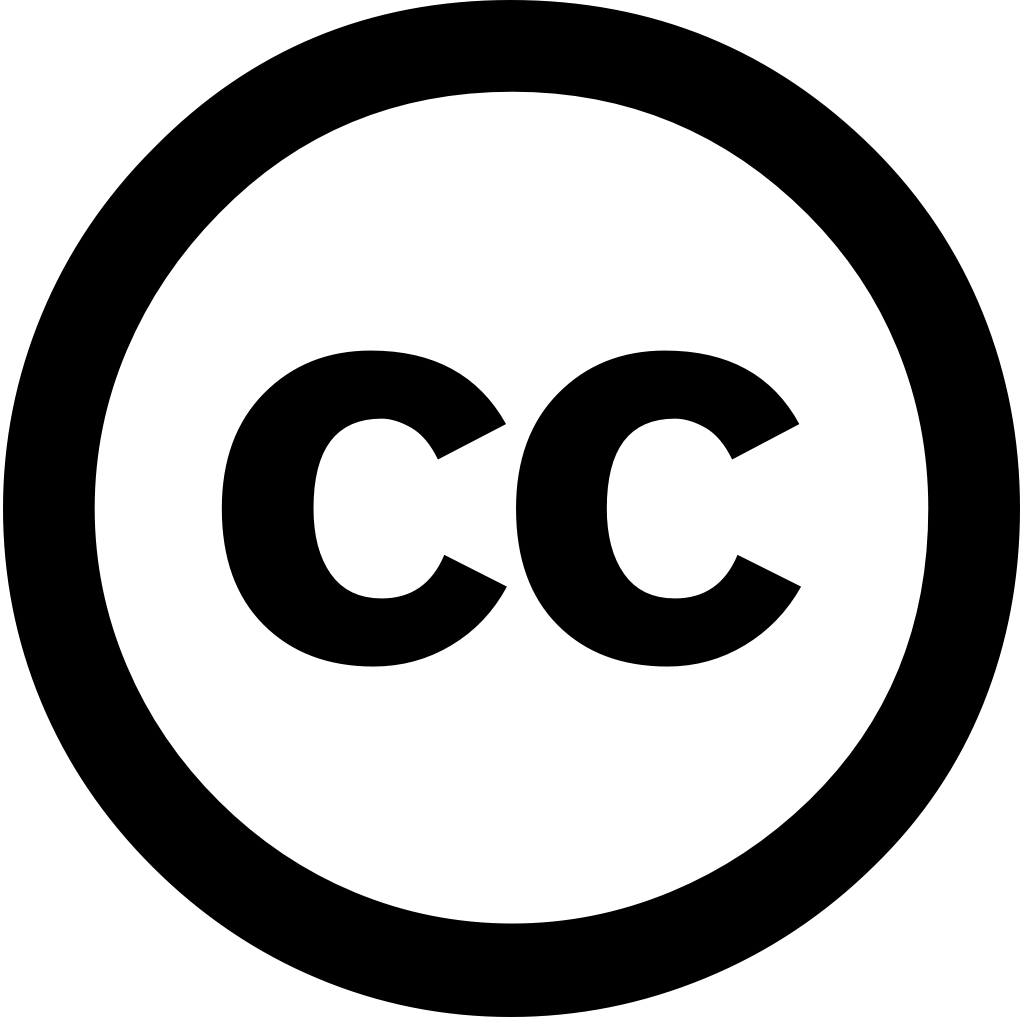
Energy Conversion and Management X, Journal Year: 2024, Volume and Issue: unknown, P. 100767 - 100767
Published: Oct. 1, 2024
Language: Английский
Energy Conversion and Management X, Journal Year: 2024, Volume and Issue: unknown, P. 100767 - 100767
Published: Oct. 1, 2024
Language: Английский
Results in Engineering, Journal Year: 2024, Volume and Issue: 21, P. 101886 - 101886
Published: Feb. 8, 2024
Photovoltaic (PV) panels stand as a prominent solution to meet the world's growing energy demands, due their resistance hard climate conditions, low-cost maintenance, and long lifetime. Nonetheless, integration of PV power into electrical grids poses significant challenge its inherent intermittency. This study aims forecast future based on historical records using Bidirectional Long Short-Term Memory (Bi-LSTM), One-Dimensional Convolutional Neural Network (1D-CNN), Gated Recurrent Unit (GRU). Various performance metrics have been used evaluate compare accuracy three models, including mean squared error, root absolute max error R-squared for evaluation. The prediction photovoltaic values registered in last hour was carried out. Two scenarios investigated, with without nighttime fit models. Results reveal that forecasting models provide exceptional accuracy, achieving correlation coefficient range 96.9–97.2% daytime both scenarios, indicating promising potential these DNNs forecasters optimizing production improving overall system efficiency. GRU, BiLSTM-based showed identical results terms RMSE, MSE MAE while 1D-CNN forecaster accurate second scenario, However, despite this improvement, it still falls behind Bi-LSTM or GRU scenarios.
Language: Английский
Citations
26Results in Engineering, Journal Year: 2024, Volume and Issue: 22, P. 102188 - 102188
Published: May 3, 2024
The home energy management (HEM) sector is going through an enormous change that includes important elements like incorporating green power, enhancing efficiency forecasting and scheduling optimization techniques, employing smart grid infrastructure, regulating the dynamics of optimal trading. As a result, ecosystem players need to clarify their roles, develop effective regulatory structures, experiment with new business models. Peer-to-Peer (P2P) trading seems be one viable options in these conditions, where consumers can sell/buy electricity to/from other users prior totally depending on utility. P2P enables exchange between prosumers, thus provide more robust platform for This strategy decentralizes market than it did previously, opening up possibilities improving trade customers Considering above scenarios, this research provides extensive insight structure, procedure, design, platform, pricing mechanism, approaches, topologies possible futuristic while examining characteristics, pros cons primary goal determining whichever approach most appropriate given situation HEMs. Moreover, HEMs load framework simulation model also proposed analyze network critically, paving technical directions scientific researchers. With cooperation, age technological advancements ushering intelligent, interconnected, reactive urban environment are brought life. In sense, path living entails reinventing as well how people interact perceive dwellings larger city. Finally, work comprehensive overview challenges terms strategies, solutions, future prospects.
Language: Английский
Citations
20Results in Engineering, Journal Year: 2023, Volume and Issue: 20, P. 101428 - 101428
Published: Sept. 26, 2023
Wastewater treatment plants (WWTPs) are energy-intensive facilities that play a critical role in meeting stringent effluent quality regulations. Accurate prediction of energy consumption WWTPs is essential for cost savings, process optimization, regulatory compliance, and reducing carbon footprint. This paper introduces an efficient approach predicting WWTPs, leveraging deep learning models, data augmentation, feature selection. Specifically, Spline Cubic interpolation enriches the dataset, while Random Forest model identifies important features. The study investigates impact lagged to capture temporal dependencies. Comparative analysis five models on original augmented datasets from Melbourne WWTP demonstrates substantial performance improvement with data. Incorporating further enhances accuracy, providing valuable insights effective management. Notably, Long Short-Term Memory (LSTM) Bidirectional Gated Recurrent Unit (BiGRU) achieve Mean Absolute Percentage Error (MAPE) values 1.36% 1.436%, outperforming state-of-the-art methods.
Language: Английский
Citations
37Computers & Electrical Engineering, Journal Year: 2024, Volume and Issue: 114, P. 109063 - 109063
Published: Jan. 14, 2024
Language: Английский
Citations
15Results in Engineering, Journal Year: 2024, Volume and Issue: 22, P. 101993 - 101993
Published: March 15, 2024
In the context of large-scale grid connection new energy, short-term load forecasting is a vital and challenging task for power system to balance supply demand. To effectively improve accuracy, method proposed aiming mine characteristics data study application artificial intelligence algorithms. this paper, seasonal trend decomposition using loess (STL) firstly applied decompose into trend, residual components component with highest complexity further decomposed by complete ensemble empirical mode adaptive noise (CEEMDAN) approach. Secondly, in order reduce number components, improved hierarchical clustering technique cluster all intrinsic functions (IMFs) obtained CEEMDAN high-frequency low-frequency components. Then, different network models are trained get prediction results total value achieved stacking them. Finally, national demand dataset Great Britain 2021–2022 used conduct ablation comparative experiments. The mean absolute percentage error (MAPE) root square (RMSE) 2.064% 724.01 MW, respectively, which verified effectiveness advancement method.
Language: Английский
Citations
13IEEE Access, Journal Year: 2024, Volume and Issue: 12, P. 23504 - 23513
Published: Jan. 1, 2024
Load forecasting in Smart Grids (SG) is a major module of current energy management systems, that play vital role optimizing resource allocation, improving grid stability, and assisting the combination renewable sources (RES). It contains predictive electricity consumption forms over certain time intervals. Forecasting remains stimulating task as load data has exhibited changing patterns because factors such weather change shifts usage behaviour. The beginning advanced analytics machine learning (ML) approaches; particularly deep (DL) mostly enhanced accuracy. Deep neural networks (DNNs) namely Long Short-Term Memory (LSTM) Convolutional Neural Networks (CNN) have achieved popularity for their capability to capture difficult temporal dependencies data. This study designs Short-Load scheme using Hybrid Learning Beluga Whale Optimization (LFS-HDLBWO) approach. intention LFS-HDLBWO technique predict SG environment. To accomplish this, initially uses Z-score normalization approach scaling input dataset. Besides, makes use convolutional bidirectional long short-term memory with an autoencoder (CBLSTM-AE) model prediction purposes. Finally, BWO algorithm could be used optimal hyperparameter selection CBLSTM-AE algorithm, which helps enhance overall results. A wide-ranging experimental analysis was made illustrate better results method. obtained value demonstrates outstanding performance system other existing DL algorithms minimum average error rate 3.43 2.26 under FE Dayton datasets, respectively.
Language: Английский
Citations
12Results in Engineering, Journal Year: 2024, Volume and Issue: 21, P. 101747 - 101747
Published: Jan. 5, 2024
Integrating uncertainties associated with photovoltaic (PV) generation is an important aspect used to ensure the planning and operation of power distribution systems. Therefore, this research proposed uncertainty model for PV by combining methods change point detection, cyclic k-means clustering (KMC), Monte Carlo simulation (MCS) freedman diaconis estimator (FDE), KMC soft-dynamic time warping (DTW). Firstly, a seasonal split was performed using detection techniques identify shifts in global horizontal irradiance (GHI) points. Secondly, GHI generated MCS each season FDE method optimize number bins data distribution. Finally, curve from simplified through soft-DTW metric, which facilitated more straightforward representation profile. The impact profile integration on quasi-dynamic flow tested IEEE 33 Bus system. voltage feeder significantly impacted integration, specifically during hours when high produced. For instance, at 11:00 a.m., values buses 18, 17, increased 0.933, 0.934, 0.935, respectively, 0.982, 0.980, 0.972. Similarly, value losses, greater produced certain hour, smaller losses generated. experimental results indicated that changes electrical parameters over according input
Language: Английский
Citations
11Results in Engineering, Journal Year: 2024, Volume and Issue: 23, P. 102773 - 102773
Published: Aug. 24, 2024
Language: Английский
Citations
8Results in Engineering, Journal Year: 2025, Volume and Issue: unknown, P. 104158 - 104158
Published: Jan. 1, 2025
Language: Английский
Citations
1Results in Engineering, Journal Year: 2024, Volume and Issue: 21, P. 101766 - 101766
Published: Jan. 7, 2024
The functioning of photovoltaic (PV) systems mainly depends on the climatic conditions, including incident solar irradiance intensity and module temperature. Thus, operating temperature are key factors for assessing monitoring performance PV systems. This work develops a cells-based sensor accurate measurement both proposed relies Neural Network (NN) soft sensor, trained to estimate modules without direct measurements. NN model uses short circuit current (ISC) open-circuit voltage (VOC) reference as inputs estimation. accuracy is validated through indoor outdoor experiments using emulator real panel, respectively. Indoor results affirmed tracking capability estimator temperature, in constant rapidly changing sudden changes. achieved an exceptional level with Mean Absolute Percentage Error (MAPE) 1.45% 1.64% Similarly, real-time data further validate obtained Normalized Root Square (NRMSE) 1.22% 3.50% outperforming other methods published literature.
Language: Английский
Citations
7