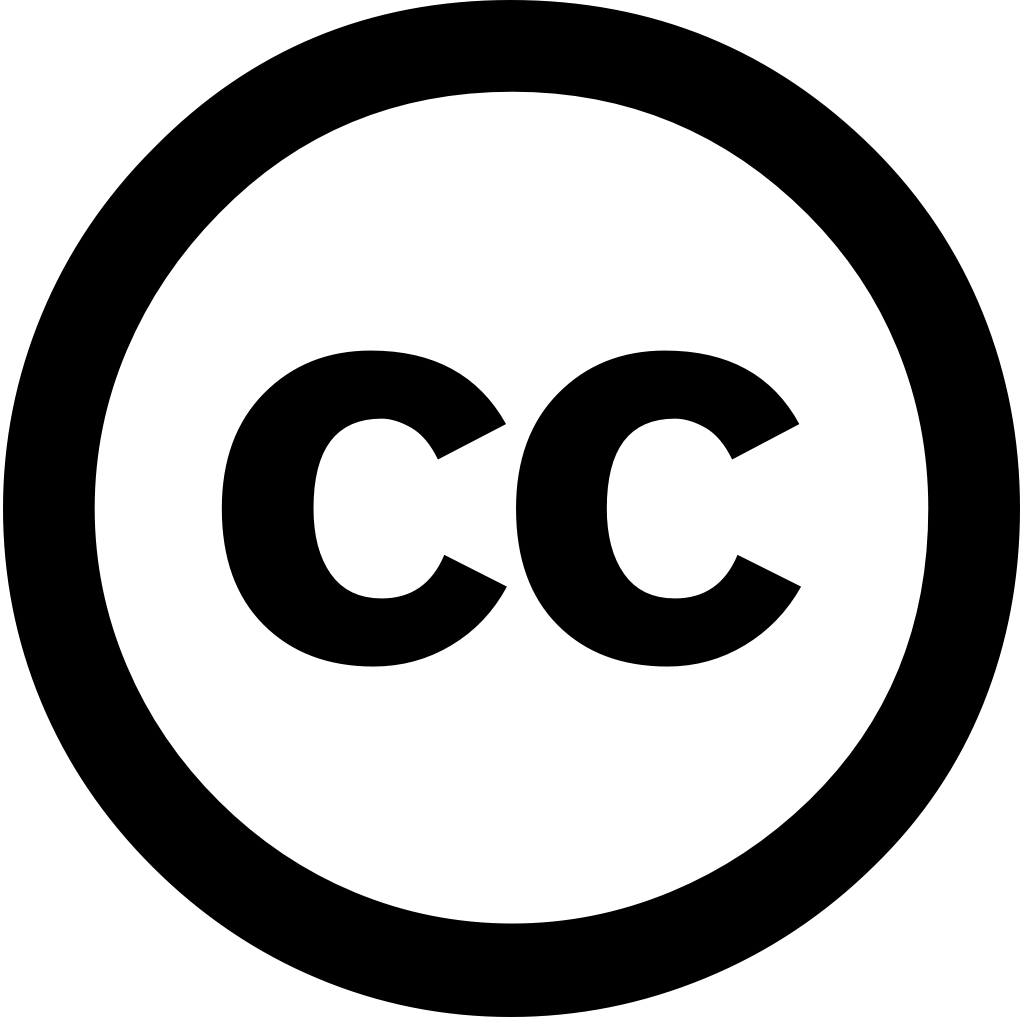
Research Square (Research Square), Journal Year: 2025, Volume and Issue: unknown
Published: March 28, 2025
Language: Английский
Research Square (Research Square), Journal Year: 2025, Volume and Issue: unknown
Published: March 28, 2025
Language: Английский
ISPRS Journal of Photogrammetry and Remote Sensing, Journal Year: 2023, Volume and Issue: 206, P. 311 - 334
Published: Nov. 28, 2023
Language: Английский
Citations
61Computers and Electronics in Agriculture, Journal Year: 2024, Volume and Issue: 217, P. 108577 - 108577
Published: Jan. 5, 2024
Language: Английский
Citations
35Nature Reviews Electrical Engineering, Journal Year: 2024, Volume and Issue: 1(4), P. 251 - 263
Published: March 26, 2024
Language: Английский
Citations
25Sustainability, Journal Year: 2024, Volume and Issue: 16(14), P. 6064 - 6064
Published: July 16, 2024
Originally, the use of hyperspectral images was for military applications, but their has been extended to precision agriculture. In particular, they are used activities related crop classification or disease detection, combining these with machine learning techniques and algorithms. The study a wide range wavelengths observation. These allow monitoring agricultural crops such as cereals, oilseeds, vegetables, fruits, other applications. ranges wavelengths, conditions maturity index nutrient status, early detection some diseases that cause losses in crops, can be studied diagnosed. Therefore, this article proposes technical review main applications perspectives challenges combine artificial intelligence algorithms deep vegetables. A systematic scientific literature carried out using 10-year observation window determine evolution integration technological tools support sustainable agriculture; among findings, information on most documented is highlighted, which cereals citrus fruits due high demand large cultivation areas, well vegetables integrating technologies. Also, being worked summarized classified, wavelength prediction, analysis tasks physiological characteristics production. This useful reference future research, based mainly classification, decision making, implement appropriate
Language: Английский
Citations
20Expert Systems with Applications, Journal Year: 2024, Volume and Issue: 257, P. 125106 - 125106
Published: Aug. 18, 2024
Language: Английский
Citations
16Sensors, Journal Year: 2023, Volume and Issue: 23(21), P. 8966 - 8966
Published: Nov. 3, 2023
As one of the important components Earth observation technology, land use and cover (LULC) image classification plays an essential role. It uses remote sensing techniques to classify specific categories ground as a means analyzing understanding natural attributes Earth’s surface state use. provides information for applications in environmental protection, urban planning, resource management. However, images are usually high-dimensional data have limited available labeled samples, so performing LULC task faces great challenges. In recent years, due emergence deep learning processing methods based on achieved remarkable results, bringing new possibilities research development classification. this paper, we present systematic review deep-learning-based classification, mainly covering following five aspects: (1) introduction main typical networks, how they work, their unique benefits; (2) summary two baseline datasets (pixel-level, patch-level) performance metrics evaluating different models (OA, AA, F1, MIOU); (3) strategies studies, including convolutional neural networks (CNNs), autoencoders (AEs), generative adversarial (GANs), recurrent (RNNs); (4) challenges faced by schemes under training samples; (5) outlooks future
Language: Английский
Citations
30Environmental Modelling & Software, Journal Year: 2024, Volume and Issue: 173, P. 105956 - 105956
Published: Jan. 10, 2024
Language: Английский
Citations
14Letters in Spatial and Resource Sciences, Journal Year: 2024, Volume and Issue: 17(1)
Published: June 24, 2024
Language: Английский
Citations
8Computers and Electronics in Agriculture, Journal Year: 2024, Volume and Issue: 230, P. 109847 - 109847
Published: Dec. 18, 2024
Language: Английский
Citations
8Sustainability, Journal Year: 2023, Volume and Issue: 15(10), P. 7854 - 7854
Published: May 11, 2023
The pace of Land Use/Land Cover (LULC) change has accelerated due to population growth, industrialization, and economic development. To understand analyze this transformation, it is essential examine changes in LULC meticulously. classification a fundamental complex task that plays significant role farming decision making urban planning for long-term development the earth observation system. Recent advances deep learning, transfer remote sensing technology have simplified problem. Deep learning particularly useful addressing issue insufficient training data because reduces need equally distributed data. In study, thirty-nine models were systematically evaluated alongside multiple using consistent set criteria. Our experiments will be conducted under controlled conditions provide valuable insights future research on models. Among our models, ResNet50, EfficientNetV2B0, ResNet152 top performers terms kappa accuracy scores. required three times longer time than EfficientNetV2B0 test computer, while ResNet50 took roughly twice as long. achieved an overall f1-score 0.967 set, with Highway class having lowest score Sea Lake highest.
Language: Английский
Citations
17