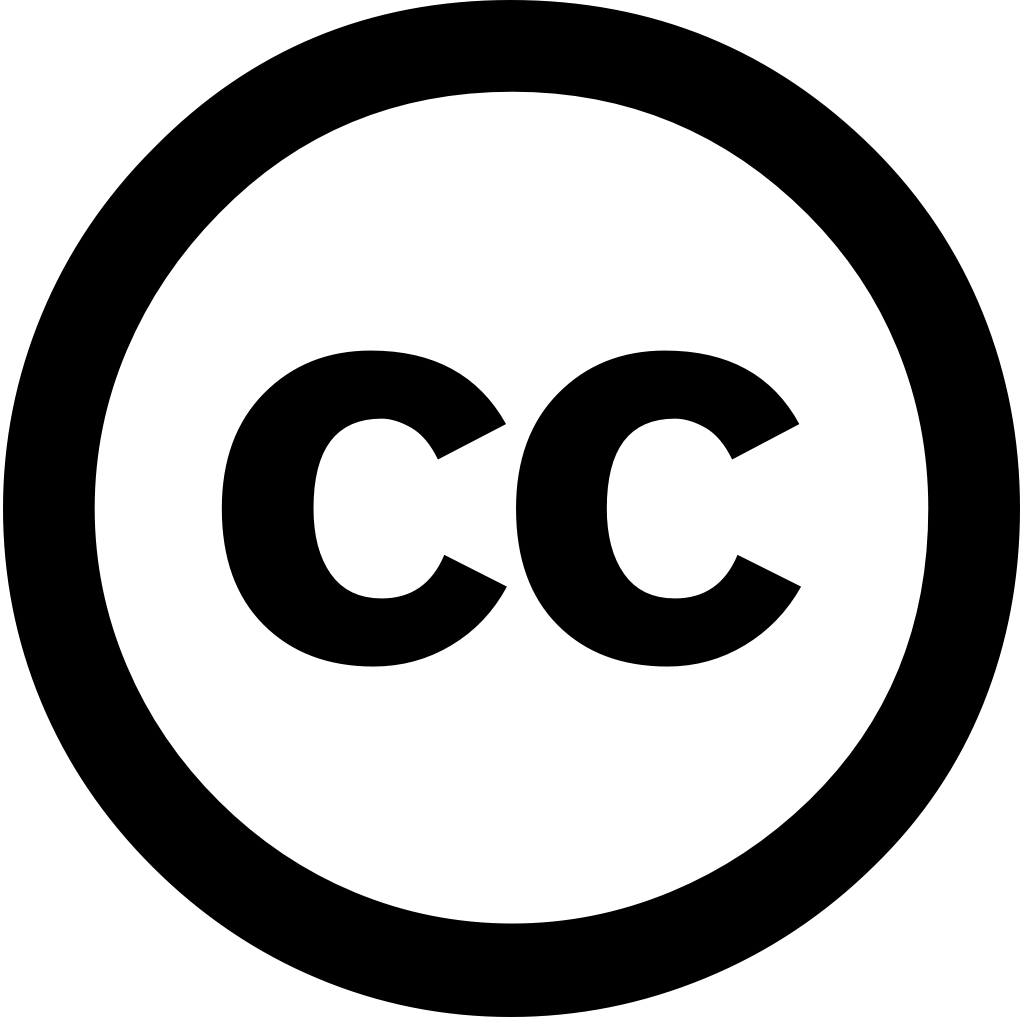
IEEE Access, Journal Year: 2024, Volume and Issue: 12, P. 112095 - 112115
Published: Jan. 1, 2024
Language: Английский
IEEE Access, Journal Year: 2024, Volume and Issue: 12, P. 112095 - 112115
Published: Jan. 1, 2024
Language: Английский
Applied Soft Computing, Journal Year: 2024, Volume and Issue: 159, P. 111650 - 111650
Published: April 30, 2024
Language: Английский
Citations
3Structures, Journal Year: 2023, Volume and Issue: 58, P. 105409 - 105409
Published: Oct. 31, 2023
Language: Английский
Citations
5Future Internet, Journal Year: 2024, Volume and Issue: 16(4), P. 124 - 124
Published: April 7, 2024
The objective of task allocation in unmanned systems is to complete tasks at minimal costs. However, the current algorithms employed for coordinating multiple frequently converge local optima, thus impeding identification best solutions. To address these challenges, this study builds upon sheep flock optimization algorithm (SFOA) by preserving individuals eliminated during iterative process within a prior knowledge set, which continuously updated. During reproduction phase algorithm, utilized guide generation new individuals, preventing their rapid reconvergence optima. This approach aids reducing frequency converges continually steering towards global optimum and thereby enhancing efficiency allocation. Finally, various scenarios are presented evaluate performances algorithms. results show that proposed paper more likely than other escape from optima find optimum.
Language: Английский
Citations
1Water, Journal Year: 2024, Volume and Issue: 16(8), P. 1134 - 1134
Published: April 16, 2024
Wireless sensor networks have gained significant attention in recent years due to their wide range of applications environmental monitoring, surveillance, and other fields. The design a groundwater quality quantity monitoring network is an important aspect aquifer restoration the prevention pollution overexploitation. Moreover, development novel localization strategy project wireless aims address challenge optimizing location relation process so as extract maximum information with minimum cost. In this study, improved hybrid butterfly artificial gorilla troop optimizer (iHBAGTO) technique applied optimize nodes’ position analysis path loss delay, RSS calculated. Butterfly Artificial Intelligence used multi-functional derivation convergence rate produce designed data localization. proposed iHBAGTO algorithm demonstrated highest 99.6%, it achieved lowest average error 4.8; consistently had delay 13.3 ms for all iteration counts, has values 8.2 dB, energy consumption value 0.01 J, received signal strength 86% counts. Overall, Proposed outperforms algorithms.
Language: Английский
Citations
1Evolutionary Intelligence, Journal Year: 2024, Volume and Issue: 17(5-6), P. 3337 - 3440
Published: May 8, 2024
Language: Английский
Citations
1Journal of Intelligent & Fuzzy Systems, Journal Year: 2024, Volume and Issue: 46(2), P. 4361 - 4380
Published: Feb. 9, 2024
The Aquila optimization (AO) algorithm has the drawbacks of local and poor accuracy when confronted with complex problems. To remedy these drawbacks, this paper proposes an Enhanced aquila (EAO) algorithm. avoid elite individual from entering optima, opposition-based learning strategy is added. enhance ability balancing global exploration exploitation, a dynamic boundary introduced. elevate algorithm’s convergence rapidity precision, retention mechanism effectiveness EAO evaluated using CEC2005 benchmark functions four images. experimental results confirm EAO’s viability efficacy. statistical Freidman test Wilcoxon rank sum are confirmed robustness. proposed outperforms previous algorithms can useful for threshold pressure vessel design.
Language: Английский
Citations
0IEEE Access, Journal Year: 2024, Volume and Issue: 12, P. 112095 - 112115
Published: Jan. 1, 2024
Language: Английский
Citations
0