracy. demonstrated improvements prediction accuracy, extreme events, highlight potential these support more informed decision-making flood risk management allocation.
Language: Английский