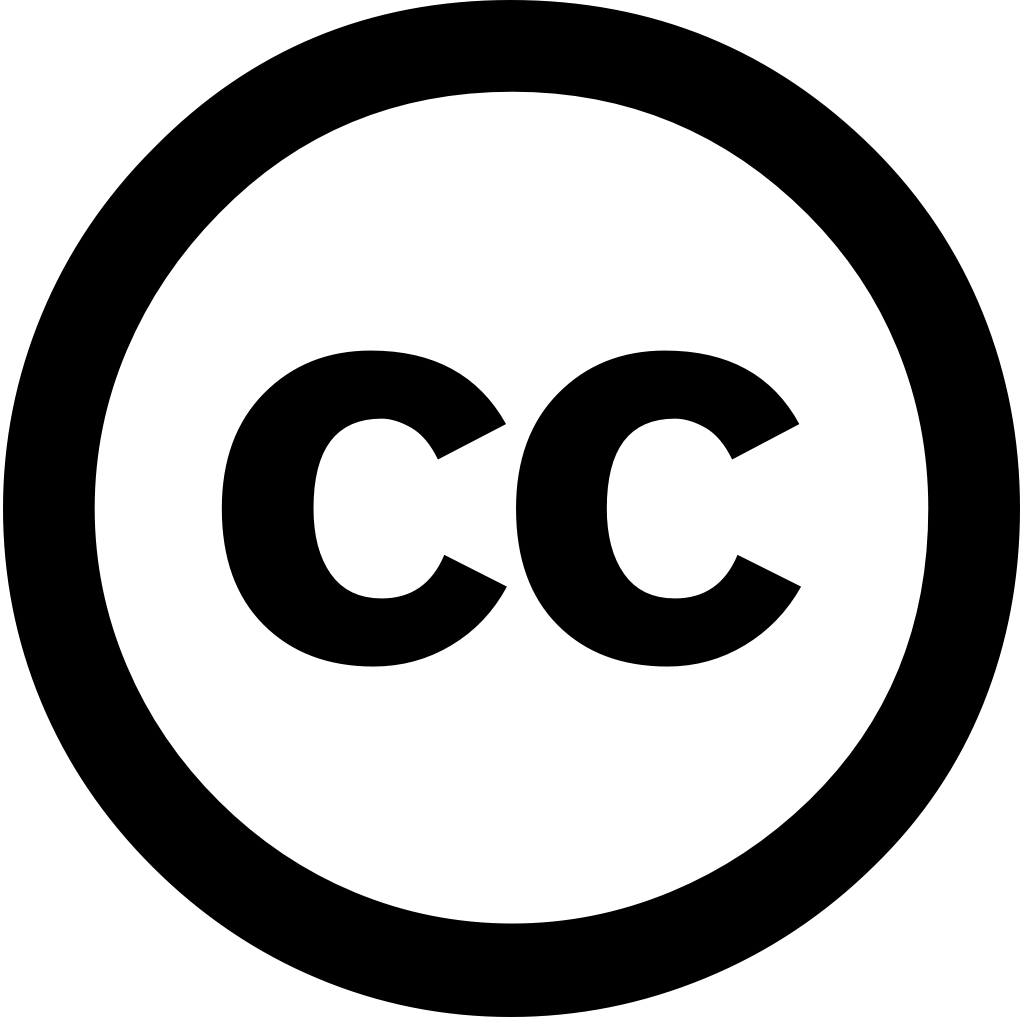
Engineering Science and Technology an International Journal, Journal Year: 2024, Volume and Issue: 60, P. 101907 - 101907
Published: Nov. 20, 2024
Language: Английский
Engineering Science and Technology an International Journal, Journal Year: 2024, Volume and Issue: 60, P. 101907 - 101907
Published: Nov. 20, 2024
Language: Английский
Journal of Energy Storage, Journal Year: 2024, Volume and Issue: 97, P. 112769 - 112769
Published: July 1, 2024
Language: Английский
Citations
12Energy Reports, Journal Year: 2024, Volume and Issue: 12, P. 2786 - 2800
Published: Sept. 2, 2024
Language: Английский
Citations
9Energy Conversion and Management X, Journal Year: 2025, Volume and Issue: unknown, P. 100980 - 100980
Published: March 1, 2025
Language: Английский
Citations
0Advances in computational intelligence and robotics book series, Journal Year: 2025, Volume and Issue: unknown, P. 69 - 80
Published: Feb. 28, 2025
This study evaluates the effectiveness of various machine learning strategies in managing energy Fuel Cell Electric Vehicles (FCEVs), focusing on fuel cell and battery inverter behaviour. The analysis compares four methods Gaussian Naive Bayes (NB), Random Forest, k-NN, AdaBoost using key metrics: Recall, f1-score, precision. NB Forest achieve identical performance for (Recall: 0.87, f1-score: 0.82, precision: 0.89) 0.66, 0.57, 0.5). In contrast, k-NN achieves a precision 0.74, while excels with 0.98 0.94. also outperforms other f1-score (0.98 cell, 0.90 battery) recall (0.95 0.84 battery), highlighting its superior behaviour control.
Language: Английский
Citations
0International Journal of Electrochemical Science, Journal Year: 2025, Volume and Issue: unknown, P. 101030 - 101030
Published: April 1, 2025
Language: Английский
Citations
0Sustainable Cities and Society, Journal Year: 2025, Volume and Issue: unknown, P. 106440 - 106440
Published: May 1, 2025
Language: Английский
Citations
0Scientific Reports, Journal Year: 2025, Volume and Issue: 15(1)
Published: May 9, 2025
Language: Английский
Citations
0Engineering Science and Technology an International Journal, Journal Year: 2024, Volume and Issue: 60, P. 101907 - 101907
Published: Nov. 20, 2024
Language: Английский
Citations
2