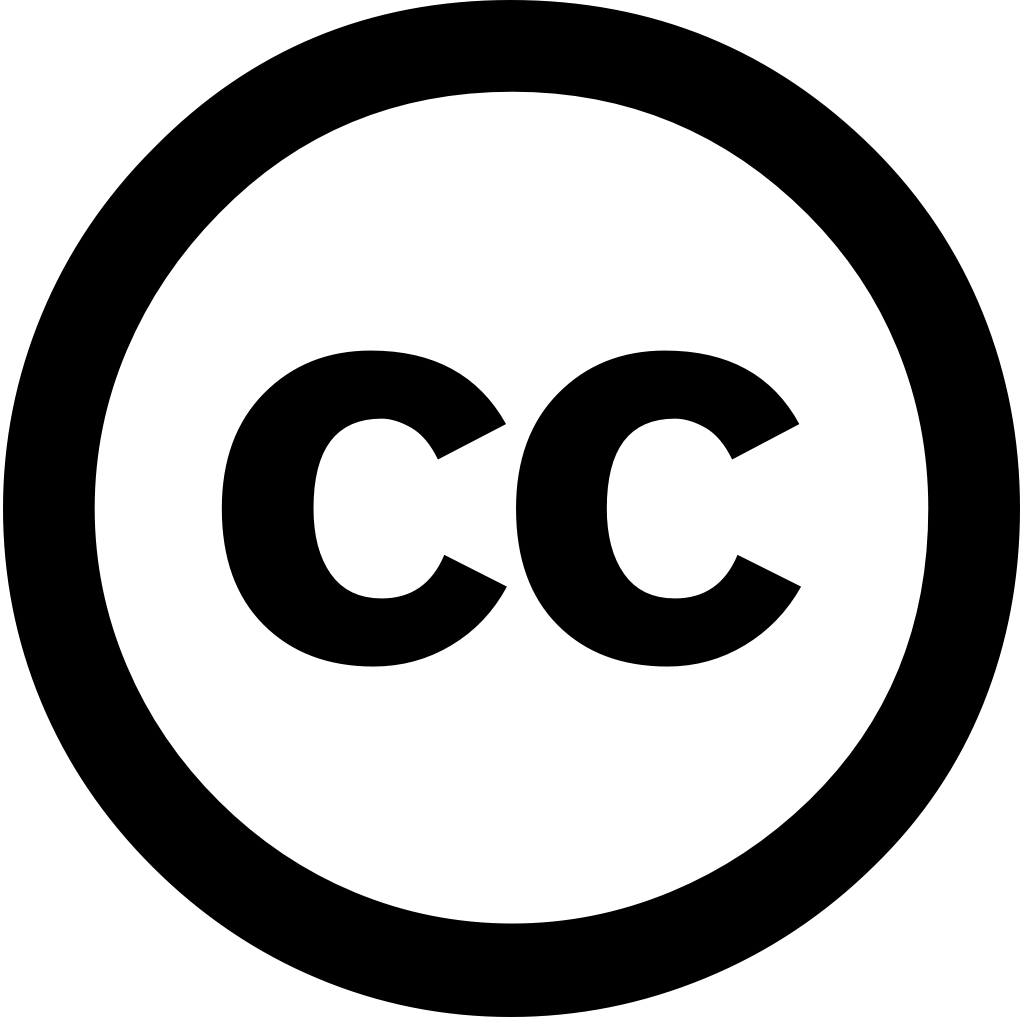
Measurement, Journal Year: 2024, Volume and Issue: unknown, P. 116477 - 116477
Published: Dec. 1, 2024
Language: Английский
Measurement, Journal Year: 2024, Volume and Issue: unknown, P. 116477 - 116477
Published: Dec. 1, 2024
Language: Английский
Automation in Construction, Journal Year: 2025, Volume and Issue: 171, P. 105973 - 105973
Published: Jan. 18, 2025
Language: Английский
Citations
1Transportation Geotechnics, Journal Year: 2024, Volume and Issue: unknown, P. 101409 - 101409
Published: Oct. 1, 2024
Language: Английский
Citations
4Automation in Construction, Journal Year: 2025, Volume and Issue: 171, P. 106011 - 106011
Published: Jan. 28, 2025
Language: Английский
Citations
0Engineering Science and Technology an International Journal, Journal Year: 2025, Volume and Issue: 63, P. 101957 - 101957
Published: Jan. 30, 2025
Language: Английский
Citations
0Journal of Computing in Civil Engineering, Journal Year: 2025, Volume and Issue: 39(3)
Published: Feb. 3, 2025
Language: Английский
Citations
0Journal of Manufacturing Systems, Journal Year: 2025, Volume and Issue: 79, P. 301 - 317
Published: Feb. 4, 2025
Language: Английский
Citations
0Tunnelling and Underground Space Technology, Journal Year: 2025, Volume and Issue: 162, P. 106607 - 106607
Published: April 14, 2025
Language: Английский
Citations
0Journal of Construction Engineering and Management, Journal Year: 2025, Volume and Issue: 151(6)
Published: April 15, 2025
Language: Английский
Citations
0AI in Civil Engineering, Journal Year: 2025, Volume and Issue: 4(1)
Published: May 1, 2025
Language: Английский
Citations
0Measurement Science and Technology, Journal Year: 2024, Volume and Issue: 35(8), P. 086211 - 086211
Published: May 21, 2024
Abstract Accurate prediction of shield attitude deviation is essential to ensure safe and efficient tunneling. However, previous studies have predominantly emphasized temporal correlation, which has limitations in engineering guidance accuracy. This research proposes a hybrid deep learning approach considering feature attention (FTA-N-GRU) for prediction. To elucidate the contributions each parameter, Integrated Gradients algorithm leveraged parameter sensitivity analysis. The results from Bangladesh Karnaphuli River Tunnel Project indicate that: proposed model outperforms other models integration can adaptively allocate weights input parameters, facilitating inexperienced operators discerning crucial variations decision-making. By incorporating attention, effectively explores connection among different output time steps, improving overall accuracy reliability. Consequently, are empowered with timely information proactively adjust operations before deviations occur, underscoring significance this promoting tunneling practices.
Language: Английский
Citations
1