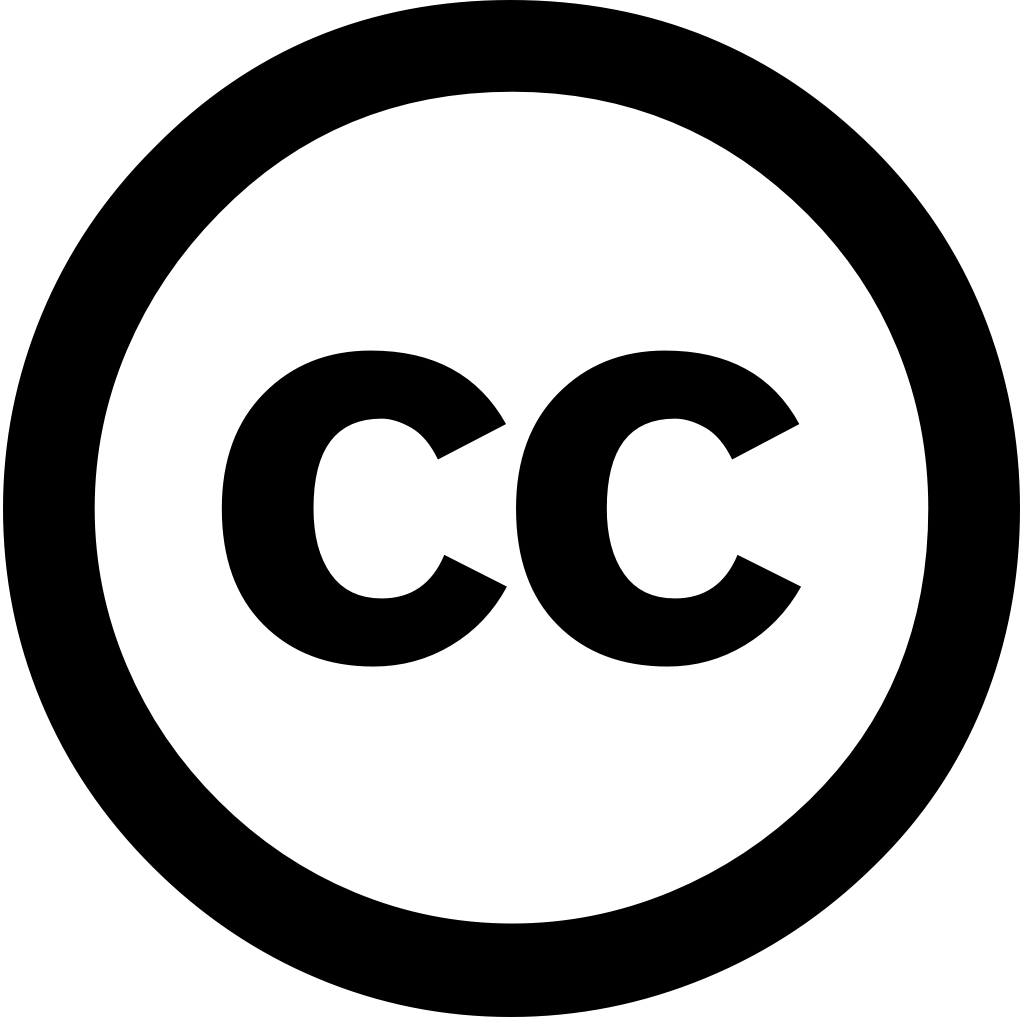
International Journal of Data Science and Analytics, Journal Year: 2025, Volume and Issue: unknown
Published: April 17, 2025
Language: Английский
International Journal of Data Science and Analytics, Journal Year: 2025, Volume and Issue: unknown
Published: April 17, 2025
Language: Английский
Diagnostics, Journal Year: 2023, Volume and Issue: 13(12), P. 2038 - 2038
Published: June 12, 2023
In public health, machine learning algorithms have been used to predict or diagnose chronic epidemiological disorders such as diabetes mellitus, which has reached epidemic proportions due its widespread occurrence around the world. Diabetes is just one of several diseases for techniques can be in diagnosis, prognosis, and assessment procedures.In this paper, we propose a new approach boosting classification based on metaheuristic optimization algorithm. The proposed proposes feature selection algorithm dynamic Al-Biruni earth radius dipper-throated (DBERDTO). selected features are then classified using random forest classifier with parameters optimized DBERDTO.The methodology evaluated compared recent methods models prove efficiency superiority. overall accuracy achieved by 98.6%. On other hand, statistical tests conducted assess significance difference analysis variance (ANOVA) Wilcoxon signed-rank tests.The results these confirmed superiority methods.
Language: Английский
Citations
23Frontiers in Energy Research, Journal Year: 2023, Volume and Issue: 11
Published: June 1, 2023
Accurate forecasting of wind speed is crucial for power systems stability. Many machine learning models have been developed to forecast accurately. However, the accuracy these still needs more improvements achieve accurate results. In this paper, an optimized model proposed boosting prediction speed. The optimization performed in terms a new algorithm based on dipper-throated (DTO) and genetic (GA), which referred as (GADTO). used optimize bidrectional long short-term memory (BiLSTM) parameters. To verify effectiveness methodology, benchmark dataset freely available Kaggle employed conducted experiments. first preprocessed be prepared further processing. addition, feature selection applied select significant features using binary version GADTO algorithm. selected are utilized learn best configuration BiLSTM model. predict future values speed, resulting predictions analyzed set evaluation criteria. Moreover, statistical test study difference approach compared other approaches analysis variance (ANOVA) Wilcoxon signed-rank tests. results tests confirmed approach’s its robustness with average root mean square error (RMSE) 0.00046, outperforms performance recent methods.
Language: Английский
Citations
22Biomimetics, Journal Year: 2023, Volume and Issue: 8(3), P. 313 - 313
Published: July 16, 2023
The virus that causes monkeypox has been observed in Africa for several years, and it linked to the development of skin lesions. Public panic anxiety have resulted from deadly repercussions infections following COVID-19 pandemic. Rapid detection approaches are crucial since reached a pandemic level. This study's overarching goal is use metaheuristic optimization boost performance feature selection classification methods identify lesions as indicators event Deep learning transfer used extract necessary features. GoogLeNet network deep framework extraction. In addition, binary implementation dipper throated (DTO) algorithm selection. decision tree classifier then label selected set optimized using continuous version DTO improve accuracy. Various evaluation compare contrast proposed approach other competing metrics: accuracy, sensitivity, specificity,
Language: Английский
Citations
21Alexandria Engineering Journal, Journal Year: 2023, Volume and Issue: 80, P. 383 - 396
Published: Sept. 1, 2023
In recent years, improvements in wireless communication have led to the development of microstrip or patch antennas. The article discusses using simulation, measurement, an RLC equivalent circuit model, and machine learning assess antenna performance. antenna's dimensions are 1.01 λ0×0.612λ0 with respect lowest operating frequency, maximum achieved gain is 6.76 dB, directivity 8.21 dBi, efficiency 83.05%. prototype's measured return loss compared CST ADS simulations. prediction determined a different supervised regression (ML) method. performance ML models by variance score, R square, mean square error (MSE), absolute (MAE), root (RMSE), squared logarithmic (MSLE), etc. With errors less than unity accuracy roughly 98%, Ridge outperforms other seven models. Gaussian process best method for predicting directivity. Finally, modeling results from ADS, as well anticipated learning, reveal that suggested good candidate LTE.
Language: Английский
Citations
21IEEE Access, Journal Year: 2023, Volume and Issue: 11, P. 92505 - 92522
Published: Jan. 1, 2023
This paper provides a novel meta-heuristic optimization algorithm for solving continuous problems efficiently in the field of numerical and engineering optimization: Piranha Foraging Optimization Algorithm (PFOA). The is inspired by flexible mobile foraging behaviour piranha swarm divides their behavior into three patterns: localized group attack, bloodthirsty cluster attack scavenging foraging, simulates above behaviors to construct two dynamic search processes exploration exploitation. PFOA uses strategies non-linear parameter control, population survival reverse evasion enable populations have better diversity at different stages help find solutions. To gain insight performance PFOA, visualization methods were used assess efficiency analyse impact characteristics modes, sensitivity parameters size on algorithm. was further tested with 27 CEC benchmark functions four real design problems, results compared 13 well-known meta-heuristics. Test based statistical such as box-line plots, Wilcoxon rank sum test Friedman multiple dimensions (30, 50, 100 fixed dimensions) show significant differences other algorithms that stable improvement. unique advantages terms equilibrium convergence speed can avoid getting trapped local optimum regions effectively solve complex spaces.
Language: Английский
Citations
20Biomimetics, Journal Year: 2023, Volume and Issue: 8(3), P. 270 - 270
Published: June 26, 2023
Breast cancer is one of the most common cancers in women, with an estimated 287,850 new cases identified 2022. There were 43,250 female deaths attributed to this malignancy. The high death rate associated type can be reduced early detection. Nonetheless, a skilled professional always necessary manually diagnose malignancy from mammography images. Many researchers have proposed several approaches based on artificial intelligence. However, they still face obstacles, such as overlapping cancerous and noncancerous regions, extracting irrelevant features, inadequate training models. In paper, we developed novel computationally automated biological mechanism for categorizing breast cancer. Using optimization approach Advanced Al-Biruni Earth Radius (ABER) algorithm, boosting classification realized. stages framework include data augmentation, feature extraction using AlexNet transfer learning, optimized convolutional neural network (CNN). learning CNN improved accuracy when results are compared recent approaches. Two publicly available datasets utilized evaluate framework, average 97.95%. To ensure statistical significance difference between methodology, additional tests conducted, analysis variance (ANOVA) Wilcoxon, addition evaluating various metrics. these emphasized effectiveness methodology current methods.
Language: Английский
Citations
19Sustainability, Journal Year: 2022, Volume and Issue: 15(1), P. 757 - 757
Published: Dec. 31, 2022
Forecasting is defined as the process of estimating change in uncertain situations. One most vital aspects many applications temperature forecasting. Using Daily Delhi Climate Dataset, we utilize time series forecasting techniques to examine predictability temperature. In this paper, a hybrid model based on combination Wavelet Decomposition (WD) and Seasonal Auto-Regressive Integrated Moving Average with Exogenous Variables (SARIMAX) was created accomplish accurate for Delhi, India. The range dataset from 2013 2017. It consists 1462 instances four features, 80% data used training 20% testing. First, WD decomposes non-stationary into multi-dimensional components. That can reduce original series’ volatility increase its stability. After that, components are inputs SARIMAX forecast City. employed work has following order: (4, 0, 1). [1], 12). experimental results demonstrated that WD-SARIMAX performs better than other recent models city. Mean Square Error (MSE), Absolute (MAE), Median (MedAE), Root (RMSE), Percentage (MAPE), determination coefficient (R2) proposed 2.8, 1.13, 0.76, 1.67, 4.9, 0.91, respectively. Furthermore, utilized over next eight years, 2017 2025.
Language: Английский
Citations
27Frontiers in Energy Research, Journal Year: 2023, Volume and Issue: 11
Published: June 29, 2023
Solar-powered water electrolysis can produce clean hydrogen for sustainable energy systems. Accurate solar generation forecasts are necessary system operation and planning. Al-Biruni Earth Radius (BER) Particle Swarm Optimization (PSO) used in this paper to ensemble forecast generation. The suggested method optimizes the dynamic hyperparameters of deep learning model recurrent neural network (RNN) using BER metaheuristic search optimization algorithm PSO algorithm. We data from HI-SEAS weather station Hawaii 4 months (September through December 2016). will level production next season our simulations compare results those other forecasting approaches. Regarding accuracy, resilience, computational economy, show that BER-PSO-RNN has great potential as a useful tool generation, which important ramifications planning execution such accuracy proposed is confirmed by two statistical analysis tests, Wilcoxon’s rank-sum one-way variance (ANOVA). With use excels processing time-series data, we discovered with algorithm, Solar System could produce, on average, 0.622 kg/day during comparison algorithms.
Language: Английский
Citations
16Energies, Journal Year: 2023, Volume and Issue: 16(3), P. 1370 - 1370
Published: Jan. 28, 2023
Introduction: Wind speed and solar radiation are two of the most well-known widely used renewable energy sources worldwide. Coal, natural gas, petroleum examples fossil fuels that not replenished thus non-renewable due to their high carbon content methods by which they generated. To predict production sources, researchers use forecasting techniques based on recent advances in machine learning approaches. Numerous prediction have significant drawbacks, including computational complexity inability generalize for various types sources. Methodology: In this paper, we proposed a novel approach capable generalizing accuracy both wind data. The is new optimization algorithm stacked ensemble model. hybrid Al-Biruni Earth Radius (BER) genetic (GA) it denoted GABER algorithm. This optimize parameters model boost improve generalization capability. Results: evaluate approach, several experiments conducted study its effectiveness superiority compared other models. addition, statistical tests assess significance difference approach. recorded results proved approach’s superiority, effectiveness, generalization, when state-of-the-art methods. Conclusions: predicting with better generalization.
Language: Английский
Citations
15Symmetry, Journal Year: 2022, Volume and Issue: 14(11), P. 2323 - 2323
Published: Nov. 5, 2022
Convolutional neural networks (CNNs) have exhibited significant performance gains over conventional machine learning techniques in solving various real-life problems computational intelligence fields, such as image classification. However, most existing CNN architectures were handcrafted from scratch and required amounts of problem domain knowledge designers. A novel deep method abbreviated TLBOCNN is proposed this paper by leveraging the excellent global search ability teaching–learning-based optimization (TLBO) to obtain an optimal design network architecture for a based on given dataset with symmetrical distribution each class data samples. variable-length encoding scheme first introduced represent learner potential different layer parameters. During teacher phase, new mainstream computation designed compute mean parameter values considering information encoded into population members variable lengths. The mechanisms determining differences between two learners lengths updating their positions are also devised both phases learners. Extensive simulation studies report that achieves classifying majority MNIST-variant datasets, displays highest accuracy, produces models lowest complexity levels compared other state-of-the-art methods due its promising ability.
Language: Английский
Citations
17