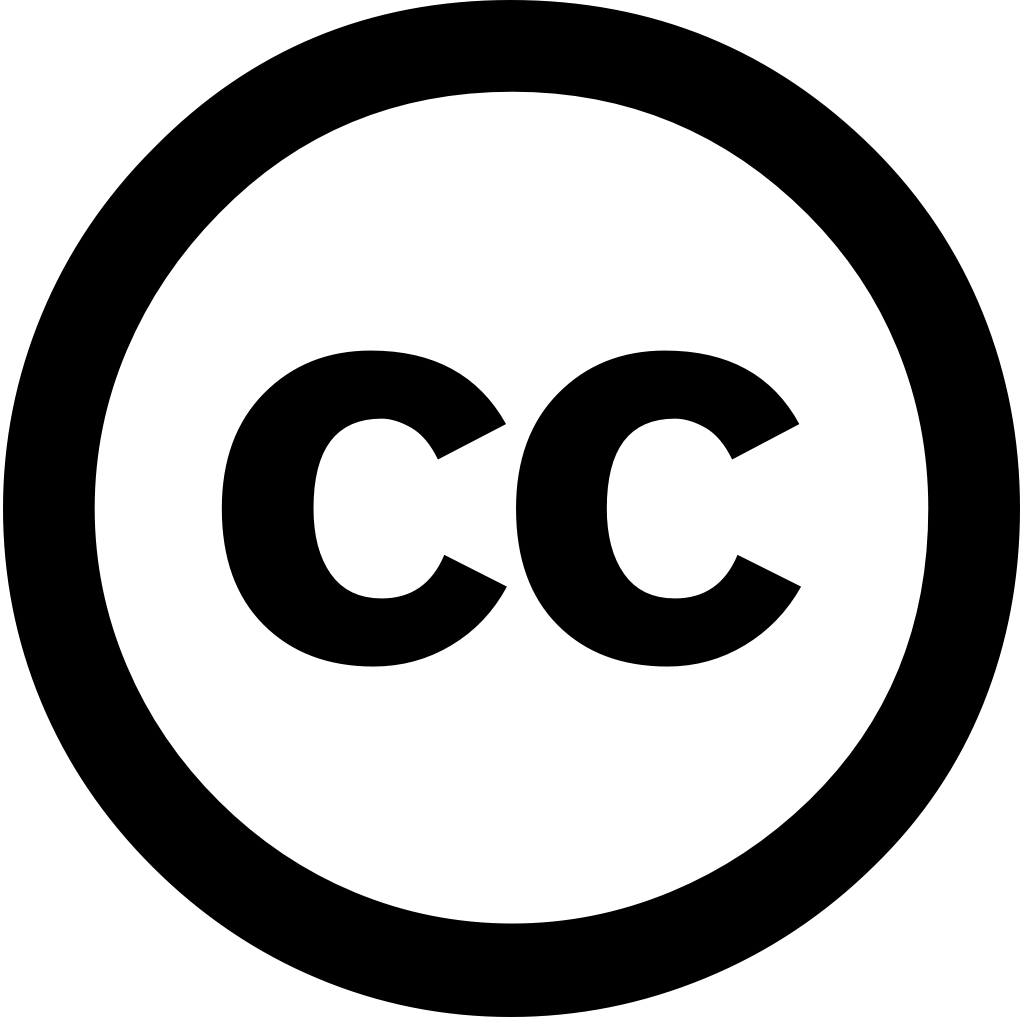
Journal of CO2 Utilization, Journal Year: 2024, Volume and Issue: 89, P. 102941 - 102941
Published: Oct. 21, 2024
Language: Английский
Journal of CO2 Utilization, Journal Year: 2024, Volume and Issue: 89, P. 102941 - 102941
Published: Oct. 21, 2024
Language: Английский
Energy and AI, Journal Year: 2024, Volume and Issue: 16, P. 100361 - 100361
Published: March 30, 2024
Coupled electrochemical systems for the direct capture and conversion of CO2 have garnered significant attention owing to their potential enhance energy- cost-efficiency by circumventing amine regeneration step. However, optimizing coupled system is more challenging than handling separated because its complexity, caused incorporation solvent heterogeneous catalysts. Nevertheless, deployment machine learning can be immensely beneficial, reducing both time cost ability simulate describe complex with numerous parameters involved. In this review, we summarized techniques employed in development solvents such as ionic liquids, well To optimize a system, these two separately developed will need combined via future.
Language: Английский
Citations
16Journal of Energy Storage, Journal Year: 2025, Volume and Issue: 111, P. 115363 - 115363
Published: Jan. 13, 2025
Language: Английский
Citations
2Energy, Journal Year: 2025, Volume and Issue: unknown, P. 135123 - 135123
Published: Feb. 1, 2025
Language: Английский
Citations
2Hybrid Advances, Journal Year: 2025, Volume and Issue: unknown, P. 100419 - 100419
Published: Feb. 1, 2025
Language: Английский
Citations
2Separation and Purification Technology, Journal Year: 2024, Volume and Issue: 354, P. 128794 - 128794
Published: July 14, 2024
Language: Английский
Citations
10Journal of Solid State Chemistry, Journal Year: 2025, Volume and Issue: unknown, P. 125174 - 125174
Published: Jan. 1, 2025
Language: Английский
Citations
1Advanced Science, Journal Year: 2025, Volume and Issue: unknown
Published: Jan. 4, 2025
Abstract Powder X‐ray diffraction (PXRD) is a prevalent technique in materials characterization. While the analysis of PXRD often requires extensive human manual intervention, and most automated method only achieved at coarse‐grained level. The more difficult important task fine‐grained crystal structure prediction from remains unaddressed. This study introduces XtalNet, first equivariant deep generative model for end‐to‐end PXRD. Unlike previous methods that rely solely on composition, XtalNet leverages as an additional condition, eliminating ambiguity enabling generation complex organic structures with up to 400 atoms unit cell. comprises two modules: Contrastive PXRD‐Crystal Pretraining (CPCP) module aligns space space, Conditional Crystal Structure Generation (CCSG) generates candidate conditioned patterns. Evaluation MOF datasets (hMOF‐100 hMOF‐400) demonstrates XtalNet's effectiveness. achieves top‐10 Match Rate 90.2% 79% hMOF‐100 hMOF‐400 conditional task, respectively. enables direct experimental measurements, need intervention external databases. opens new possibilities determination accelerated discovery novel materials.
Language: Английский
Citations
1Industrial & Engineering Chemistry Research, Journal Year: 2025, Volume and Issue: 64(9), P. 4637 - 4668
Published: Feb. 24, 2025
This review discusses the transformative impact of convergence artificial intelligence (AI) and laboratory automation on discovery synthesis metal–organic frameworks (MOFs). MOFs, known for their tunable structures extensive applications in fields such as energy storage, drug delivery, environmental remediation, pose significant challenges due to complex processes high structural diversity. Laboratory has streamlined repetitive tasks, enabled high-throughput screening reaction conditions, accelerated optimization protocols. The integration AI, particularly Transformers large language models (LLMs), further revolutionized MOF research by analyzing massive data sets, predicting material properties, guiding experimental design. emergence self-driving laboratories (SDLs), where AI-driven decision-making is coupled with automated experimentation, represents next frontier research. While remain fully realizing potential this synergistic approach, AI heralds a new era efficiency innovation engineering materials.
Language: Английский
Citations
1JACS Au, Journal Year: 2024, Volume and Issue: 4(10), P. 3727 - 3743
Published: Sept. 12, 2024
Renowned for their high porosity and structural diversity, metal-organic frameworks (MOFs) are a promising class of materials wide range applications. In recent decades, with the development large-scale databases, MOF community has witnessed innovations brought by data-driven machine learning methods, which have enabled deeper understanding chemical nature MOFs led to novel structures. Notably, is continuously rapidly advancing as new methodologies, architectures, data representations actively being investigated, implementation in discovery vigorously pursued. Under these circumstances, it important closely monitor research trends identify technologies that introduced. this Perspective, we focus on emerging within field MOFs, challenges they face, future directions development.
Language: Английский
Citations
8The Journal of Physical Chemistry C, Journal Year: 2024, Volume and Issue: 128(24), P. 10202 - 10215
Published: June 5, 2024
In this article, we propose simple, interpretable machine learning (ML) features aimed at improving the accuracy of ML models in predicting adsorption gases, such as methane, ethane, propane, krypton, and xenon, metal–organic frameworks (MOFs). particular, introduce energy-based that incorporate surface energy histograms radial distribution functions. These effectively capture spatial variations interaction energy, thereby predictive capabilities models. We further extend approach to predict carbon dioxide nitrogen a diverse set MOFs under ambient conditions by including Coulombic component proposed features. The final model combines with geometric characteristics Henry's law constants. This integration results significantly increased models, especially within moderate pressure regime, when compared previous studies. Using CoRE MOF database for training testing, our achieves prediction R2 > 0.96 xenon various conditions, 0.80 dioxide, 0.97 nitrogen. CRAFTED simulated isotherms nitrogen, demonstrate robustness single-component specific temperature. test, 0.87 0.90 physics-based nature allows us provide some interpretation why predictions are better materials than others. According interpretation, an accurate representation shape potential plays important role capturing process adsorption. Finally, acknowledge certain limitations current oversimplification representing geometry interactions molecules polar interactions.
Language: Английский
Citations
7