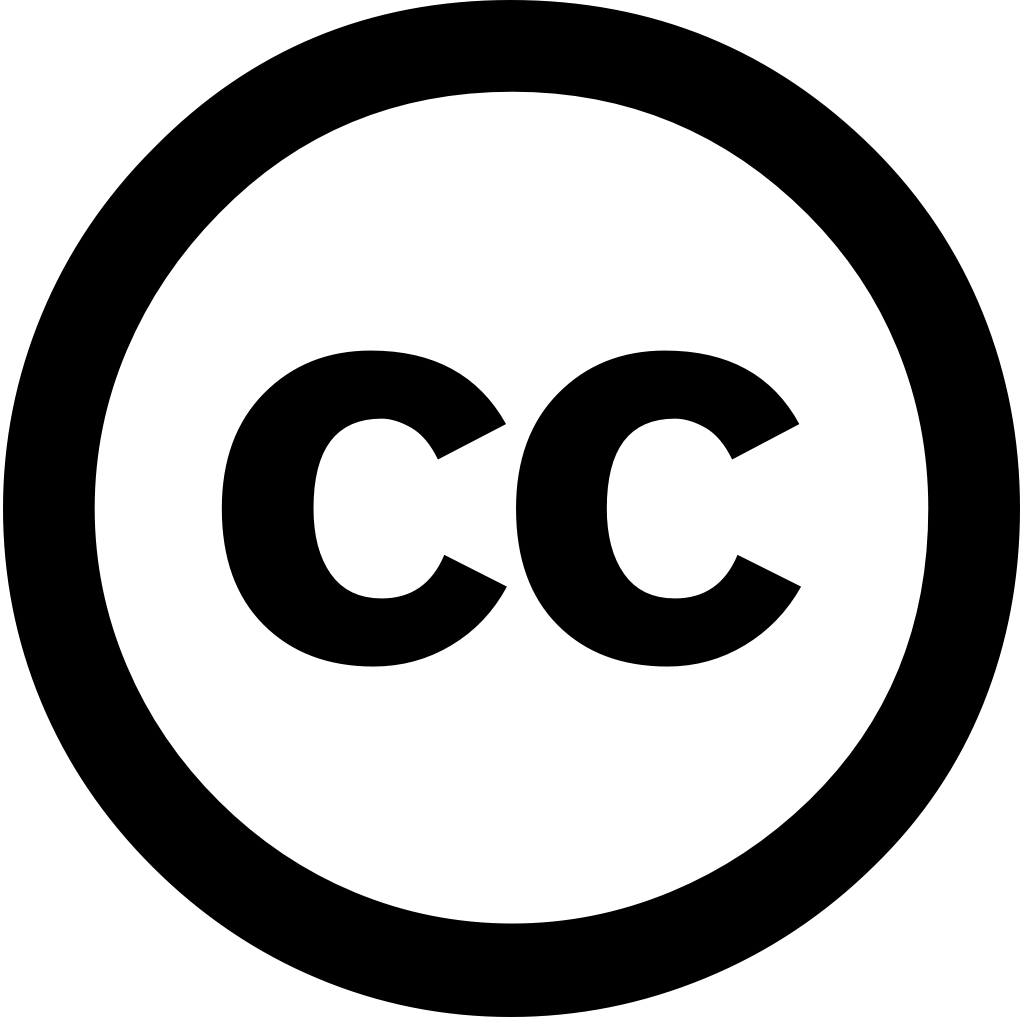
IEEE Access, Journal Year: 2024, Volume and Issue: 12, P. 84643 - 84679
Published: Jan. 1, 2024
Language: Английский
IEEE Access, Journal Year: 2024, Volume and Issue: 12, P. 84643 - 84679
Published: Jan. 1, 2024
Language: Английский
Blockchains, Journal Year: 2025, Volume and Issue: 3(1), P. 1 - 1
Published: Jan. 1, 2025
Federated learning (FL) has emerged as an efficient machine (ML) method with crucial privacy protection features. It is adapted for training models in Internet of Things (IoT)-related domains, including smart healthcare systems (SHSs), where the introduction IoT devices and technologies can arise various security concerns. However, FL cannot solely address all challenges, privacy-enhancing (PETs) blockchain are often integrated to enhance frameworks within SHSs. The critical questions remain regarding how these they contribute enhancing This survey addresses by investigating recent advancements on combination PETs healthcare. First, this emphasizes integration into context. Second, challenge integrating FL, it examines three main technical dimensions such blockchain-enabled model storage, aggregation, gradient upload frameworks. further explores collectively ensure integrity confidentiality data, highlighting their significance building a trustworthy SHS that safeguards sensitive patient information.
Language: Английский
Citations
3The Journal of Supercomputing, Journal Year: 2024, Volume and Issue: 81(1)
Published: Dec. 10, 2024
The integration of artificial intelligence (AI) has caused information and communication technology (ICT) to undergo a number recent rapid fluctuations. These changes have primarily affected the areas management, end-to-end device interconnectivity, resource organization, communication, networking, application-related aspects ICT. Owing complex structure applicational connectedness, evaluating each aforementioned opportunities concurrently reflects idea heterogeneity. association multiple end devices, particularly in interoperable space, integrity, privacy protection, security, provenance, massive volume everyday media data generated modern healthcare setting could also provide significant issues. To address these issues, decentralized, secure, economical optimization, intelligent network activities organization are necessary. Blockchain plays crucial role providing distributed storage sharing, exchange for automated decision-making, privacy, security AI-enabled machine learning (ML) models. However, models—support vector machine, particular—have impact on growth consortium networks among connected nodes, resolving issues with scalability, processing. By three main problems seamless peer-to-peer between infrastructure we novel technique this proposed architecture. approach is unique, as demonstrated by simulation-based results, which display huge differences 1.37%, 1.56%, 1.87%, respectively. background evaluation consists following areas: (i) protect decision-making; (ii) integrity smooth sharing exchange; (iii) optimization enable across heterogeneous devices.
Language: Английский
Citations
17Artificial Intelligence Review, Journal Year: 2024, Volume and Issue: 57(9)
Published: Aug. 8, 2024
Abstract The fusion of blockchain and artificial intelligence (AI) marks a paradigm shift in healthcare, addressing critical challenges securing electronic health records (EHRs), ensuring data privacy, facilitating secure transmission. This study provides comprehensive analysis the adoption AI within spotlighting their role fortifying security transparency leading trajectory for promising future realm healthcare. Our study, employing PRISMA model, scrutinized 402 relevant articles, narrative to explore review includes architecture blockchain, examines applications with without integration, elucidates interdependency between blockchain. major findings include: (i) it protects transfer, digital records, security; (ii) enhances EHR COVID-19 transmission, thereby bolstering healthcare efficiency reliability through precise assessment metrics; (iii) addresses like security, decentralized computing, forming robust tripod. revolutionize by EHRs, enhancing security. Private reflects sector’s commitment improved accessibility. convergence promises enhanced disease identification, response, overall efficacy, key sector challenges. Further exploration advanced features integrated enhance outcomes, shaping global delivery guaranteed innovation.
Language: Английский
Citations
11Elsevier eBooks, Journal Year: 2025, Volume and Issue: unknown, P. 89 - 108
Published: Jan. 1, 2025
Language: Английский
Citations
1Healthcare, Journal Year: 2024, Volume and Issue: 12(24), P. 2587 - 2587
Published: Dec. 22, 2024
Federated learning (FL) is revolutionizing healthcare by enabling collaborative machine across institutions while preserving patient privacy and meeting regulatory standards. This review delves into FL's applications within smart health systems, particularly its integration with IoT devices, wearables, remote monitoring, which empower real-time, decentralized data processing for predictive analytics personalized care. It addresses key challenges, including security risks like adversarial attacks, poisoning, model inversion. Additionally, it covers issues related to heterogeneity, scalability, system interoperability. Alongside these, the highlights emerging privacy-preserving solutions, such as differential secure multiparty computation, critical overcoming limitations. Successfully addressing these hurdles essential enhancing efficiency, accuracy, broader adoption in healthcare. Ultimately, FL offers transformative potential secure, data-driven promising improved outcomes, operational sovereignty ecosystem.
Language: Английский
Citations
5Published: April 29, 2024
In the ever-evolving healthcare sector, widespread adoption of Internet Things and wearable technologies facilitates remote patient monitoring. However, existing client/server infrastructure poses significant security privacy challenges, necessitating strict adherence to data regulations. To combat these issues, a decentralized approach is imperative, blockchain technology emerges as compelling solution for strengthening medical systems security. This paper introduces HNMblock, model that elevates realms epidemiological monitoring, system security, wellness enhancement. By harnessing transparency immutability inherent in blockchain, HNMblock empowers real-time, tamper-proof tracking data, enabling swift responses disease outbreaks. Furthermore, it fortifies through advanced cryptographic techniques smart contracts, with paramount focus on safeguarding privacy. also fosters personalized health care, encouraging involvement data-informed decision-making. The integration within domain, exemplified by holds potential revolutionize management, surveillance, wellness, meticulously explored this research article.
Language: Английский
Citations
4Advances in computational intelligence and robotics book series, Journal Year: 2025, Volume and Issue: unknown, P. 155 - 186
Published: March 7, 2025
Federated learning is an emerging powerful approach for training ML models across decentralized data sources, such as in healthcare, without actually transferring the sensitive to a central server. Although FL naturally reduces risks of privacy since are confined local devices, it still can be highly vulnerable sophisticated attacks model inversion, poisoning, and inference attacks. Advanced privacy-preserving techniques that nowadays studied addressing security concerns this paper include differential privacy, homomorphic encryption, secure aggregation. These, therefore, open room adopted solution some privacy-sensitive domains. This conducts thorough review discussed outlines customized framework healthcare applications allow patient safeguarded detrimental effects on performance accuracy. Conclusion:
Language: Английский
Citations
0Sensors, Journal Year: 2025, Volume and Issue: 25(9), P. 2795 - 2795
Published: April 29, 2025
Internet of Medical Things (IoMT) is a rapidly evolving field with the potential to bring significant changes healthcare. While several surveys have examined structure and operation these systems, critical aspects such as interoperability, sustainability, security, runtime self-adaptation, configurability are sometimes overlooked. Interoperability essential for integrating data from various devices platforms provide comprehensive view patient's health. Sustainability addresses environmental impact IoMT technologies, crucial in context green computing. Security ensures protection sensitive patient breaches manipulation. Runtime self-adaptation allows systems adjust changing conditions environments. Configurability enables frameworks monitor diverse manage different treatment paths. This article reviews current techniques addressing highlights areas requiring further research.
Language: Английский
Citations
0Future Internet, Journal Year: 2024, Volume and Issue: 17(1), P. 2 - 2
Published: Dec. 25, 2024
The development of medical data and resources has become essential for enhancing patient outcomes operational efficiency in an age when digital innovation healthcare is becoming more important. rapid growth the Internet Medical Things (IoMT) changing management, but it also brings serious issues like privacy, malicious attacks, service quality. In this study, we present EdgeGuard, a novel decentralized architecture that combines blockchain technology, federated learning, edge computing to address those challenges coordinate across IoMT networks. EdgeGuard uses privacy-preserving learning approach keep sensitive local promote collaborative model training, solving issues. To prevent modification unauthorized access, blockchain-based access control integrity verification system. improve system scalability by offloading computational tasks from devices with limited resources. We have made several technological advances, including lightweight consensus mechanism designed networks, adaptive resource allocation method based on reinforcement algorithm optimized differential privacy. create smart contracts secure multi-party protocol updates. outperforms existing solutions terms performance, value, privacy protection wide range real-world datasets. This work enhances safe, effective, management ecosystems while maintaining outstanding standards security efficiency, enabling large-scale healthcare.
Language: Английский
Citations
2Internet of Things, Journal Year: 2024, Volume and Issue: 28, P. 101420 - 101420
Published: Nov. 5, 2024
Language: Английский
Citations
1