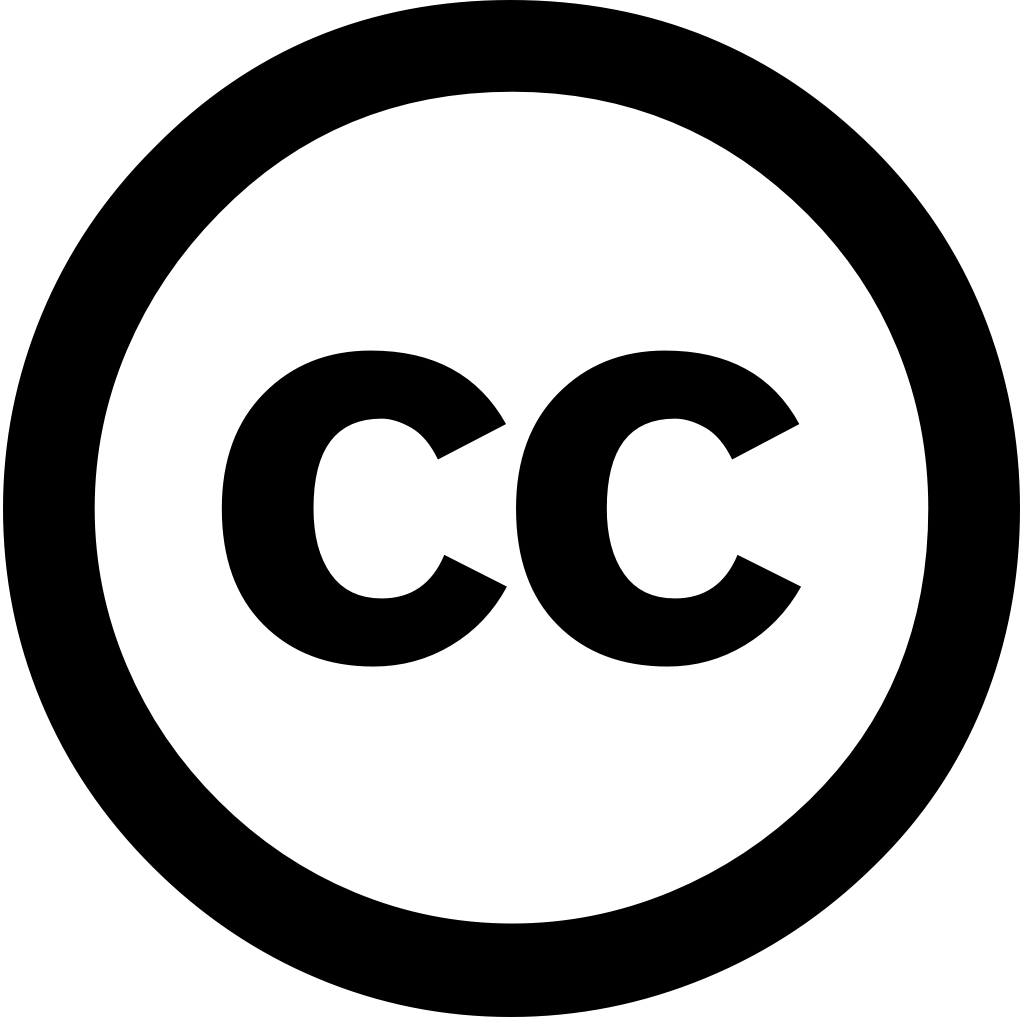
International Journal of Disaster Risk Reduction, Journal Year: 2024, Volume and Issue: unknown, P. 105129 - 105129
Published: Dec. 1, 2024
Language: Английский
International Journal of Disaster Risk Reduction, Journal Year: 2024, Volume and Issue: unknown, P. 105129 - 105129
Published: Dec. 1, 2024
Language: Английский
Sustainable Cities and Society, Journal Year: 2024, Volume and Issue: 108, P. 105496 - 105496
Published: May 5, 2024
Language: Английский
Citations
24International Journal of Disaster Risk Reduction, Journal Year: 2025, Volume and Issue: 117, P. 105170 - 105170
Published: Jan. 5, 2025
Language: Английский
Citations
4Sustainable Cities and Society, Journal Year: 2024, Volume and Issue: 108, P. 105508 - 105508
Published: May 5, 2024
Language: Английский
Citations
15Sustainable Cities and Society, Journal Year: 2024, Volume and Issue: 112, P. 105619 - 105619
Published: July 3, 2024
Language: Английский
Citations
9Applied Sciences, Journal Year: 2025, Volume and Issue: 15(1), P. 465 - 465
Published: Jan. 6, 2025
Emergency situation awareness during sudden natural disasters presents significant challenges. Traditional methods, characterized by low spatial and temporal resolution as well coarse granularity, often fail to comprehensively capture disaster situations. However, social media platforms, a vital source of sensing, offer potential supplement situational awareness. This paper proposes an innovative framework for based on multimodal data from identify content related typhoon disasters. Integrating text image facilitates near real-time monitoring the public perspective. In this study, Typhoon Haikui (Strong No. 11 2023) was chosen case study validate effectiveness proposed method. We employed ERNIE language processing model complement Deeplab v3+ deep learning semantic segmentation extracting damage information media. A visualization analysis disaster-affected areas performed categorizing types. Additionally, Geodetector used investigate heterogeneity its underlying factors. approach allowed us analyze spatiotemporal patterns evolution, enabling rapid assessment facilitating emergency response efforts. The results show that method significantly enhances effectively identifying different types sensing data.
Language: Английский
Citations
1Automation in Construction, Journal Year: 2025, Volume and Issue: 174, P. 106151 - 106151
Published: March 31, 2025
Language: Английский
Citations
1Sustainable Cities and Society, Journal Year: 2024, Volume and Issue: 107, P. 105440 - 105440
Published: April 12, 2024
Language: Английский
Citations
8Sustainable Cities and Society, Journal Year: 2024, Volume and Issue: 104, P. 105321 - 105321
Published: Feb. 29, 2024
Language: Английский
Citations
7Sustainable Cities and Society, Journal Year: 2024, Volume and Issue: 106, P. 105362 - 105362
Published: March 20, 2024
Language: Английский
Citations
7Water, Journal Year: 2024, Volume and Issue: 16(17), P. 2476 - 2476
Published: Aug. 30, 2024
In the context of increasing frequency urban flooding disasters caused by extreme weather, accurate and timely identification monitoring flood risks have become increasingly important. This article begins with a bibliometric analysis literature on identification, revealing that since 2017, this area has global research hotspot. Subsequently, it presents systematic review current mainstream technologies, drawing from both traditional emerging data sources, which are categorized into sensor-based (including contact non-contact sensors) big data-based social media surveillance camera data). By analyzing advantages disadvantages each technology their different focuses, paper points out largely emphasizes more “intelligent” technologies. However, these technologies still certain limitations, sensor techniques retain significant in practical applications. Therefore, future risk should focus integrating multiple fully leveraging strengths sources to achieve real-time flooding.
Language: Английский
Citations
5