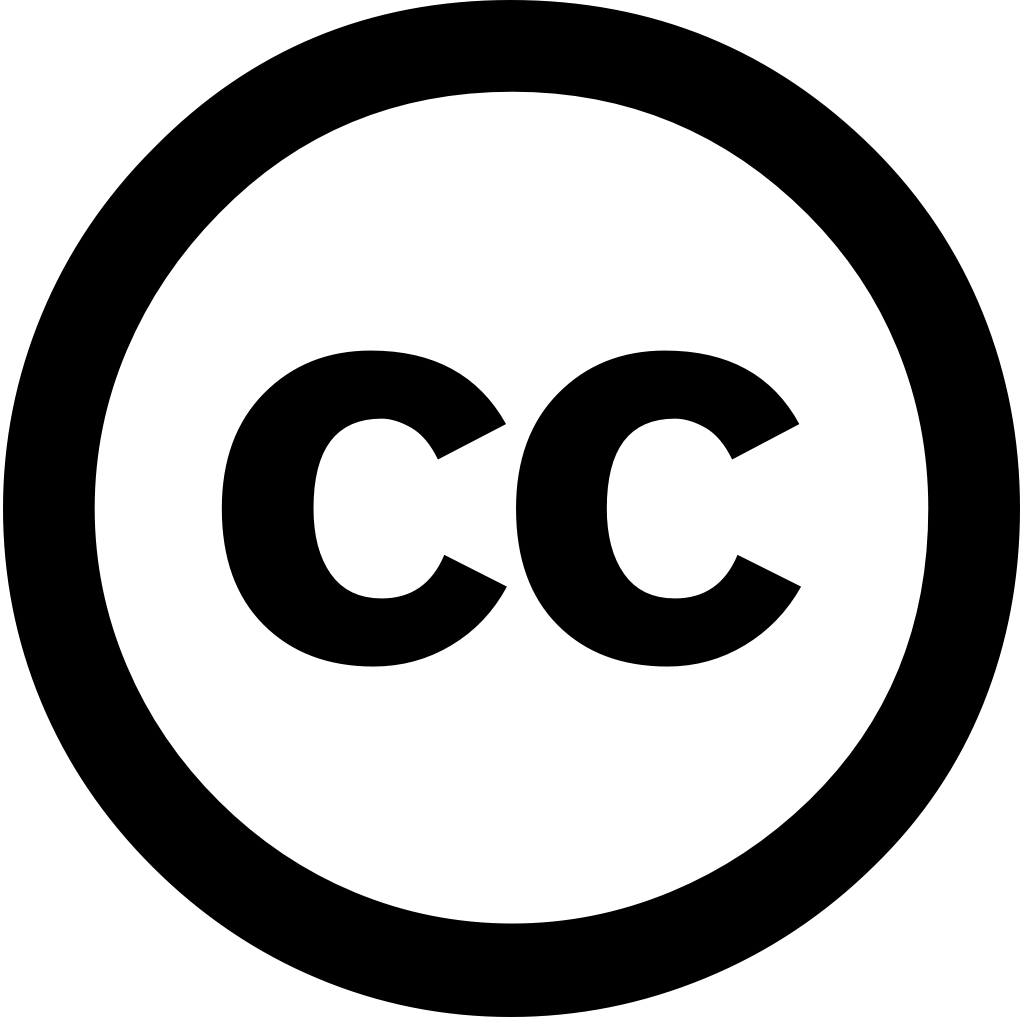
Composites Science and Technology, Journal Year: 2025, Volume and Issue: unknown, P. 111127 - 111127
Published: Feb. 1, 2025
Language: Английский
Composites Science and Technology, Journal Year: 2025, Volume and Issue: unknown, P. 111127 - 111127
Published: Feb. 1, 2025
Language: Английский
Sustainable Energy Technologies and Assessments, Journal Year: 2024, Volume and Issue: 73, P. 104097 - 104097
Published: Nov. 29, 2024
Language: Английский
Citations
4Computers & Chemical Engineering, Journal Year: 2025, Volume and Issue: unknown, P. 109049 - 109049
Published: Feb. 1, 2025
Language: Английский
Citations
0Materials Circular Economy, Journal Year: 2025, Volume and Issue: 7(1)
Published: Feb. 22, 2025
Language: Английский
Citations
0Remote Sensing, Journal Year: 2025, Volume and Issue: 17(5), P. 774 - 774
Published: Feb. 23, 2025
Wheat (Triticum aestivum L.) is one of the world’s primary food crops, and timely accurate yield prediction essential for ensuring security. There has been a growing use remote sensing, climate data, their combination to estimate yields, but optimal indices time window wheat in arid regions remain unclear. This study was conducted (1) assess performance widely recognized sensing predict at different growth stages, (2) evaluate predictive accuracy machine learning models, (3) determine appropriate period regions, (4) impact parameters on model accuracy. The vegetation indices, due proven effectiveness, used this include Normalized Difference Vegetation Index (NDVI), Enhanced (EVI), Atmospheric Resistance (ARVI). Moreover, four viz. Decision Trees (DTs), Random Forest (RF), Gradient Boosting (GB), Bagging (BTs), were evaluated region. whole divided into three windows: tillering grain filling (December 15–March), stem elongation (January heading (February–March 15). developed Google Earth Engine (GEE), combining data. results showed that RF with ARVI could accurately maturity stages an R2 > 0.75 error less than 10%. stage identified as regions. While delivered best results, GB EVI slightly lower precision still outperformed other models. It concluded multisource data models promising approach
Language: Английский
Citations
0Composites Science and Technology, Journal Year: 2025, Volume and Issue: unknown, P. 111127 - 111127
Published: Feb. 1, 2025
Language: Английский
Citations
0