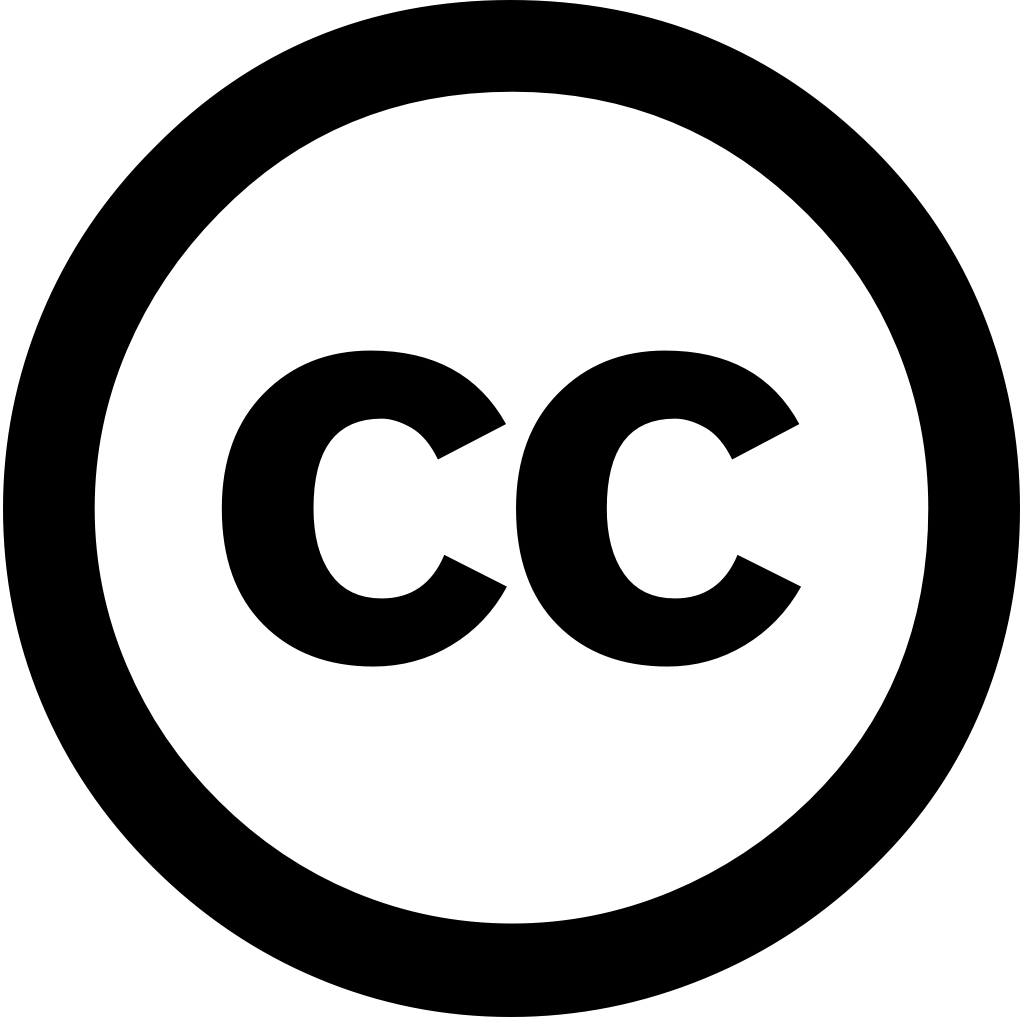
IEEE Access, Journal Year: 2024, Volume and Issue: 12, P. 182799 - 182813
Published: Jan. 1, 2024
Language: Английский
IEEE Access, Journal Year: 2024, Volume and Issue: 12, P. 182799 - 182813
Published: Jan. 1, 2024
Language: Английский
Emirates Journal of Food and Agriculture, Journal Year: 2024, Volume and Issue: 36, P. 1 - 9
Published: April 18, 2024
The impact of deep learning (DL) is substantial across numerous domains, particularly in agriculture. Within this context, our study focuses on the classification problematic soybean seeds. dataset employed encompasses five distinct classes, totaling 5513 images. Our model, based InceptionV3 architecture, undergoes modification with addition supplementary layers to enhance efficiency and performance. Techniques such as transfer learning, adaptive rate adjustment (to 0.001), model checkpointing are integrated optimize accuracy. During initial evaluation, achieved 88.07% accuracy training 86.67% validation. Subsequent implementation tuning strategies significantly improves Augmenting architecture additional layers, including Average Pooling, Flatten, Dense, Dropout, Softmax, plays a pivotal role enhancing Evaluation metrics, precision, recall, F1-score, underscore model’s effectiveness. Precision ranges from 0.9706 1.0000, while recall values demonstrate high capture all classes. reflecting balance between precision exhibits remarkable performance ranging 0.9851 1.0000. Comparative analysis existing studies reveals competitive 98.73% by proposed model. While variations exist specific purposes datasets among studies, showcases promising seed classification, contributing advancements agricultural technology for crop health assessment management.
Language: Английский
Citations
20Sensors, Journal Year: 2025, Volume and Issue: 25(4), P. 1248 - 1248
Published: Feb. 18, 2025
Navigation systems are developing rapidly; nevertheless, tasks becoming more complex, significantly increasing the number of challenges for robotic systems. can be separated into global and local navigation. While navigation works according to predefined data about environment, uses sensory dynamically react adjust trajectory. Tasks complex with addition dynamic obstacles, multiple robots, or, in some cases, inspection places that not physically reachable by humans. Cognitive require only detecting an object but also evaluating it without direct recognition. For this purpose, sensor fusion methods employed. However, sensors different physical nature sometimes cannot directly extract required information. As a result, AI increasingly popular acquired information controlling generating robot trajectories. In work, review mobile localization is presented comparing them listing advantages disadvantages their combinations. Also, integration path-planning looked into. Moreover, analyzed evaluated. Furthermore, concept channel navigation, designed based on research literature, presented. Lastly, discussion conclusions drawn.
Language: Английский
Citations
2Agriculture, Journal Year: 2024, Volume and Issue: 14(7), P. 978 - 978
Published: June 23, 2024
Accurately classifying the quality of apples is crucial for maximizing their commercial value. Deep learning techniques are being widely adopted apple classification tasks, achieving impressive results. While existing research excels at variety, size, shape, and defects, color deformity analysis remain an under-explored area. Therefore, this study investigates feasibility utilizing convolutional neural networks (CNN) to classify based on machine vision technology. Firstly, a custom-assembled system was constructed collecting images. Then, image processing performed extract largest fruit diameter from 45 images taken each apple, establishing dataset. Three classic CNN models (AlexNet, GoogLeNet, VGG16) were employed with parameter optimization three-category task (non-deformed slice–red non-deformed stripe–red deformed apple) features. VGG16 achieved best results accuracy 92.29%. AlexNet GoogLeNet 91.66% 88.96% accuracy, respectively. Ablation experiments model, which found that block contributed task. Finally, prediction using conducted 150 90.50%, comparable or better than other models. This provides insights into deep methods.
Language: Английский
Citations
7Comprehensive Reviews in Food Science and Food Safety, Journal Year: 2024, Volume and Issue: 23(6)
Published: Nov. 1, 2024
Food fraud undermines consumer trust, creates economic risk, and jeopardizes human health. Therefore, it is essential to develop efficient technologies for rapid reliable analysis of food quality safety authentication. Machine vision-based methods have emerged as promising solutions the nondestructive authenticity quality. The Industry 4.0 revolution has introduced new trends in this field, including use deep learning (DL), a subset artificial intelligence, which demonstrates robust performance generalization capabilities, effectively extracting features, processing extensive data. This paper reviews recent advances machine vision various DL-based algorithms authentication, DL lightweight DL, used such adulteration identification, variety freshness detection, identification by combining them with system or smartphones portable devices. review explores limitations challenges include overfitting, interpretability, accessibility, data privacy, algorithmic bias, design deployment DLs, miniaturization sensing Finally, future developments field are discussed, development real-time detection systems that incorporate combination expansion databases. Overall, techniques expected enable faster, more affordable, accurate authentication methods.
Language: Английский
Citations
5MethodsX, Journal Year: 2024, Volume and Issue: 13, P. 103051 - 103051
Published: Nov. 16, 2024
Language: Английский
Citations
5Deleted Journal, Journal Year: 2025, Volume and Issue: 67(1)
Published: Feb. 1, 2025
Language: Английский
Citations
0Measurement, Journal Year: 2025, Volume and Issue: 253, P. 117527 - 117527
Published: April 16, 2025
Language: Английский
Citations
0Sensors, Journal Year: 2025, Volume and Issue: 25(8), P. 2433 - 2433
Published: April 12, 2025
The high nutritional and medicinal value of apples has contributed to their widespread cultivation worldwide. Unfavorable factors in the healthy growth trees extensive orchard work are threatening profitability apples. This study reviewed deep learning combined with computer vision for monitoring apple tree fruit production processes past seven years. Three types models were used real-time target recognition tasks: detection including You Only Look Once (YOLO) faster region-based convolutional network (Faster R-CNN); classification Alex (AlexNet) residual (ResNet); segmentation (SegNet), mask regional neural (Mask R-CNN). These have been successfully applied detect pests diseases (located on leaves, fruits, trunks), organ (including blossoms, branches), yield, post-harvest defects. introduced methods, outlined current research these methods production. advantages disadvantages discussed, difficulties faced future trends summarized. It is believed that this important construction smart orchards.
Language: Английский
Citations
0Procedia Computer Science, Journal Year: 2025, Volume and Issue: 257, P. 127 - 132
Published: Jan. 1, 2025
Language: Английский
Citations
0Advances in computational intelligence and robotics book series, Journal Year: 2025, Volume and Issue: unknown, P. 37 - 62
Published: March 7, 2025
This chapter explores deep learning techniques for image clustering and classification, crucial tasks in computer vision. It discusses unsupervised methods supervised classification approaches, including traditional like k-means hierarchical clustering. The also highlights the transformative impact of CNNs, ensemble methods, transfer classification. uses case studies fruit agriculture brain tumor medical imaging to illustrate real-world applicability these models. Challenges data limitations, computational requirements, explainability are discussed. Future trends include self-supervised multimodal aims guide researchers practitioners leveraging effective across various domains.
Language: Английский
Citations
0