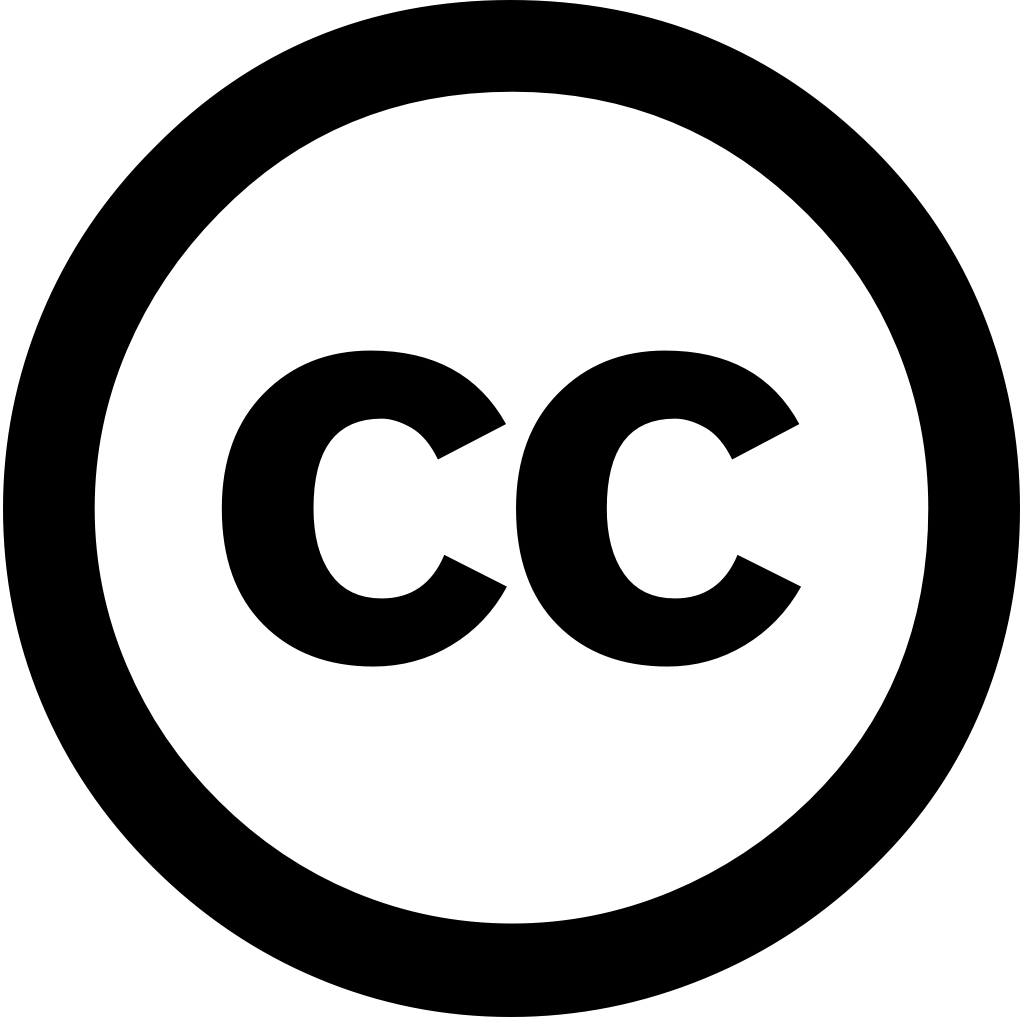
International Journal of Applied Earth Observation and Geoinformation, Journal Year: 2025, Volume and Issue: 139, P. 104559 - 104559
Published: April 28, 2025
Language: Английский
International Journal of Applied Earth Observation and Geoinformation, Journal Year: 2025, Volume and Issue: 139, P. 104559 - 104559
Published: April 28, 2025
Language: Английский
Remote Sensing of Environment, Journal Year: 2023, Volume and Issue: 295, P. 113714 - 113714
Published: July 18, 2023
Language: Английский
Citations
80IEEE Transactions on Geoscience and Remote Sensing, Journal Year: 2024, Volume and Issue: 62, P. 1 - 11
Published: Jan. 1, 2024
Solar-induced
chlorophyll
fluorescence
(SIF)
shows
potential
in
exploring
plant
responses
to
environmental
changes
caused
by
extreme
climatic
factors.
However,
how
accurately
assess
climate
stresses
(especially
the
low-temperature
stress)
suffered
on
crops
at
regional
scale
a
systematic
approach
has
not
been
extensively
explored.
In
this
study,
we
developed
vegetation
stress
index
(CVSI)
and
quantify
impacts
of
large
scales
combining
TROPOspheric
Monitoring
Instrument
(TROPOMI)
SIF
land
surface
temperature
(LST)
data
through
an
easy-to-operate
approach.
This
was
employed
identify
conditions
Henan
Province's
winter
wheat
2018.
Results
indicate
that,
influenced
characteristics,
northern
part
Province
experienced
more
severe
than
those
southern
part.
The
daily
average
values
reductions
0.74,
0.45,
0.61,
0.86
mW
Language: Английский
Citations
36International Journal of Applied Earth Observation and Geoinformation, Journal Year: 2024, Volume and Issue: 127, P. 103643 - 103643
Published: Jan. 9, 2024
Corn is the dominant crop planted in Northeast China, and its accurate timely mapping important for food security agricultural management China. However, absence of enough labels challenging corn a regional area using machine learning methods or deep methods. In this study, an efficient way automatic labelling areas by combining Global Ecosystem Dynamics Investigation (GEDI) data Sentinel-2 images proposed. We explore height vertical structure differences between other crops derived from GEDI features generate automatically referencing type products transferring models historical years. The trained networks points decision trees Random Forest (RF) classifier can be transferred to arbitrary target are combined perform wall-to-wall random forest algorithm GEDI-based labels. This approach used map China 2019 2022, classification results validated independent collected field campaigns 2023, published maps, official statistics. Our reveal that our proposed method achieves high accuracy robustness with average overall 0.91 testing spatial-type stratified sampling. correlation coefficient (R2) classified result statistical reach 0.96 0.98, respectively. These demonstrate potential label collection vegetation difference provide new on large-scale.
Language: Английский
Citations
13Remote Sensing, Journal Year: 2024, Volume and Issue: 16(2), P. 277 - 277
Published: Jan. 10, 2024
Timely and accurate rice spatial distribution maps play a vital role in food security social stability. Early-season mapping is of great significance for yield estimation, crop insurance, national policymaking. Taking Tongjiang City Heilongjiang Province with strong heterogeneity as study area, hierarchical K-Means binary automatic classification method based on phenological feature optimization (PFO-HKMAR) proposed, using Google Earth Engine platform Sentinel-1/2, Landsat 7/8 data. First, SAR backscattering intensity time series reconstructed used to construct optimize polarization characteristics. A new index named VH-sum built, which defined the summation VH specific periods temporal changes characteristics different land cover types. Then comes selection, optimization, reconstruction optical Finally, PFO-HKMAR established Simple Non-Iterative Clustering. can achieve early-season one month before harvest, overall accuracy, Kappa, F1 score reaching 0.9114, 0.8240 0.9120, respectively (F1 greater than 0.9). Compared two datasets Northeast China ARM-SARFS, scores are improved by 0.0507–0.1957, 0.1029–0.3945, 0.0611–0.1791, respectively. The results show that be promoted enable mapping, provide valuable timely information stakeholders decision makers.
Language: Английский
Citations
10Scientific Data, Journal Year: 2024, Volume and Issue: 11(1)
Published: May 2, 2024
Abstract China, as the world’s biggest soybean importer and fourth-largest producer, needs accurate mapping of its planting areas for global food supply stability. The challenge lies in gathering collating ground survey data different crops. We proposed a spatiotemporal migration method leveraging vegetation indices’ temporal characteristics. This uses feature space six integrals from crops’ phenological curves concavity-convexity index to distinguish non-soybean samples cropland. Using limited number actual our method, we extracted features optical time-series images throughout growing season. cloud rain-affected were supplemented with SAR data. then used random forest algorithm classification. Consequently, developed 10-meter resolution ChinaSoybean10 maps ten primary soybean-producing provinces 2019 2022. map showed an overall accuracy about 93%, aligning significantly statistical yearbook data, confirming reliability. research aids growth monitoring, yield estimation, strategy development, resource management, scarcity mitigation, promotes sustainable agriculture.
Language: Английский
Citations
8ISPRS Journal of Photogrammetry and Remote Sensing, Journal Year: 2024, Volume and Issue: 213, P. 14 - 32
Published: May 25, 2024
Language: Английский
Citations
8Remote Sensing, Journal Year: 2024, Volume and Issue: 16(8), P. 1376 - 1376
Published: April 13, 2024
Crop classification is indispensable for agricultural monitoring and food security, but early-season mapping has remained challenging. Synthetic aperture radar (SAR), such as RADARSAT Constellation Mission (RCM) Sentinel-1, can meet higher requirements on the reliability of satellite data acquisition with all-weather all-day imaging capability to supply dense observations in early crop season. This study applied local window attention transformer (LWAT) time-series SAR data, including RCM classification. The performance this integration was evaluated over crop-dominated regions (corn, soybean wheat) southwest Ontario, Canada. Comparative analyses against several machine learning deep methods revealed superiority LWAT, achieving an impressive F1-score 97.96% a Kappa coefficient 97.08% northern region F1-scores 98.07% 97.02% southern when leveraging from respectively. Additionally, by incremental procedure, evolution accuracy determined Sentinel-1 analyzed, which demonstrated that performed better at beginning season could achieve comparable achieved utilizing both datasets. Moreover, stem elongation corn identified crucial phenological stage acquire acceptable maps explores potential provide reliable prior information enough assist in-season production forecasting decision making.
Language: Английский
Citations
7Remote Sensing of Environment, Journal Year: 2024, Volume and Issue: 314, P. 114387 - 114387
Published: Aug. 28, 2024
Language: Английский
Citations
7Earth system science data, Journal Year: 2024, Volume and Issue: 16(7), P. 3213 - 3231
Published: July 10, 2024
Abstract. Soybean, an essential food crop, has witnessed a steady rise in demand recent years. There is lack of high-resolution annual maps depicting soybean-planting areas China, despite China being the world's largest consumer and fourth-largest producer soybean. To address this gap, we developed novel Regional Adaptation Spectra-Phenology Integration method (RASP) based on Sentinel-2 remote sensing images from Google Earth Engine (GEE) platform. We utilized various auxiliary data (e.g., cropland layer, detailed phenology observations) to select specific spectra indices that differentiate soybeans most effectively other crops across regions. These features were then input for unsupervised classifier (K-means), likely type was determined by cluster assignment dynamic time warping (DTW). For first time, generated dataset with high spatial resolution 10 m, spanning 2017 2021 (ChinaSoyArea10m). The R2 values between mapping results census at both county prefecture levels consistently around 0.85 2017–2020. Moreover, overall accuracy field level 2017, 2018, 2019 77.08 %, 85.16 86.77 respectively. Consistency improved (R2 increased 0.53 0.84) compared existing m crop-type Northeast (Crop Data Layer, CDL) samples supervised classification methods. ChinaSoyArea10m very spatially consistent two datasets (CDL GLAD (Global Land Analysis Discovery) maize–soybean map). provides important information sustainable soybean production management as well agricultural system modeling optimization. can be downloaded open-data repository (DOI: https://doi.org/10.5281/zenodo.10071427, Mei et al., 2023).
Language: Английский
Citations
5ISPRS Journal of Photogrammetry and Remote Sensing, Journal Year: 2024, Volume and Issue: 215, P. 271 - 291
Published: July 16, 2024
Near real-time (NRT) crop type mapping plays a crucial role in modeling development, managing food supply chains, and supporting sustainable agriculture. The low-latency updates on distribution also help assess the impacts of weather extremes climate change agricultural production timely fashion, aiding identification early risks insecurity as well rapid assessments damage. Yet NRT is challenging due to obstacle acquiring reference labels during current season for model building. Meanwhile, models constructed with historical corresponding satellite imagery may not be applicable spatiotemporal variability phenology. difficulty characterizing phenology remains significant hurdle mapping. To tackle these issues, novel emergence-based thermal phenological framework (EMET) proposed this study field-level EMET comprises three key components: hybrid deep learning image fusion, thermal-based normalization, characterization. fusion integrates super-resolution convolutional neural network (SRCNN) long short-term memory (LSTM) generate daily observations high spatial resolution NRT. normalization innovatively synthesizes within-season emergence (WISE) time accumulation throughout growing season, normalize progress derived from temporally dense imagery. normalized series are then fed into an advanced classifier, self-attention based LSTM (SAtLSTM) model, identify types. Results Illinois Minnesota U.S. Corn Belt suggest that significantly enhances scalability A consistently higher overall accuracy yielded by compared calendar-based WISE-based benchmark scenarios. When transferred different sites testing years, maintains advantage over 5% early- mid-season. Moreover, reaches 85% month earlier than benchmarks, it can accurately characterize types 90% late July. F1 scores both corn soybeans achieve around paves way large-scale satellite-based at field level, which largely reduce market volatility enhance security, benefit variety applications optimize management towards more production.
Language: Английский
Citations
5