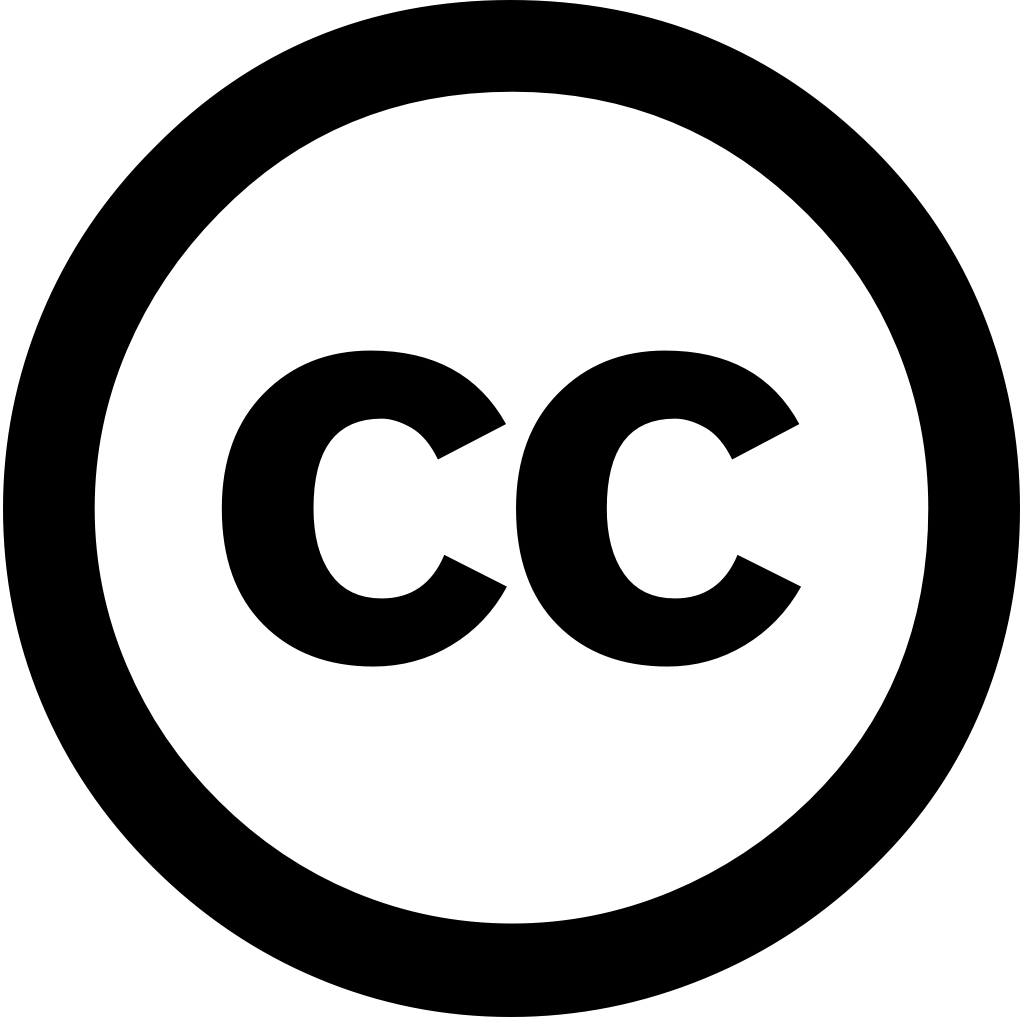
International Journal of Applied Earth Observation and Geoinformation, Journal Year: 2024, Volume and Issue: 136, P. 104315 - 104315
Published: Dec. 12, 2024
Language: Английский
International Journal of Applied Earth Observation and Geoinformation, Journal Year: 2024, Volume and Issue: 136, P. 104315 - 104315
Published: Dec. 12, 2024
Language: Английский
Health & Place, Journal Year: 2025, Volume and Issue: 91, P. 103411 - 103411
Published: Jan. 1, 2025
Language: Английский
Citations
1Cartography and Geographic Information Science, Journal Year: 2025, Volume and Issue: unknown, P. 1 - 13
Published: Feb. 24, 2025
Language: Английский
Citations
0Systems, Journal Year: 2025, Volume and Issue: 13(3), P. 187 - 187
Published: March 7, 2025
Within globalization, the significance of urban innovation cooperation has become increasingly evident. However, faces challenges due to various factors—social, economic, and spatial—making it difficult for traditional methods uncover intricate nonlinear relationships among them. Consequently, this research concentrates on cities within Yangtze River Delta region, employing an explainable machine learning model that integrates eXtreme Gradient Boosting (XGBoost), SHapley Additive exPlanations (SHAP), Partial Dependence Plots (PDPs) investigate interactive effects multidimensional factors impacting cooperation. The findings indicate XGBoost outperforms LR, SVR, RF, GBDT in terms accuracy effectiveness. Key results are summarized as follows: (1) Urban exhibits different phased characteristics. (2) There exist between factors, them, Scientific Technological dimension contributes most (30.59%) significant positive promoting effect later stage after surpassing a certain threshold. In Social Economic (23.61%), number Internet Users (IU) individually. Physical Space (20.46%) generally mutation points during early stages development, with overall predominantly characterized by trends. (3) Through application PDP, is further determined IU synergistic per capita Foreign Direct Investment (FDI), public library collections (LC), city night light data (NPP), while exhibiting negative antagonistic Average Annual Wage Staff (AAS) Enterprises above Designated Size Industry (EDS). (4) For at developmental stages, tailored development proposals should be formulated based single-factor contribution multifactor interaction effects. These insights enhance our understanding elucidate influencing factors.
Language: Английский
Citations
0Applied Geography, Journal Year: 2024, Volume and Issue: 171, P. 103373 - 103373
Published: Aug. 10, 2024
Language: Английский
Citations
3Heritage Science, Journal Year: 2024, Volume and Issue: 12(1)
Published: Nov. 27, 2024
Abstract As a vital carrier of traditional culture, Intangible Cultural Heritage (ICH) not only preserves historical value but also fosters cultural identity and confidence. This study utilizes explainable machine learning coupled coordination models to analyze the spatial distribution formation mechanisms ICH resources in Jiangsu-Zhejiang-Shanghai (Jiang-Zhe-Hu). The results indicate that (1) Jiang-Zhe-Hu exhibit clustered pattern characterized by “three primary cores two secondary cores.” core areas are Shanghai, Hangzhou, Suzhou, while Yangzhou Nanjing. (2) Population, number religious places, GDP have significant positive impact on Jiang-Zhe-Hu. NDVI road mileage relatively minor effects distribution. (3) In terms resources, Zhejiang Province overall has higher level than Jiangsu Province, with Lishui having highest evaluation most abundant resources. Regarding tourism industry development, Shanghai comprehensive value, followed Nanjing, Wuxi, Changzhou, all which high levels development. (4) According model analysis, demonstrates best coupling degree between industry, achieving good level. integration is better southern (e.g., Suzhou), there still imbalances development northern. an compared Jiangsu, more balanced However, room for improvement deep industry.
Language: Английский
Citations
3International Journal of Applied Earth Observation and Geoinformation, Journal Year: 2024, Volume and Issue: 136, P. 104315 - 104315
Published: Dec. 12, 2024
Language: Английский
Citations
3