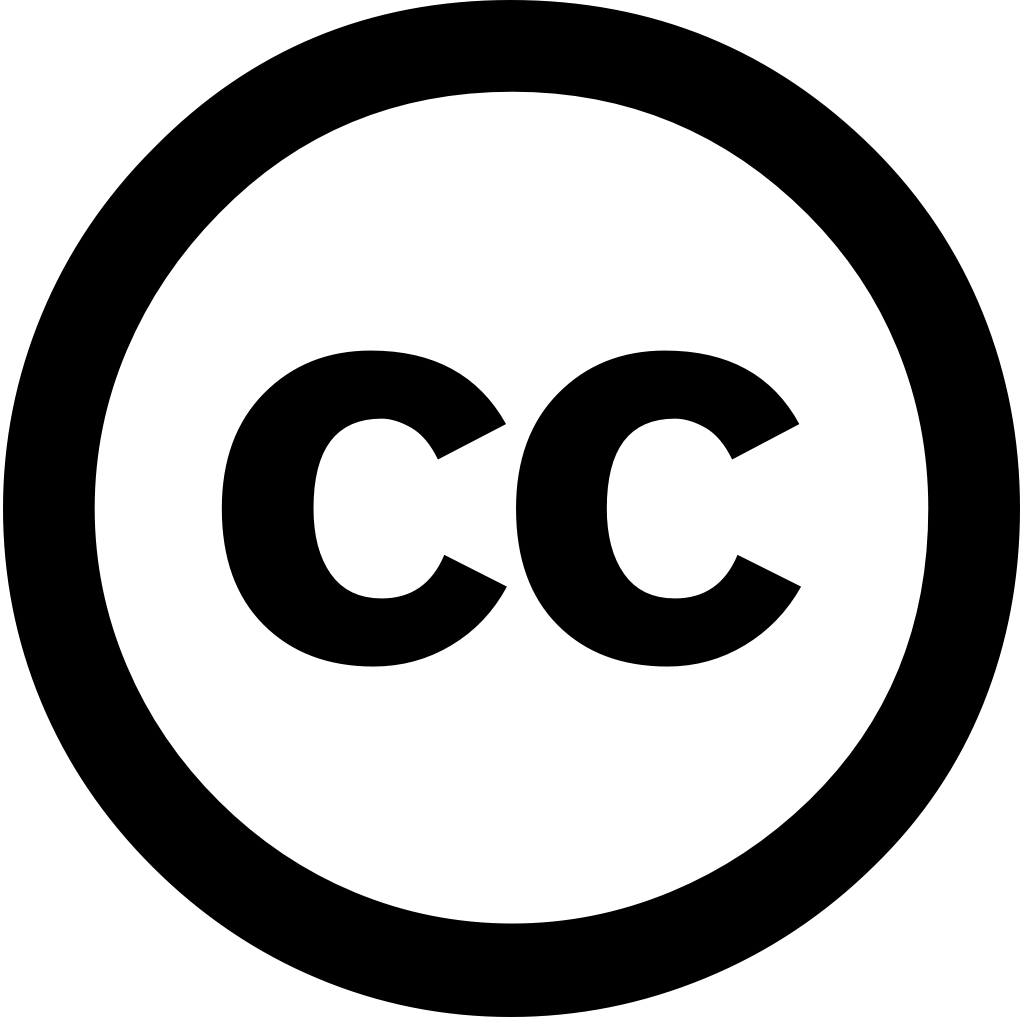
IEEE Access, Journal Year: 2023, Volume and Issue: 11, P. 112953 - 112972
Published: Jan. 1, 2023
In
the
advanced
metering
infrastructure
(AMI)
of
smart
grid,
meters
(SMs)
are
deployed
to
collect
fine-grained
electricity
consumption
data,
enabling
billing,
load
monitoring,
and
efficient
energy
management.
However,
some
consumers
engage
in
fraudulent
behavior
by
hacking
their
meters,
leading
either
traditional
theft
or
more
sophisticated
evasion
attacks
(EAs).
EAs
aim
illegally
reduce
bills
while
deceiving
detection
mechanisms.
The
current
methods
for
identifying
such
raise
privacy
concerns
due
need
access
consumers'
detailed
data
train
To
address
concerns,
federated
learning
(FL)
is
proposed
as
a
collaborative
training
approach
across
multiple
consumers.
Adversarial
(AT)
has
shown
promise
countering
threats
on
machine
models.
This
paper,
first,
investigates
susceptibility
classifiers
trained
FL
both
independent
identically
distributed
(IID)
Non-IID
data.
Then,
it
effectiveness
AT
securing
global
detector
against
EAs,
assuming
no
misbehavior
from
participant
process.
After
that,
we
introduce
three
novel
attacks,
namely
Language: Английский