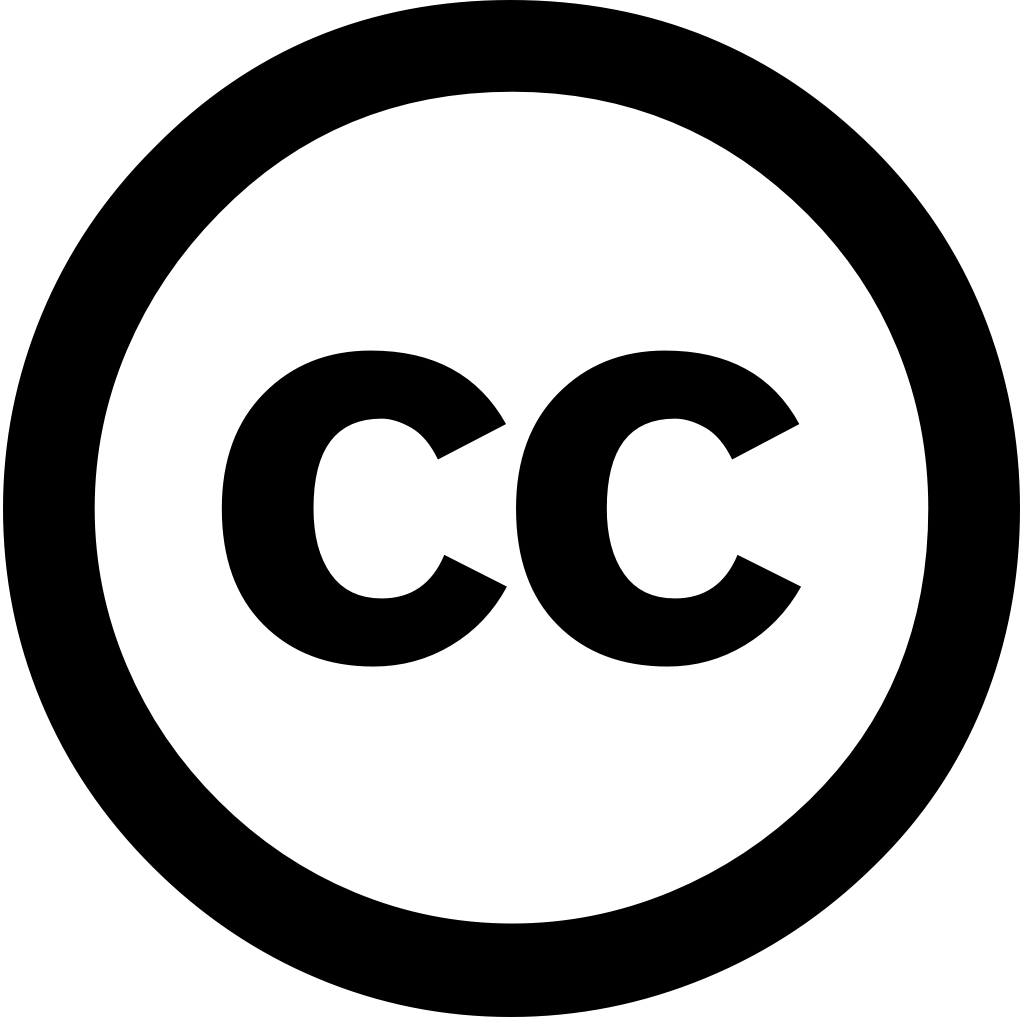
Neuron, Journal Year: 2023, Volume and Issue: 111(7), P. 1020 - 1036
Published: April 1, 2023
Language: Английский
Neuron, Journal Year: 2023, Volume and Issue: 111(7), P. 1020 - 1036
Published: April 1, 2023
Language: Английский
Nature Reviews Molecular Cell Biology, Journal Year: 2021, Volume and Issue: 23(1), P. 40 - 55
Published: Sept. 13, 2021
Language: Английский
Citations
1277Annual Review of Neuroscience, Journal Year: 2020, Volume and Issue: 43(1), P. 249 - 275
Published: July 8, 2020
Significant experimental, computational, and theoretical work has identified rich structure within the coordinated activity of interconnected neural populations. An emerging challenge now is to uncover nature associated computations, how they are implemented, what role play in driving behavior. We term this computation through population dynamics. If successful, framework will reveal general motifs quantitatively describe dynamics implement computations necessary for goal-directed Here, we start with a mathematical primer on dynamical systems theory analytical tools apply perspective experimental data. Next, highlight some recent discoveries resulting from successful application systems. focus studies spanning motor control, timing, decision-making, working memory. Finally, briefly discuss promising lines investigation future directions framework.
Language: Английский
Citations
530Nature Neuroscience, Journal Year: 2020, Volume and Issue: 23(2), P. 260 - 270
Published: Jan. 6, 2020
Language: Английский
Citations
339Neuron, Journal Year: 2020, Volume and Issue: 107(6), P. 1048 - 1070
Published: Sept. 1, 2020
Language: Английский
Citations
295Neuron, Journal Year: 2018, Volume and Issue: 98(6), P. 1099 - 1115.e8
Published: June 1, 2018
Language: Английский
Citations
293Current Opinion in Neurobiology, Journal Year: 2019, Volume and Issue: 55, P. 103 - 111
Published: March 13, 2019
Language: Английский
Citations
279Nature, Journal Year: 2019, Volume and Issue: 577(7790), P. 386 - 391
Published: Dec. 25, 2019
Language: Английский
Citations
277Neuron, Journal Year: 2019, Volume and Issue: 103(2), P. 292 - 308.e4
Published: June 3, 2019
Language: Английский
Citations
241Neuron, Journal Year: 2019, Volume and Issue: 103(5), P. 934 - 947.e5
Published: July 15, 2019
Language: Английский
Citations
224eLife, Journal Year: 2020, Volume and Issue: 9
Published: Sept. 17, 2020
Mechanistic modeling in neuroscience aims to explain observed phenomena terms of underlying causes. However, determining which model parameters agree with complex and stochastic neural data presents a significant challenge. We address this challenge machine learning tool uses deep density estimators—trained using simulations—to carry out Bayesian inference retrieve the full space compatible raw or selected features. Our method is scalable features can rapidly analyze new after initial training. demonstrate power flexibility our approach on receptive fields, ion channels, Hodgkin–Huxley models. also characterize circuit configurations giving rise rhythmic activity crustacean stomatogastric ganglion, use these results derive hypotheses for compensation mechanisms. will help close gap between data-driven theory-driven models dynamics.
Language: Английский
Citations
207