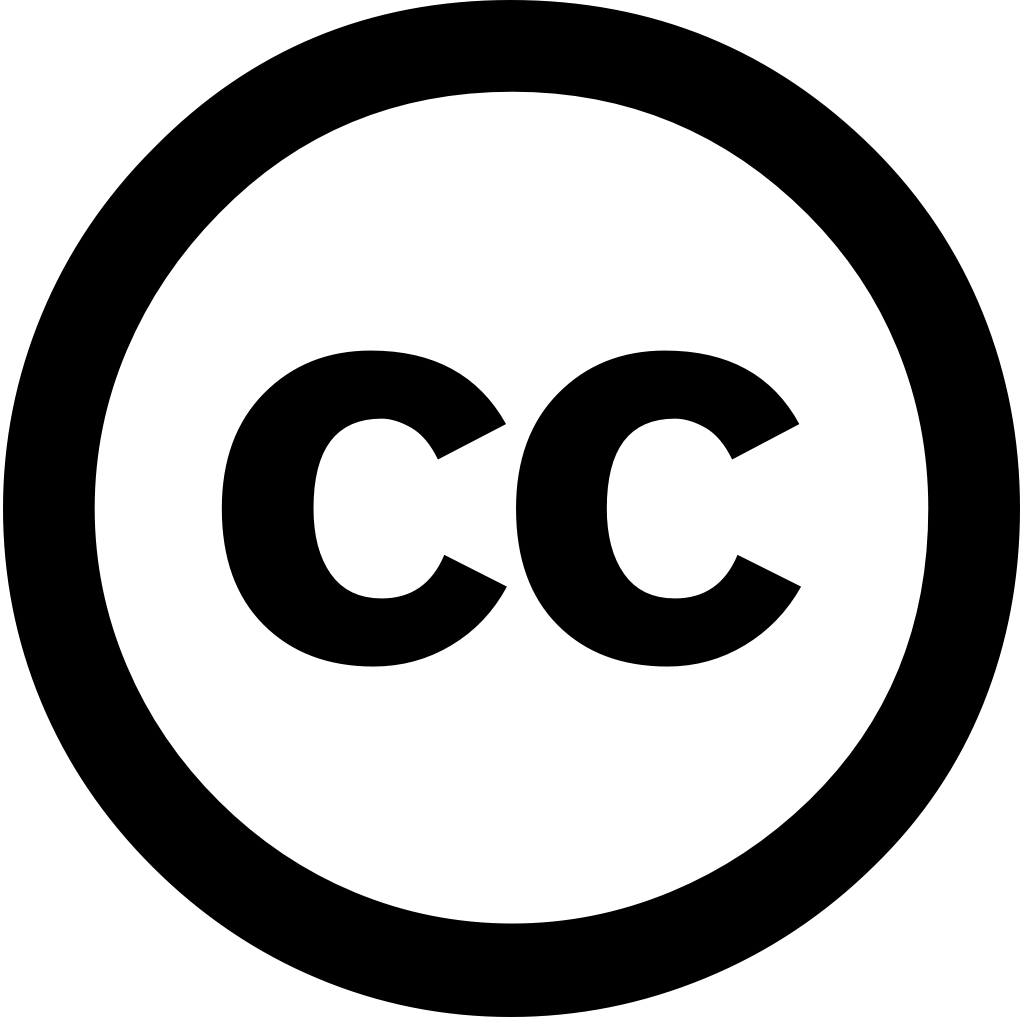
Remote Sensing Applications Society and Environment, Journal Year: 2025, Volume and Issue: unknown, P. 101473 - 101473
Published: Jan. 1, 2025
Language: Английский
Remote Sensing Applications Society and Environment, Journal Year: 2025, Volume and Issue: unknown, P. 101473 - 101473
Published: Jan. 1, 2025
Language: Английский
Remote Sensing, Journal Year: 2020, Volume and Issue: 12(24), P. 4184 - 4184
Published: Dec. 21, 2020
Climate variability and recurrent droughts have caused remarkable strain on water resources in most regions across the globe, with arid semi-arid areas being hardest hit. The impacts been notable surface resources, which are already under threat from massive abstractions due to increased demand, as well poor conservation unsustainable land management practices. Drought climate variability, their associated gained attention recent decades nations seek enhance mitigation adaptation mechanisms. Although use of satellite technologies has, late, prominence generating timely spatially explicit information drought different regions, they somewhat hampered by difficulties detecting evolution its complex nature, varying scales, magnitude occurrence, inherent data gaps. Currently, a number studies conducted monitor assess sub-Saharan Africa using remotely sensed in-situ datasets. This study therefore provides detailed overview progress made tracking remote sensing, including relevance monitoring hydrological Africa. paper further discusses traditional sensing methods drought, application key challenges, particular emphasis Additionally, characteristics limitations various sensors, indices, namely, Standardized Precipitation Index (SPI), Palmer Severity (PDSI), Normalized Difference Vegetation (NDVI), Condition (VCI), Water Requirement Satisfaction (WRSI), (NDWI), Modified (MNDWI), Land Surface (LSWI+5), (MNDWI+5), Automated Extraction (shadow) (AWEIsh), (non-shadow) (AWEInsh), discussed. scientific research strides knowledge gaps for investigations highlighted. While has advancing this review indicates need assessing especially context change demand. results suggests that Landsat-8 Sentinel-2 likely be best suited bodies, availability at relatively low cost, impressive spectral, spatial, temporal characteristics. effective indices SPI, PDSI, NDVI, VCI, NDWI, MNDWI, MNDWI+5, AWEIsh, AWEInsh. Overall, findings emphasize increasing role potential resources. However, there is future consider spatial integration techniques, radar data, precipitation, cloud computing, machine learning or artificial intelligence (AI) techniques improve understanding scales.
Language: Английский
Citations
154ISPRS Journal of Photogrammetry and Remote Sensing, Journal Year: 2021, Volume and Issue: 178, P. 282 - 296
Published: July 5, 2021
Language: Английский
Citations
125ISPRS Journal of Photogrammetry and Remote Sensing, Journal Year: 2021, Volume and Issue: 180, P. 163 - 173
Published: Aug. 26, 2021
Language: Английский
Citations
111Earth system science data, Journal Year: 2023, Volume and Issue: 15(1), P. 265 - 293
Published: Jan. 17, 2023
Abstract. Wetlands, often called the “kidneys of earth”, play an important role in maintaining ecological balance, conserving water resources, replenishing groundwater and controlling soil erosion. Wetland mapping is very challenging because its complicated temporal dynamics large spatial spectral heterogeneity. An accurate global 30 m wetland dataset that can simultaneously cover inland coastal zones lacking. This study proposes a novel method for by combining automatic sample extraction method, existing multi-sourced products, satellite time-series images stratified classification strategy. approach allowed generation first map with fine system (GWL_FCS30), including five sub-categories (permanent water, swamp, marsh, flooded flat saline) three tidal (mangrove, salt marsh flats), which was developed using Google Earth Engine platform. We combined expert knowledge, training refinement rules visual interpretation to generate geographically distributed samples. Second, we integrated Landsat reflectance products Sentinel-1 synthetic aperture radar (SAR) imagery various water-level phenological information capture heterogeneity wetlands. Third, applied strategy local adaptive random forest models produce at each 5∘×5∘geographical tile 2020. Lastly, GWL_FCS30, mosaicked 961 5∘×5∘ regional maps, validated 25 708 validation samples, achieved overall accuracy 86.44 % kappa coefficient 0.822. The cross-comparisons other demonstrated GWL_FCS30 performed better capturing patterns wetlands had significant advantages over diversity sub-categories. statistical analysis showed area reached 6.38 million km2, 6.03 km2 0.35 wetlands, approximately 72.96 were poleward 40∘ N. Therefore, conclude proposed suitable large-area product has potential provide vital support management. 2020 freely available https://doi.org/10.5281/zenodo.7340516 (Liu et al., 2022).
Language: Английский
Citations
85Remote Sensing of Environment, Journal Year: 2024, Volume and Issue: 311, P. 114316 - 114316
Published: July 18, 2024
Accurate and high-resolution land cover (LC) information is vital for addressing contemporary environmental challenges. With the advancement of satellite data acquisition, cloud-based processing, deep learning technology, Global Land Cover (GLC) map production has become increasingly feasible. a growing number available GLC maps, comprehensive evaluation comparison necessary to assess their accuracy suitability diverse uses. This particularly applies maps lacking statistically robust assessment or sufficient reported detail on validation procedures. study conducts comparative independent recent 10 m namely ESRI Use/Land (LULC), ESA WorldCover, Google World Resources Institute (WRI)'s Dynamic World, examining spatial representation thematic at global, continental, national (for 47 larger countries) levels. Since impacted by reference uncertainty owing geolocation labelling errors, five approaches dealing with were evaluated. Of considered approaches, using sample label supplemented majority within neighborhood found produce more reasonable estimates compared overly optimistic approach any pessimistic direct between labels. Overall global accuracies range 73.4% ± 0.7% (95% confidence interval) 83.8% 0.4% WorldCover having highest followed LULC. The quality varies across different LC classes, continents, countries. maps' was assessed various homogeneity levels 3 × kernel. Although as this reveals that LULC have less than WorldCover. All lower in heterogenous landscapes some countries such Mozambique, Tanzania, Nigeria, Spain. To select most suitable product, users should consider both map's over area interest appropriate application. For future mapping, producers are encouraged adopt standardized class definitions ensure comparability maps. Additionally, heterogeneous key features be improved versions Independent efforts regional levels, well changes, strengthened enhance utility these scales long-term monitoring.
Language: Английский
Citations
19Remote Sensing, Journal Year: 2020, Volume and Issue: 12(12), P. 2010 - 2010
Published: June 23, 2020
The diversity of change detection (CD) methods and the limitations in generalizing these techniques using different types remote sensing datasets over various study areas have been a challenge for CD applications. Additionally, most implemented two intensive time-consuming steps: (a) predicting areas, (b) decision on predicted areas. In this study, novel framework based convolutional neural network (CNN) is proposed to not only address aforementioned problems but also considerably improve level accuracy. CNN-based contains three parallel channels: first second channels, respectively, extract deep features original first- second-time imagery third channel focuses extraction differencing staking features. each includes convolution kernels: 1D-, 2D-, 3D-dilated-convolution. effectiveness reliability method are evaluated benchmark (i.e., multispectral, hyperspectral, Polarimetric Synthetic Aperture RADAR (PolSAR)). results maps both visually statistically by calculating nine accuracy indices. Moreover, compared those several state-of-the-art algorithms. All prove that outperforms other techniques. For instance, considering scenarios, Overall Accuracies (OAs) Kappa Coefficients (KCs) better than 95.89% 0.805, Miss Detection (MD) False Alarm (FA) rates lower 12% 3%, respectively.
Language: Английский
Citations
94Remote Sensing, Journal Year: 2021, Volume and Issue: 13(4), P. 700 - 700
Published: Feb. 14, 2021
Mapping crop types and land cover in smallholder farming systems sub-Saharan Africa remains a challenge due to data costs, high cloud cover, poor temporal resolution of satellite data. With improvement technology image processing techniques, there is potential for integrating from sensors with different spectral characteristics resolutions effectively map cover. In our Malawi study area, it common that are no cloud-free images available the entire growth season. The goal this experiment produce detailed type maps agricultural landscapes using Sentinel-1 (S-1) radar data, Sentinel-2 (S-2) optical S-2 PlanetScope fusion, S-1 C2 matrix H/α polarimetric decomposition. We evaluated ability combine these two locations. random forest algorithm, trained collected field, complemented samples digitized Google Earth Pro DigitalGlobe, was used classification experiments. results show fused + covariance (C2) decomposition (an entropy-based method) fusion outperformed all other combinations, producing higher overall accuracies (OAs) (>85%) Kappa coefficients (>0.80). These OAs represent 13.53% 11.7% on Sentinel-2-only (OAs < 80%) Thimalala Edundu, respectively. also provided accurate insights into distribution area. findings suggest cloud-dense resource-poor locations, fusing presents an opportunity operational mapping support food security environmental management decision-making.
Language: Английский
Citations
86Remote Sensing, Journal Year: 2020, Volume and Issue: 12(10), P. 1614 - 1614
Published: May 18, 2020
Saline wetlands experience large temporal fluctuations in water supply during the year and are recharged only or mainly through precipitation, meaning they vulnerable to climate-change-induced aridification. Most passive satellite sensors unsuitable for continuous wetland monitoring due cloud cover their relatively low resolution. However, active such as C-band synthetic aperture radar of Sentinel-1 satellites offer free, cloud-independent data. We examined surface changes from October 2014 November 2018 strictly protected area (13,000 ha) Upper-Kiskunság Alkaline Lakes region Danube–Tisza Interfluve Hungary, with aim helping nature protection planning. Changes sensitivity can be defined based on knowledge variability. developed a method detection automatic classification, applying so-called WEKA K-Means clustering algorithm. For data processing analysis, we used Google Earth Engine platform. In terms validation, compared our results multispectral Modified Normalized Difference Water Index (MNDWI) derived Landsat 8 Sentinel-2 top-of-atmosphere reflectance images using threshold-based binary classifier (receiver operator characteristics) MNDWI Using two completely distinct methods operating wavelength ranges, obtained adequately matching results, Spearman’s correlation coefficients (ρ) ranging 0.54 0.80.
Language: Английский
Citations
74International Journal of Applied Earth Observation and Geoinformation, Journal Year: 2021, Volume and Issue: 102, P. 102453 - 102453
Published: July 26, 2021
Wetland vegetation is susceptible to climate change and human disturbance, has experienced significant losses degradation. However, the spatial patterns of dynamics for China's inland lake wetlands remain unknown. In this paper, an adaptive-stacking algorithm based on Google Earth Engine was proposed map distribution Dongting Lake wetland using Sentinel-1/2 DEM data. Subsequently, LandTrendr utilized analyze over 1999–2018 Landsat normalized combustion ratio time-series. By overlaying latest types dynamics, main were examined. Results showed that overall accuracy kappa coefficient classification 94.59% 0.92, respectively, which higher than those support vector machine random forest. The detection three (vegetation gain, loss, no changes) 83.67%. past 20 years, 2,604.43 5,458.84 km2 land loss respectively. increase in areas forest, reed, sedge 1330.39, 86.42, 136.97 km2, We found recovery condition around good, demonstrated key role played by national ecological protection policy.
Language: Английский
Citations
74IEEE Transactions on Geoscience and Remote Sensing, Journal Year: 2021, Volume and Issue: 60, P. 1 - 14
Published: Oct. 15, 2021
While
deep
learning
models
have
been
extensively
applied
to
land-use
land-cover
(LULC)
problems,
it
is
still
a
relatively
new
and
emerging
topic
for
separating
classifying
wetland
types.
On
the
other
hand,
ensemble
has
demonstrated
promising
results
in
improving
boosting
classification
accuracy.
Accordingly,
this
study
aims
develop
system
mapping
complex
areas
by
incorporating
satellite
datasets.
To
end,
time
series
of
Sentinel-1
dual-polarized
Synthetic
Aperture
Radar
(SAR)
dataset,
alongside
Sentinel-2
multispectral
imagery
(MSI),
are
used
as
input
data
model.
In
order
increase
diversity
extracted
features,
proposed
model,
herein
called
WetNet,
consists
three
different
submodels,
comprising
several
recurrent
convolutional
layers.
Furthermore,
multiple
ensembling
sections
added
stages
model
transferability
(to
areas)
reliability
final
results.
WetNet
evaluated
area
located
Newfoundland,
Canada.
Experimental
indicate
that
outperforms
state-of-the-art
(e.g.,
InceptionResnetV2,
InceptionV3,
DenseNet121)
terms
both
accuracy
processing
time.
This
makes
an
efficient
large-scale
application.
The
python
code
available
at
following
link
sake
reproducibility:
Language: Английский
Citations
67