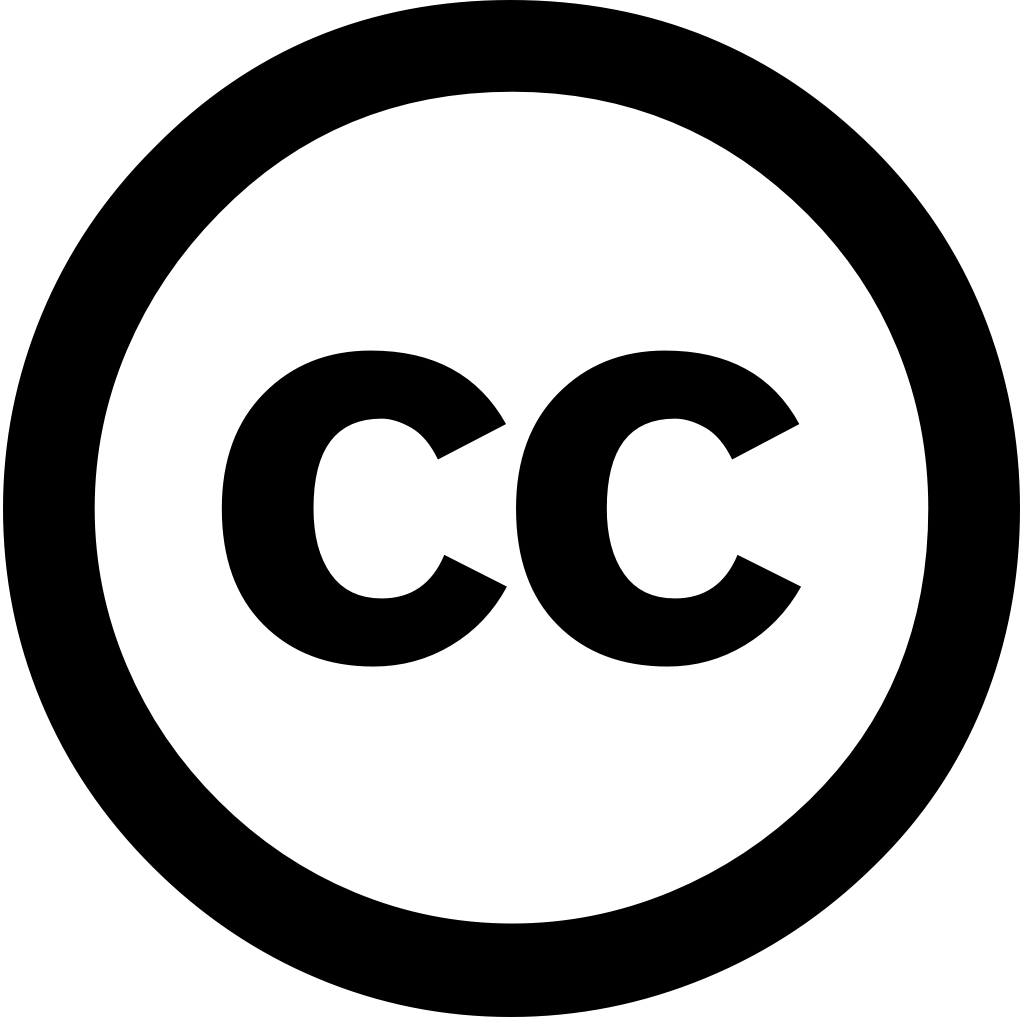
Geoenvironmental Disasters, Journal Year: 2024, Volume and Issue: 11(1)
Published: Sept. 5, 2024
Language: Английский
Geoenvironmental Disasters, Journal Year: 2024, Volume and Issue: 11(1)
Published: Sept. 5, 2024
Language: Английский
Scientific Reports, Journal Year: 2024, Volume and Issue: 14(1)
Published: June 25, 2024
Abstract This research suggests a robust integration of artificial neural networks (ANN) for predicting swell pressure and the unconfined compression strength expansive soils ( P s UCS -ES). Four novel ANN-based models, namely ANN-PSO (i.e., particle swarm optimization), ANN-GWO grey wolf ANN-SMA slime mould algorithm) alongside ANN-MPA marine predators’ were deployed to assess -ES. The models trained using nine most influential parameters affecting -ES, collected from broader range 145 published papers. observed results compared with predictions made by metaheuristics models. efficacy all these formulated was evaluated utilizing mean absolute error (MAE), Nash–Sutcliffe (NS) efficiency, performance index ρ , regression coefficient R 2 ), root square (RMSE), ratio RMSE standard deviation actual observations (RSR), variance account (VAF), Willmott’s agreement (WI), weighted percentage (WMAPE). All developed -ES had an significantly > 0.8 overall dataset. However, excelled in yielding high values training dataset TrD testing TsD validation VdD ). model also exhibited lowest MAE 5.63%, 5.68%, 5.48% respectively. model’s revealed that exceeded 0.9 . decreased Also, yielded higher (0.89, 0.93, 0.94) comparatively low (5.11%, 5.67, 3.61%) case PSO, GWO, SMA, witnessed overfitting problem because aforementioned 0.62, 0.56, 0.58 On contrary, no significant observation recorded ANN-base tested a-20 index. For maximum points lie within ± 20% error. sensitivity as well monotonicity analyses depicted trending corroborate existing literature. Therefore, it can be inferred recently built swarm-based ANN particularly ANN-MPA, solve complexities tuning hyperparameters ANN-predicted replicated practical scenarios geoenvironmental engineering.
Language: Английский
Citations
30Bulletin of Engineering Geology and the Environment, Journal Year: 2025, Volume and Issue: 84(2)
Published: Jan. 28, 2025
Language: Английский
Citations
1Transportation Infrastructure Geotechnology, Journal Year: 2025, Volume and Issue: 12(2)
Published: Feb. 1, 2025
Language: Английский
Citations
1Land, Journal Year: 2025, Volume and Issue: 14(3), P. 536 - 536
Published: March 4, 2025
Coastal areas are highly dynamic regions where surface deformation due to natural and anthropogenic activities poses significant challenges. Synthetic Aperture Radar (SAR) interferometry techniques, such as Persistent Scatterer Interferometry (PSInSAR), provide advanced capabilities monitor with high precision. This study applies PSInSAR techniques estimate along coastal zones from 2017 2020 using Sentinel-1 data. In the densely populated of Pasni, an annual subsidence rate 130 mm is observed, while northern, less region experiences uplift 70 per year. Seawater intrusion emerging issue causing in Pasni’s areas. It infiltrates freshwater aquifers, primarily excessive groundwater extraction rising sea levels. Over time, seawater destabilizes underlying soil rock structures, leading or gradual sinking ground surface. form risks infrastructure, agriculture, local ecosystem. Land varies area’s coastline. The eastern region, which reclaimed, particularly affected by erosion. results derived SAR data indicate major urban districts. information crucial for management, hazard assessment, planning sustainable development region.
Language: Английский
Citations
1Transportation Infrastructure Geotechnology, Journal Year: 2024, Volume and Issue: 11(6), P. 3903 - 3940
Published: Aug. 5, 2024
Language: Английский
Citations
8Journal of Central South University, Journal Year: 2024, Volume and Issue: unknown
Published: Aug. 5, 2024
Language: Английский
Citations
7Journal of Rock Mechanics and Geotechnical Engineering, Journal Year: 2024, Volume and Issue: unknown
Published: Sept. 1, 2024
Language: Английский
Citations
7Natural Hazards, Journal Year: 2024, Volume and Issue: unknown
Published: Sept. 19, 2024
Language: Английский
Citations
6Bulletin of Engineering Geology and the Environment, Journal Year: 2024, Volume and Issue: 83(11)
Published: Oct. 25, 2024
Language: Английский
Citations
6Geohazard Mechanics, Journal Year: 2024, Volume and Issue: unknown
Published: June 1, 2024
Effective control of blasting outcomes depends on a thorough understanding rock geology and the integration geological characteristics with blast design parameters. This study underscores importance adapting parameters to conditions optimize utilization explosive energy for fragmentation. To achieve this, data fifty geo-blast were collected used train machine learning algorithms. The objective was develop predictive models estimating oversize percentage, incorporating seven controlled components one uncontrollable index. employed combination hybrid long-short-term memory (LSTM), support vector regression, random forest Among these, LSTM model enhanced tree seed algorithm (LSTM-TSA) demonstrated highest prediction accuracy when handling large datasets. LSTM-TSA soft computing specifically leveraged various such as burden, spacing, stemming length, drill hole charge powder factor, joint set number. estimated percentage values these determined 0.7 m, 0.9 0.65 1.4 1.03 kg/m3, 35%, 2, respectively. Application resulted in significant 28.1% increase crusher's production rate, showcasing its effectiveness improving operations.
Language: Английский
Citations
5