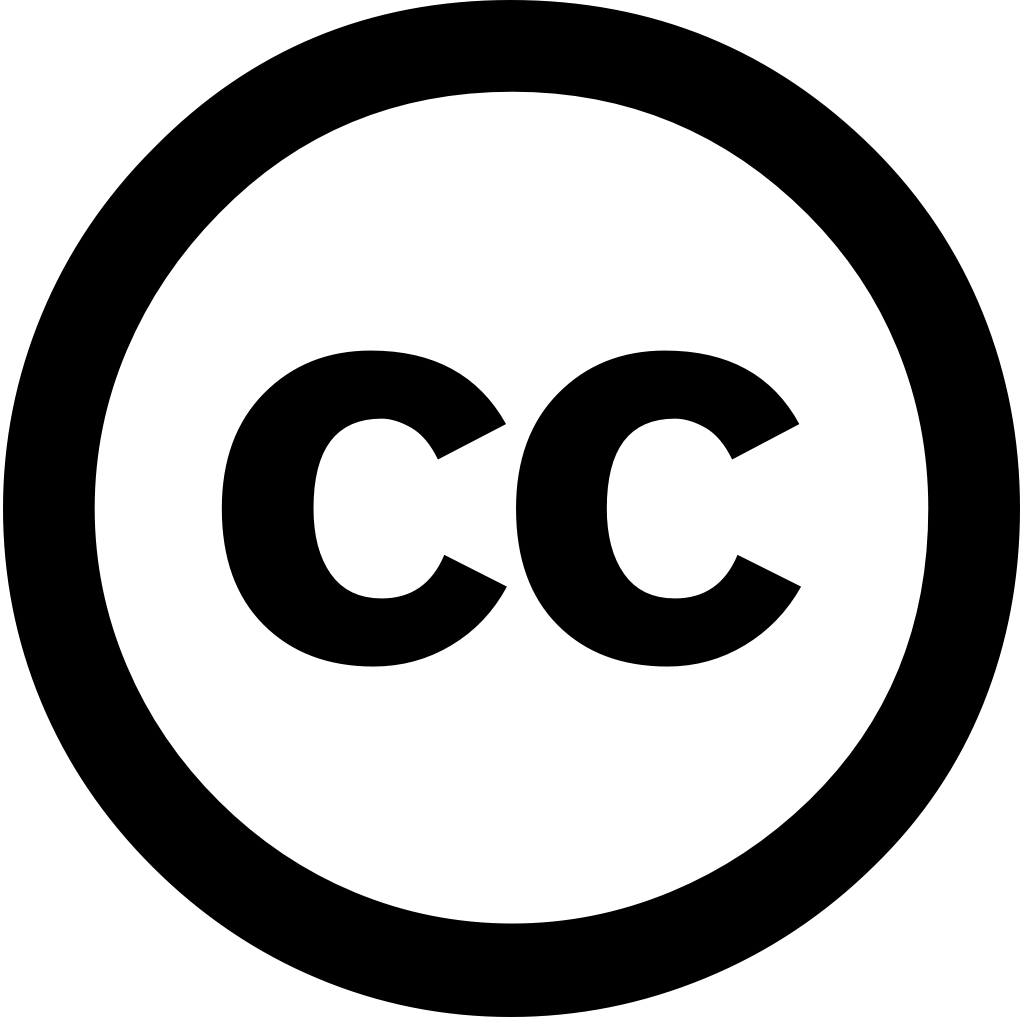
npj Computational Materials, Journal Year: 2025, Volume and Issue: 11(1)
Published: April 14, 2025
Language: Английский
npj Computational Materials, Journal Year: 2025, Volume and Issue: 11(1)
Published: April 14, 2025
Language: Английский
Mechanical Systems and Signal Processing, Journal Year: 2023, Volume and Issue: 200, P. 110535 - 110535
Published: June 27, 2023
Language: Английский
Citations
77Progress in Materials Science, Journal Year: 2024, Volume and Issue: 147, P. 101348 - 101348
Published: July 31, 2024
Language: Английский
Citations
64Acta Materialia, Journal Year: 2024, Volume and Issue: 271, P. 119904 - 119904
Published: April 8, 2024
Language: Английский
Citations
30Nature Communications, Journal Year: 2024, Volume and Issue: 15(1)
Published: March 25, 2024
Abstract High-efficient heat dissipation plays critical role for high-power-density electronics. Experimental synthesis of ultrahigh thermal conductivity boron arsenide (BAs, 1300 W m −1 K ) cooling substrates into the wide-bandgap semiconductor gallium nitride (GaN) devices has been realized. However, lack systematic analysis on transfer across GaN-BAs interface hampers practical applications. In this study, by constructing accurate and high-efficient machine learning interatomic potentials, we perform multiscale simulations heterostructures. Ultrahigh interfacial conductance 260 MW −2 is achieved, which lies in well-matched lattice vibrations BAs GaN. The strong temperature dependence found between 300 to 450 K. Moreover, competition grain size boundary resistance revealed with increasing from 1 nm 1000 μm. Such deep-potential equipped not only promote applications electronics, but also offer approach designing advanced management systems.
Language: Английский
Citations
21Advanced Energy Materials, Journal Year: 2024, Volume and Issue: 14(22)
Published: March 19, 2024
Abstract Lithium‐ion batteries (LIBs) have played an essential role in the energy storage industry and dominated power sources for consumer electronics electric vehicles. Understanding electrochemistry of LIBs at molecular scale is significant improving their performance, stability, lifetime, safety. Classical dynamics (MD) simulations could directly capture atomic motions thus provide dynamic insights into electrochemical processes ion transport during charging discharging that are usually challenging to observe experimentally, which momentous developing with superb performance. This review discusses developments MD approaches using non‐reactive force fields, reactive machine learning potential modeling chemical reactions reactants electrodes, electrolytes, electrode‐electrolyte interfaces. It also comprehensively how interactions, structures, transport, reaction affect electrode capacity, interfacial properties. Finally, remaining challenges envisioned future routes commented on high‐fidelity, effective simulation methods decode invisible interactions LIBs.
Language: Английский
Citations
17Materials Science and Engineering R Reports, Journal Year: 2023, Volume and Issue: 154, P. 100738 - 100738
Published: May 22, 2023
Language: Английский
Citations
34The Journal of Physical Chemistry Letters, Journal Year: 2023, Volume and Issue: 14(7), P. 1808 - 1822
Published: Feb. 10, 2023
Thermoelectric (TE) materials can directly convert heat to electricity and vice versa have broad application potential for solid-state power generation refrigeration. Over the past few decades, efforts been made develop new TE with high performance. However, traditional experiments simulations are expensive time-consuming, limiting development of materials. Machine learning (ML) has increasingly applied study in recent years. This paper reviews progress ML-based material research. The ML predicting optimizing properties materials, including electrical thermal transport optimization functional targeted properties, is reviewed. Finally, future research directions discussed.
Language: Английский
Citations
33Science China Materials, Journal Year: 2025, Volume and Issue: unknown
Published: Jan. 2, 2025
Language: Английский
Citations
1Energy and AI, Journal Year: 2022, Volume and Issue: 8, P. 100153 - 100153
Published: March 12, 2022
In recent years, (big) data science has emerged as the "fourth paradigm" in physical research. Data-driven techniques, e.g. machine learning, are advantageous dealing with problems of high-dimensional features and complex mappings between quantities, which otherwise great difficulty or huge cost other scientific paradigms. past five years so, there been a rapid growth learning-assisted research on thermal transport. this perspective, we review progress intersection learning transport, where methods generally serve surrogate models for predicting transport properties, tools designing structures desired properties exploring mechanisms. We provide perspectives about advantages comparison to physics-based studying properties. also discuss how improve accuracy predictive analytics efficiency structural optimization, guidance better utilizing learning-based advance Finally, identify several outstanding challenges active area well opportunities future developments, including developing suitable small datasets, discovering effective descriptors, generating dataset from experiments validating results experiments, making breakthroughs via new physics.
Language: Английский
Citations
36Nano Energy, Journal Year: 2022, Volume and Issue: 103, P. 107846 - 107846
Published: Sept. 23, 2022
Language: Английский
Citations
36