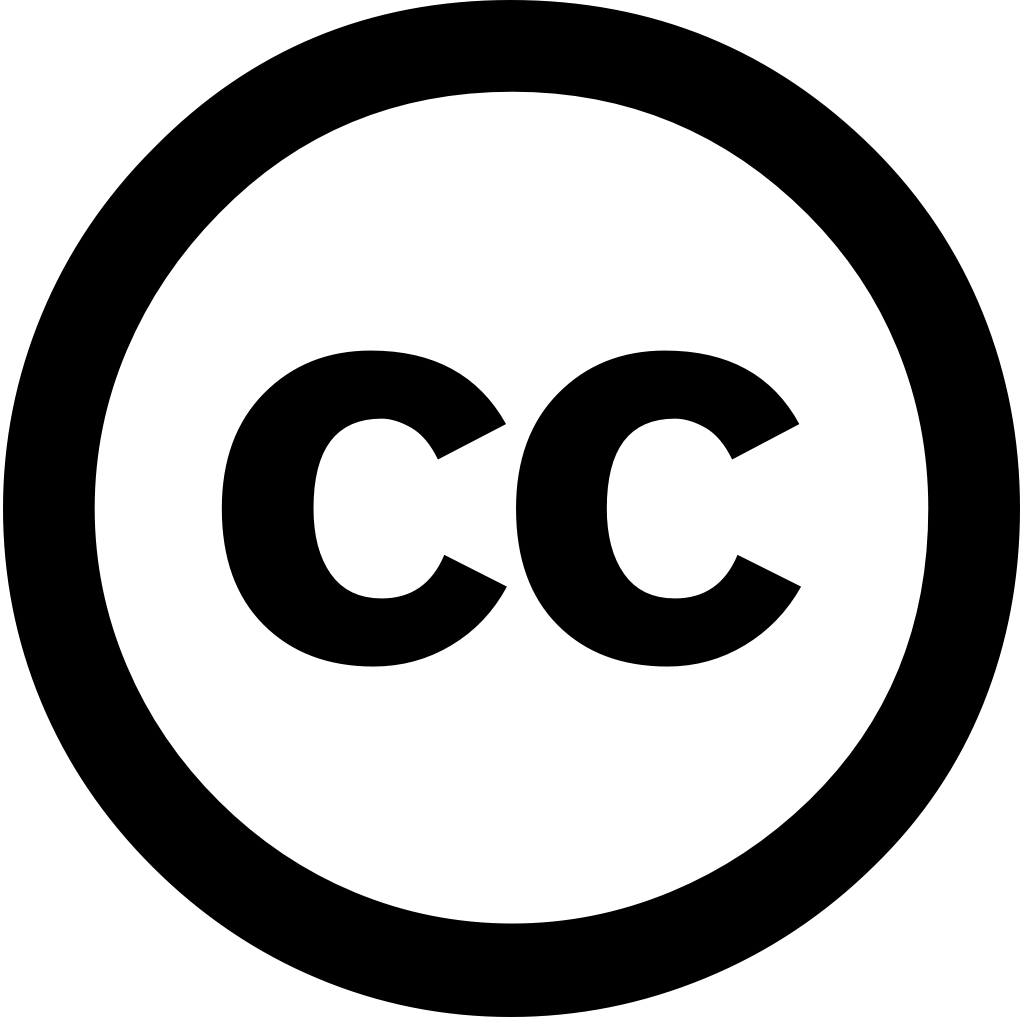
Molecular & Cellular Proteomics, Journal Year: 2025, Volume and Issue: unknown, P. 100917 - 100917
Published: Jan. 1, 2025
Language: Английский
Molecular & Cellular Proteomics, Journal Year: 2025, Volume and Issue: unknown, P. 100917 - 100917
Published: Jan. 1, 2025
Language: Английский
Nucleic Acids Research, Journal Year: 2024, Volume and Issue: 53(D1), P. D609 - D617
Published: Nov. 18, 2024
The aim of the UniProt Knowledgebase (UniProtKB; https://www.uniprot.org/) is to provide users with a comprehensive, high-quality and freely accessible set protein sequences annotated functional information. In this publication, we describe ongoing changes our production pipeline limit available in UniProtKB high-quality, non-redundant reference proteomes. We continue manually curate scientific literature add latest data use machine learning techniques. also encourage community curation ensure key publications are not missed. an update on automatic annotation methods used by predict information for unreviewed entries describing unstudied proteins. Finally, updates website described, including new tab linking genomic recognition its value community, database has been awarded Global Core Biodata Resource status.
Language: Английский
Citations
159Nucleic Acids Research, Journal Year: 2024, Volume and Issue: 53(D1), P. D20 - D29
Published: Nov. 11, 2024
The National Center for Biotechnology Information (NCBI) provides online information resources biology, including the GenBank® nucleic acid sequence repository and PubMed® of citations abstracts published in life science journals. NCBI search retrieval operations most these data from 31 distinct repositories knowledgebases. E-utilities serve as programming interface these. Resources receiving significant updates past year include PubMed, PubMed Central, Bookshelf, NIH Comparative Genomics Resource, BLAST, Sequence Read Archive, Taxonomy, iCn3D, Conserved Domain Database, Pathogen Detection, antimicrobial resistance PubChem. These can be accessed through home page at https://www.ncbi.nlm.nih.gov.
Language: Английский
Citations
17Nature Microbiology, Journal Year: 2025, Volume and Issue: 10(1), P. 20 - 27
Published: Jan. 2, 2025
Language: Английский
Citations
2Nucleic Acids Research, Journal Year: 2024, Volume and Issue: 53(D1), P. D30 - D44
Published: Nov. 11, 2024
The National Genomics Data Center (NGDC), which is a part of the China for Bioinformation (CNCB), offers comprehensive suite database resources to support global scientific community. Amidst unprecedented accumulation multi-omics data, CNCB-NGDC committed continually evolving and updating its core through big data archiving, integrative analysis value-added curation. Over past year, has expanded collaborations with international databases established new subcenters focusing on biodiversity, traditional Chinese medicine tumor genetics. Substantial efforts have been made toward encompassing broad spectrum developing innovative enhancing existing resources. Notably, developed single-cell omics (scTWAS Atlas), genome variation (VDGE), health disease (CVD Atlas, CPMKG, Immunosenescence Inventory, HemAtlas, Cyclicpepedia, IDeAS), biodiversity biosynthesis (RefMetaPlant, MASH-Ocean) research tools (CCLHunter). All services are publicly accessible at https://ngdc.cncb.ac.cn.
Language: Английский
Citations
14Nucleic Acids Research, Journal Year: 2024, Volume and Issue: 52(W1), P. W287 - W293
Published: May 15, 2024
The PSIRED Workbench is a long established and popular bioinformatics web service offering wide range of machine learning based analyses for characterizing protein structure function. In this paper we provide an update the recent additions developments to webserver, with focus on new Deep Learning methods. We briefly discuss some trends in server usage since publication AlphaFold2 give overview upcoming service. PSIPRED available at http://bioinf.cs.ucl.ac.uk/psipred.
Language: Английский
Citations
10Cell, Journal Year: 2024, Volume and Issue: unknown
Published: Dec. 1, 2024
Language: Английский
Citations
9bioRxiv (Cold Spring Harbor Laboratory), Journal Year: 2025, Volume and Issue: unknown
Published: Jan. 4, 2025
Microbiology research has historically focused on a few species of model organisms. Our bibliographic analysis finds extreme bias in the distribution bacteriology across species, with half all papers referencing only ten and 74% known remaining unstudied. Microbiologists will need to broaden their perspective embrace complexity develop complete understanding microbial world.
Language: Английский
Citations
1Advanced Science, Journal Year: 2025, Volume and Issue: unknown
Published: Feb. 3, 2025
Abstract Unexpected toxicity has become a significant obstacle to drug candidate development, accounting for 30% of discovery failures. Traditional assessment through animal testing is costly and time‐consuming. Big data artificial intelligence (AI), especially machine learning (ML), are robustly contributing innovation progress in toxicology research. However, the optimal AI model different types usually varies, making it essential conduct comparative analyses methods across domains. The diverse sources also pose challenges researchers focusing on specific studies. In this review, 10 categories drug‐induced examined, summarizing characteristics applicable ML models, including both predictive interpretable algorithms, striking balance between breadth depth. Key databases tools used prediction highlighted, toxicology, chemical, multi‐omics, benchmark databases, organized by their focus function clarify roles prediction. Finally, strategies turn into opportunities analyzed discussed. This review may provide with valuable reference understanding utilizing available resources bridge mechanistic insights, further advance application drugs‐induced
Language: Английский
Citations
1New Zealand Veterinary Journal, Journal Year: 2025, Volume and Issue: unknown, P. 1 - 6
Published: Feb. 4, 2025
Case history A gland of a cow with high somatic cell count (385,000 cells/mL at 116 days in milk) was enrolled therapeutic subclinical mastitis study following positive pre-enrolment culture. One week later, the quarter treated 1 g procaine penicillin on three occasions, 12 hours apart (Day 0 and 1). further milk sample obtained from Days 6, 14, 21 after initiation treatment.
Language: Английский
Citations
1Journal of King Saud University - Computer and Information Sciences, Journal Year: 2024, Volume and Issue: 36(7), P. 102126 - 102126
Published: July 14, 2024
Corn diseases significantly impact crop yields, posing a major challenge to agricultural productivity. Early and accurate detection of these is crucial for effective management mitigation. Existing methods, mostly relying on analyzing corn leaves, often lack the precision identify classify wide range under varying conditions. This study introduces novel approach detecting using image processing deep learning techniques, aiming enhance accuracy through pre-processing, improved feature extraction selection, classification algorithms. A new Convolutional Neural Network (CNN) model named TreeNet, with 35 layers 38 connections, proposed. TreeNet pre-trained Plant Village imaging dataset. For YCbCr color space utilized improve representation contrast. Feature performed two models, Darknet-53, DenseNet-201, features fused serial-based fusion method. The Entropy-coded Sine Cosine Algorithm applied optimizing set classification. selected are used train Support Vector Machine (SVM) K-Nearest Neighbor (KNN) classifiers, extensive experiments conducted both 5-fold 10-fold cross-validation, sizes ranging from 200 1150. proposed method achieves accuracy, precision, recall, F1-score 99.8%, 99%, 100%, respectively, surpassing existing benchmarks. integration Darknet-53 along robust pre-processing improves disease detection, highlighting potential advanced CNN architectures in agriculture.
Language: Английский
Citations
8