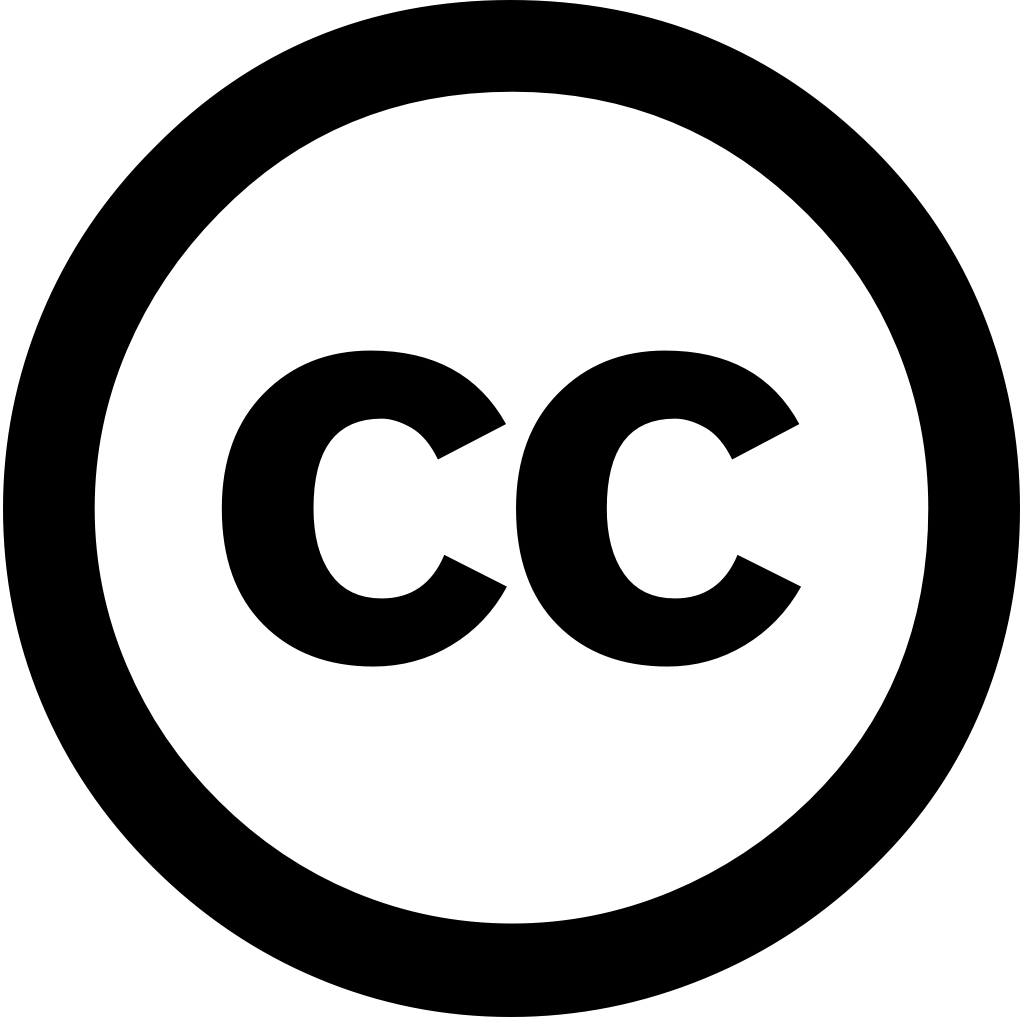
International Journal of Applied Earth Observation and Geoinformation, Journal Year: 2024, Volume and Issue: 133, P. 104113 - 104113
Published: Aug. 28, 2024
Language: Английский
International Journal of Applied Earth Observation and Geoinformation, Journal Year: 2024, Volume and Issue: 133, P. 104113 - 104113
Published: Aug. 28, 2024
Language: Английский
Journal of Human Earth and Future, Journal Year: 2024, Volume and Issue: 5(2), P. 216 - 242
Published: June 1, 2024
The management and monitoring of land use in geothermal fields are crucial for the sustainable utilization water resources, as well striking a balance between production renewable energy preservation environment. This study primarily compared Support Vector Machine (SVM) Random Forest (RF) machine learning methods, using satellite imagery from Landsat 8 Sentinel 2 2021 2023, to monitor Patuha area. objective is improve practices by accurately categorizing different cover types. comparative analysis assessed efficacy these techniques upholding sustainability regions. examined application SVM RF techniques, with particular emphasis on parameter refinement model assessment, enhance classification accuracy. By employing Kernlab e1071 algorithm comparison, research sought produce precise Land Use Model Map, which underscores significance advanced analytical environmental management. approach was utmost importance improving reinforcing practices. evaluation methods demonstrates superiority terms accuracy, stability, precision, particularly intricate urban settings, hence establishing it preferred tasks demanding high reliability. areas alignment Sustainable Development Goals (SDGs) 6 15, fosters conservation ecosystems. Doi: 10.28991/HEF-2024-05-02-06 Full Text: PDF
Language: Английский
Citations
15IEEE Transactions on Geoscience and Remote Sensing, Journal Year: 2024, Volume and Issue: 62, P. 1 - 13
Published: Jan. 1, 2024
Deep learning (DL) has been widely applied into hyperspectral image (HSI) classification owing to its promising feature and representation capabilities. However, limited by the spatial resolution of sensors, existing DL-based approaches mainly focus on pixel-level spectral information extraction through complex network architecture design, while ignoring existence mixed pixels in actual scenarios. To tackle this difficulty, we propose a novel dual-branch subpixel-guided for HSI classification, called DSNet, which automatically integrates subpixel convolutional class features introducing deep autoencoder unmixing enhance performance. DSNet is capable fully considering physically nonlinear properties within subpixels adaptively generating diagnostic abundances an unsupervised manner achieve more reliable decision boundaries label distributions. The fusion module designed ensure high-quality across pixel features, further promoting stable joint classification. Experimental results three benchmark datasets demonstrate effectiveness superiority compared with state-of-the-art approaches. codes will be available at https://github.com/hanzhu97702/DSNet, contributing remote sensing community.
Language: Английский
Citations
8International Journal of Remote Sensing, Journal Year: 2024, Volume and Issue: 45(14), P. 4883 - 4896
Published: July 5, 2024
The recent deployment of new satellite-based hyperspectral imaging sensors, along with advances in classification methods, has enhanced the acquisition information on spatiotemporal patterns Land Use/Land Cover (LULC).. With this context, study presented paper seeks to assess combined utilization Pixxel's technology demonstrator satellite mission Support Vector Machines (SVM) and Random Forest (RF) machine learning classifiers for LULC mapping an agricultural area located Queensland, Australia. Accuracy assessment resulting maps been done based relevant accuracy metrics. It observed that SVMs outperformed RF terms both overall urban vegetation cover accuracy. An 92.34% kappa 0.90 was reported SVMs' results, whereas RF, 89.65% 0.89 kappa, respectively. Both produced similar results classes mango tree sugarcane crops, minor differences were depiction water bodies artificial surfaces. Our findings provide insightful perspectives potential Pixxel datasets delineating distribution landscapes, showcasing sensors' promising field.
Language: Английский
Citations
5Environmental Modelling & Software, Journal Year: 2025, Volume and Issue: unknown, P. 106405 - 106405
Published: Feb. 1, 2025
Language: Английский
Citations
0Land, Journal Year: 2025, Volume and Issue: 14(3), P. 643 - 643
Published: March 18, 2025
The mapping of land use/cover (LULC) types is a crucial tool for natural resource management and monitoring changes in both human physical environments. Unmanned aerial vehicles (UAVs) provide high-resolution data, enhancing the capability accurate LULC representation at potentially very high spatial resolutions. In present study, two widely used supervised classification methods, namely Maximum Likelihood Classification (MLC) Mahalanobis Distance (MDC), were applied to analyze image data collected by UAVs from typical Mediterranean site located Greece. study area, characterized diverse uses (urban, agricultural, areas), served as an ideal field comparing methods. Although methods produced comparable results, MLC outperformed MDC, with overall accuracy 96.58% Kappa coefficient 0.942, compared MDC which 92.77% 0.878 reported. This highlights advantages using produce robust information on geospatial variability given area resolution cost-efficient, timely, on-demand manner. Such can help decision- policy-making ensuring more sustainable environment. study’s limitations, including small relatively homogeneous are acknowledged. Future research could focus exploring use advanced techniques, such deep learning landscapes, would assist present’s approach applicability.
Language: Английский
Citations
0Scientific Data, Journal Year: 2025, Volume and Issue: 12(1)
Published: March 27, 2025
Accurate land cover data was fundamental for formulating sound planning and sustainable development strategies. This study focused on the Tibetan Plateau (TP), a globally sensitive ecological area, developed locally tailored annual 30 m resolution dataset from 1990 to 2023 (TPLCD). Leveraging Google Earth Engine (GEE) platform Landsat processing, LandTrendr employed generate robust, high-precision training samples. Subsequently, random forest classification spatiotemporal smoothing strategies were applied precisely map dynamics of TP. Rigorous validation through visual interpretation, authoritative third-party datasets (Geo-Wiki GLCVSS), thematic cross-comparisons, revealed an overall accuracy 84.8%, Kappa coefficient 0.78, fully affirming dataset's high reliability. provided invaluable empirical evidence understanding vulnerability adaptability TP's ecosystem.
Language: Английский
Citations
0Gondwana Research, Journal Year: 2025, Volume and Issue: unknown
Published: April 1, 2025
Language: Английский
Citations
0Earth, Journal Year: 2024, Volume and Issue: 5(2), P. 244 - 254
Published: June 19, 2024
Land use/land cover (LULC) is a fundamental concept of the Earth’s system intimately connected to many phases human and physical environment. LULC mappings has been recently revolutionized by use high-resolution imagery from unmanned aerial vehicles (UAVs). The present study proposes an innovative approach for obtaining maps using consumer-grade UAV combined with two machine learning classification techniques, namely RF SVM. methodology presented herein tested at Mediterranean agricultural site located in Greece. emphasis placed on commercially available, low-cost RGB camera which typical consumer’s option available today almost worldwide. results evidenced capability SVM when data very high spatial resolution. Such information can be practical value both farmers decision-makers reaching most appropriate decisions this regard.
Language: Английский
Citations
3Published: April 10, 2024
Analysis of land use/land cover (LULC) in the catchment areas is first action toward safeguarding freshwater resources. The LULC information watershed has gained popularity natural science field as it helps water resource managers and environmental health specialists develop conservation strategies based on available quantitative information. Thus, remote sensing cornerstone addressing environmental-related issues at level. In this study, performance four machine learning algorithms (MLAs), such Random Forests (RF), Support Vector Machine (SVM), Artificial Neural Networks (ANN), Naïve Bayes (NB) was investigated to classify into nine relevant classes undulating landscape using Landsat 8 Operational Land Imager (L8-OLI) imagery. assessment MLAs were visual inspection analyst commonly used metrics, user’s accuracy (UA), producers’ (PA), overall (OA), kappa coefficient. produced good results, where RF (OA= 97.02%, Kappa= 0.96), SVM 89.74 %, 0.88), ANN 87%, 0.86), NB 68.64 0.58). results show outstanding model over with a small margin. While yielded satisfactory which could be primarily influenced by its sensitivity limited training samples. contrast, robust due an ability high-dimensional data data.
Language: Английский
Citations
2Remote Sensing, Journal Year: 2024, Volume and Issue: 16(12), P. 2219 - 2219
Published: June 19, 2024
Analysis of land use/land cover (LULC) in catchment areas is the first action toward safeguarding freshwater resources. LULC information watershed has gained popularity natural science field as it helps water resource managers and environmental health specialists develop conservation strategies based on available quantitative information. Thus, remote sensing cornerstone addressing environmental-related issues at level. In this study, performance four machine learning algorithms (MLAs), namely Random Forests (RFs), Support Vector Machines (SVMs), Artificial Neural Networks (ANNs), Naïve Bayes (NB), were investigated to classify into nine relevant classes undulating landscape using Landsat 8 Operational Land Imager (L8-OLI) imagery. The assessment MLAs was a visual inspection analyst commonly used metrics, such user’s accuracy (UA), producers’ (PA), overall (OA), kappa coefficient. produced good results, where RF (OA = 97.02%, Kappa 0.96), SVM 89.74%, 0.88), ANN 87%, 0.86), NB 68.64%, 0.58). results show outstanding model over with significant margin. While yielded satisfactory its sensitivity limited training samples could primarily influence these results. contrast, robust be due an ability high-dimensional data data.
Language: Английский
Citations
1