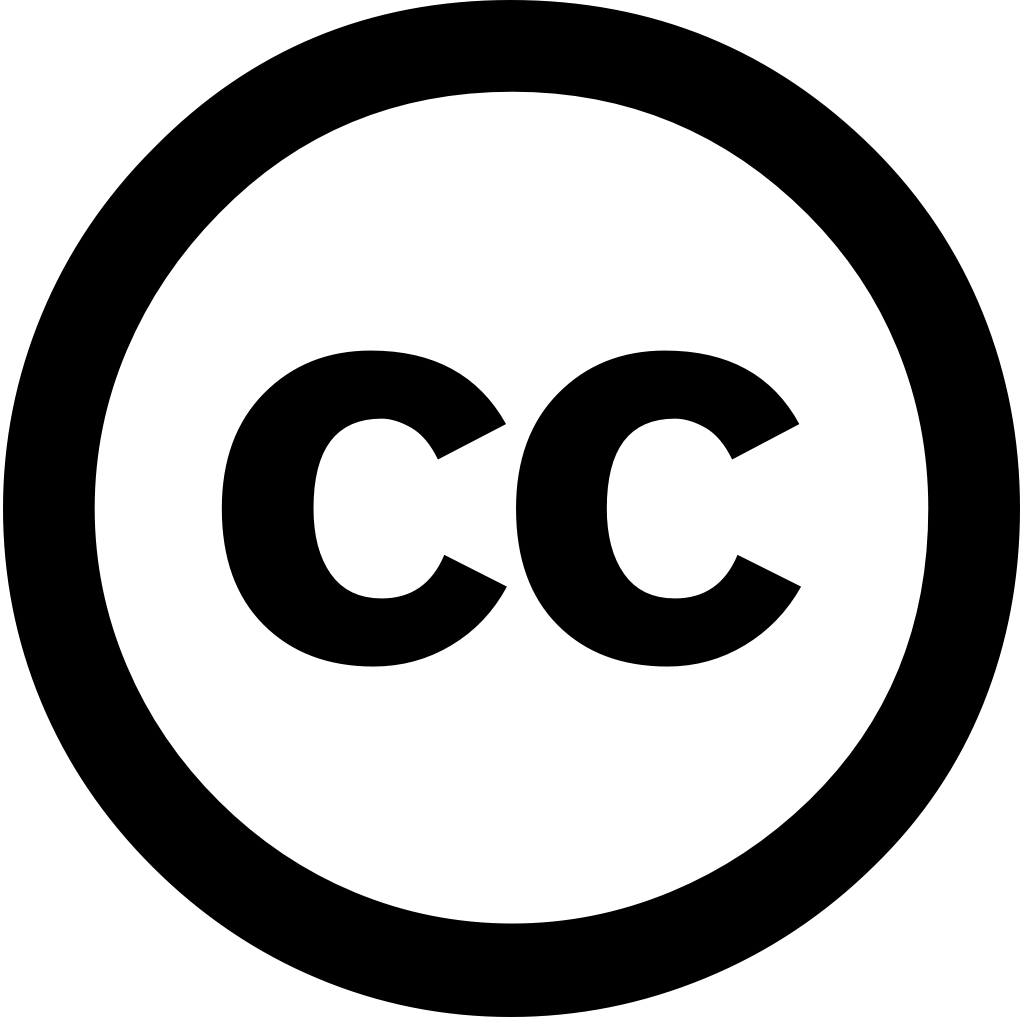
Research Square (Research Square), Journal Year: 2024, Volume and Issue: unknown
Published: July 30, 2024
Language: Английский
Research Square (Research Square), Journal Year: 2024, Volume and Issue: unknown
Published: July 30, 2024
Language: Английский
Ocean Engineering, Journal Year: 2025, Volume and Issue: 326, P. 120907 - 120907
Published: March 11, 2025
Language: Английский
Citations
2Computer Methods and Programs in Biomedicine, Journal Year: 2025, Volume and Issue: 261, P. 108606 - 108606
Published: Jan. 20, 2025
Language: Английский
Citations
1Computer Science Review, Journal Year: 2025, Volume and Issue: 56, P. 100719 - 100719
Published: Jan. 5, 2025
Language: Английский
Citations
0Concurrency and Computation Practice and Experience, Journal Year: 2025, Volume and Issue: 37(4-5)
Published: Feb. 10, 2025
ABSTRACT The morphological characteristics of electrocardiograms (ECGs) serve as a fundamental basis for diagnosing arrhythmias. Convolutional neural networks (CNNs), leveraging their local receptive field properties, effectively capture the features ECG signals and have been extensively employed in automatic diagnosis However, variability duration renders single‐scale convolutional kernels inadequate fully extracting these features. To address this limitation, study proposes multi‐scale parallel joint optimization network (MPJO_CNN). proposed method utilizes varying scales to extract features, further refining via computation implementing strategy enhance classification performance. Experimental results demonstrate that on MIT‐BIH arrhythmia database, not only achieved state‐of‐the‐art performance, with an accuracy 99.41% F1 score 98.09%, but also showed high sensitivity classes fewer samples.
Language: Английский
Citations
0Scientific Reports, Journal Year: 2025, Volume and Issue: 15(1)
Published: Feb. 19, 2025
The limited availability of labeled ECG data restricts the application supervised deep learning methods in detection. Although existing self-supervised approaches have been applied to analysis, they are predominantly image-based, which limits their effectiveness. To address these limitations and provide novel insights, we propose a Temporal-Spatial Self-Supervised Learning (TSSL) method specifically designed for TSSL leverages intrinsic temporal spatial characteristics signals enhance feature representation. Temporally, retain consistent identity information over time, enabling model generate stable representations same individual across different time points while isolating leads preserve unique features. Spatially, from various capture heart's activity perspectives, revealing both commonalities distinct patterns. captures correlations by maintaining consistency relationships between leads. Experimental results on CPSC2018, Chapman, PTB-XL databases demonstrate that introduces new capabilities effectively utilizing information, achieving superior performance compared approaching full-label training with only 10% data. This highlights TSSL's ability deeper insights enhanced extraction beyond mere improvements. We make our code publicly available https://github.com/cwp9731/temporal-spatial-self-supervised-learning.
Language: Английский
Citations
0Journal Of Big Data, Journal Year: 2025, Volume and Issue: 12(1)
Published: March 5, 2025
Language: Английский
Citations
0Neurocomputing, Journal Year: 2025, Volume and Issue: unknown, P. 130172 - 130172
Published: April 1, 2025
Language: Английский
Citations
0Smart Science, Journal Year: 2024, Volume and Issue: unknown, P. 1 - 14
Published: June 10, 2024
Mobile ad hoc networks (MANETs) facilitate rapid deployment due to independence from established infrastructure, relying instead on wireless technology where each node functions as a source, destination, or intermediary router. However, existing techniques fail adequately address security and privacy concerns inherent in MANETs, rendering them impractical for real-world deployment. To tackle these challenges, this paper proposes the Blockchain Based Trusted Distributed Routing Scheme MANET using Latent Encoder Coupled Generative Adversarial Network Optimized with Binary Emperor Penguin Optimizer (LEGAN-BEPO-BCMANET). This scheme leverages blockchain establish fair proof-of-reputation system, ensuring trusted decentralized routing based authenticated token transactions. By integrating (LEGAN) optimized optimizer (BEPO), enhances efficiency security. A comprehensive analysis is performed focusing aspects such double-spending prevention, transaction distinguishability, information integrity, self-modification resilience. The proposed approach implemented NS3 software, evaluation metrics including throughput of transactions average energy consumption recorded. Overall, LEGAN-BEPO-BCMANET offers robust solution challenges faced by paving way practical scenarios. performance technique attains 29.786%, 19.25%, 22.93%, 27.21%, 31.02%, 26.91%, 25.61% greater throughput, compared methods like Blockchain-based BATMAN protocol utilizing an ensemble algorithm (BATMAN-MANET), Block chain-based distributed dropout extreme learning neural network (DEELNN-MANET), secured structure new directed acyclic graph-blockchain internet things environment (DAG-MANET), An Link State Protocol Framework Efficient Video-Packet Transmission Security over (OLSRP-MANET), Auto-metric Graph Neural Technology Protected Dynamic Optimum (AGNN-MANET) Data security-based MANETs under key management process (DSR-MANET) respectively.
Language: Английский
Citations
1Physics of Fluids, Journal Year: 2024, Volume and Issue: 36(12)
Published: Dec. 1, 2024
In the field of geological exploration, accurately distinguishing between different types fluids is crucial for development oil, gas, and mineral resources. Due to scarcity labeled samples, traditional supervised learning methods face significant limitations when processing well log data. To address this issue, paper presents a novel fluid classification method known as Resilient Semi-Supervised Meta-Learning Network (RSSMLN) based on wavelet transform K-means optimization, which combines advantages few-shot semi-supervised learning, aiming optimize recognition in Initially, study employs small set samples train initial model utilizes pseudo-label generation clustering prototypes, thereby enhancing model's accuracy generalization ability. Subsequently, during feature extraction process, preprocessing techniques are introduced enhance time-frequency representation data through multi-scale decomposition. This process effectively captures high-frequency low-frequency features, providing structured information subsequent convolution operations. By employing dual-channel heterogeneous convolutional kernel extractor, RSSMLN can capture subtle features significantly improve accuracy. Experimental results indicate that compared various standard deep models, achieves superior performance identification tasks. research provides reliable solution oilfield applications offers scientific support resource exploration evaluation.
Language: Английский
Citations
1Alexandria Engineering Journal, Journal Year: 2024, Volume and Issue: 105, P. 460 - 470
Published: Aug. 13, 2024
Language: Английский
Citations
1