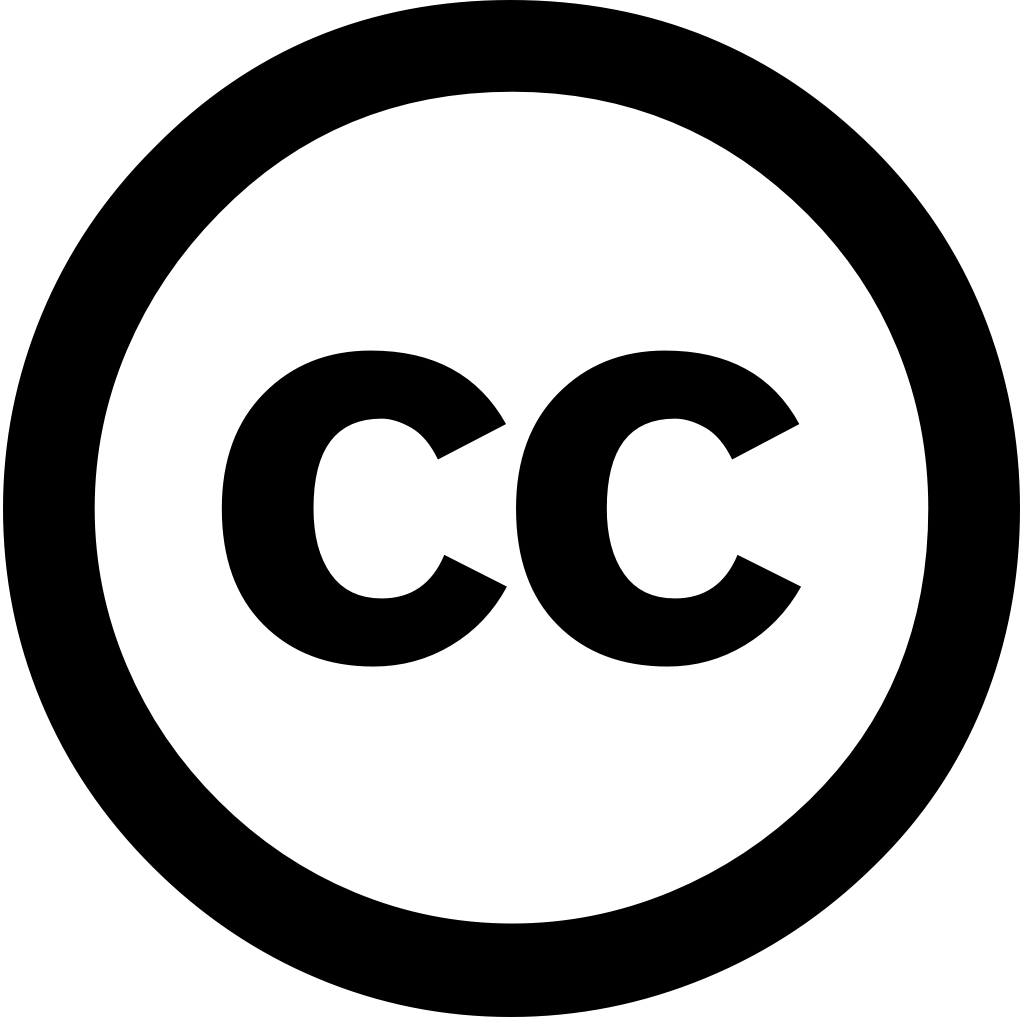
The Science of The Total Environment, Journal Year: 2025, Volume and Issue: 963, P. 178303 - 178303
Published: Jan. 20, 2025
Language: Английский
The Science of The Total Environment, Journal Year: 2025, Volume and Issue: 963, P. 178303 - 178303
Published: Jan. 20, 2025
Language: Английский
Natural Hazards Research, Journal Year: 2023, Volume and Issue: 3(3), P. 420 - 436
Published: May 19, 2023
The unique characteristics of drainage conditions in the Pagla river basin cause flooding and harm socioeconomic environment. main purpose this study is to investigate comparative utility six machine learning algorithms improve flood susceptibility ensemble techniques' capability elucidate underlying patterns floods make a more accurate prediction susceptibilities basin. In present scenario, frequency area becomes high with heavy sudden rainfall, so it essential mitigation measure. At First, spatial database was built 200 locations sixteen influencing factors, its process help Geographic Information System (GIS) environment build up different models applying techniques. It has found zone using learning-based Artificial Neural Network (ANN), Support Vector Machine (SVM), Random Forest (RF), Reduced Error Pruning Tree (REPTree), Logistic Regression (LR), Bagging helping GIS model validation Receiver Operating Characteristic Curve (ROC). Afterward, all gate accuracy zone. calculated under very 8.69%, 14.92%, 14.17%, 12.98%, 14.65%, 13.24% 13.41% for ANN, SVM, RF, REPTree, LR Bagging, respectively. Finally, ROC curve, Standard (SE), Confidence Interval (CI) at 95 per cent were used assess compare performance models. obtained results indicate that are highly accepted Area Under (AUC) between 0.889 (LR) 0.926 (Ensemble). After application, ROC, Ensemble suited highest compared other projecting area. curve AUC values 0.918 0.926, SE (0.023, 034), narrowest CI (95 cent) (0.873–0.962, 0.859–0.993) whereas (the ROC) value (0.914, 0.919), both training datasets. ensembling, result shows susceptible located lower part area, lie 4.46 6.00 result. areas comprise low height belong Murarai I, II, Suti I II C.D. block West Bengal. current will policymakers researcher determine conditioning problems prospects.
Language: Английский
Citations
46Geoscience Frontiers, Journal Year: 2024, Volume and Issue: 15(6), P. 101890 - 101890
Published: July 9, 2024
Landslide susceptibility assessment is crucial in predicting landslide occurrence and potential risks. However, traditional methods usually emphasize on larger regions of landsliding rely relatively static environmental conditions, which exposes the hysteresis refined-scale temporal dynamic changes. This study presents an improved approach by integrating machine learning models based random forest (RF), logical regression (LR), gradient boosting decision tree (GBDT) with interferometric synthetic aperture radar (InSAR) technology comparing them to their respective original models. The results demonstrated that combined improves prediction accuracy reduces false negative positive errors. LR-InSAR model showed best performance at both regional smaller scale, particularly when identifying areas high very susceptibility. Modeling were verified using data from field investigations including unmanned aerial vehicle (UAV) flights. great significance accurately assess help reduce prevent risk.
Language: Английский
Citations
44Environmental Monitoring and Assessment, Journal Year: 2024, Volume and Issue: 196(2)
Published: Jan. 4, 2024
Language: Английский
Citations
20Geoscience Frontiers, Journal Year: 2024, Volume and Issue: 15(3), P. 101780 - 101780
Published: Jan. 9, 2024
Flash floods (FFs) are amongst the most devastating hazards in arid regions response to climate change and can cause loss of agricultural land, human lives infrastructure. One major challenges is high-intensity rainfall events affecting low-lying areas that vulnerable FF. Several works this field have been conducted using ensemble machine learning models geohydrological models. However, current advancement eXtreme deep learning, which named factorisation (xDeepFM), for FF susceptibility mapping (FSM) lacking literature. The study introduces a new model employs previously unapplied approach enhance FSM capturing severity floods. proposed has three main objectives: (i) During- after-flood effects assessed through flood detection techniques Sentinel-1 data. (ii) Flood inventory updated remote sensing-based methods. derived implemented next step. (iii) An map generated an xDeepFM model. Therefore, aims apply estimate susceptible 13 factors emirates Fujairah, UAE. performance metrics show recall 0.9488), F1-score 0.9107), precision (0.8756) overall accuracy 90.41%. applied compared with traditional models, specifically neural network (78%), support vector (85.4%) random forest (88.75%). Random achieves high accuracy, due its strong depends on contribution, dataset size quality, available computational resources. Comparatively, efficiently complicated prediction problems having non-collinearity huge datasets. obtained denotes narrow basins, lowland coastal riverbank up 5 km (Fujairah) highly prone FF, whilst alluvial plains Al Dhaid hilly Fujairah low probability. city bounded by high-rise steep hills Gulf Oman, elevate water levels during heavy rainfall. Four synchronised influencing factors, namely, rainfall, elevation, drainage density, distance from geomorphology, account nearly 50% total contributing very susceptibility. This offers platform planners decision makers take timely actions potential mitigating
Language: Английский
Citations
17Ecological Indicators, Journal Year: 2023, Volume and Issue: 147, P. 109968 - 109968
Published: Feb. 6, 2023
Landslide susceptibility mapping is a meaningful method to avoid and reduce the loss from landslide hazard. The main goal of current paper propose hybrid model explore effect combining Best-first decision tree (BFT) with Bagging, Cascade generalization, Decorate, MultiboostAB, Random SubSpace measure achievement each combination model. Firstly, inventory map was produced using 364 landslides in Yongxin County China, then non-landslide data were generated based on buffer method. Secondly, 255 non-landslides randomly chosen for training rest 109 validation data. Then, fifteen environment factors chosen. Thirdly, Support vector machines (SVM) applied analysis most useful modeling. result demonstrated that all Several statistical indexes used performance, results revealed five models performed better than single BFT BFT-D BFT-B best effective can be adapted susceptibility. maps by will help land use arrangement groundwork expansion County.
Language: Английский
Citations
38Journal of Hydrology, Journal Year: 2023, Volume and Issue: 624, P. 129961 - 129961
Published: July 19, 2023
Language: Английский
Citations
28Applied Soft Computing, Journal Year: 2023, Volume and Issue: 148, P. 110846 - 110846
Published: Sept. 13, 2023
Language: Английский
Citations
25IEEE Access, Journal Year: 2023, Volume and Issue: 11, P. 107194 - 107217
Published: Jan. 1, 2023
Ensemble multifeatured deep learning methodology has emerged as a powerful approach to overcome the limitations of single models in terms generalization, robustness, and performance. This survey provides an extended review ensemble models, their applications, challenges, future directions. We explore potential applications these across various domains, including computer vision, medical imaging, natural language processing, speech recognition. By combining strengths multiple features, have demonstrated improved performance adaptability diverse problem settings. also discuss challenges associated with such model interpretability, computational complexity, selection, adversarial personalized federated learning. highlights recent advancements addressing emphasizes importance continued research tackling issues enable widespread adoption models. It outlook on directions, focusing development new algorithms, frameworks, hardware architectures that can efficiently handle large-scale computations required by Moreover, it underlines need for better understanding trade-offs between accuracy, resources optimize design deployment
Language: Английский
Citations
24Applied Water Science, Journal Year: 2024, Volume and Issue: 14(4)
Published: March 14, 2024
Abstract Among the various natural disasters that take place around world, flood is considered to be most extensive. There have been several floods in Buzău river basin, and as a result of this, area has chosen study area. For purpose this research, we applied deep learning machine benchmarks order prepare potential maps at basin scale. In regard 12 predictors, 205 non-flood locations were used input data into following 3 complex models: Deep Learning Neural Network-Harris Hawk Optimization-Index Entropy (DLNN-HHO-IOE), Multilayer Perceptron-Harris (MLP-HHO-IOE) Stacking ensemble-Harris (Stacking-HHO-IOE). The sample was divided training (70%) validating (30%) sample, meanwhile prediction ability conditioning factors tested through Correlation-based Feature Selection method. ROC Curve statistical metrics involved results validation. modeling process stated algorithms showed important predictors are represented by: slope (importance ≈ 20%), distance from 17.5%), land use 12%) TPI 10%). importance values compute susceptibility, while Natural Breaks method classify results. high very susceptibility spread on approximately 35–40% zone. Curve, terms Success, Rate shows highest performance achieved FPI DLNN-HHO-IOE (AUC = 0.97), followed by Stacking-HHO-IOE 0.966) MLP-HHO-IOE 0.953), Prediction indicates being performant model with an AUC 0.977, 0.97) 0.924).
Language: Английский
Citations
13Journal of Hydrologic Engineering, Journal Year: 2024, Volume and Issue: 29(6)
Published: Sept. 14, 2024
Language: Английский
Citations
11