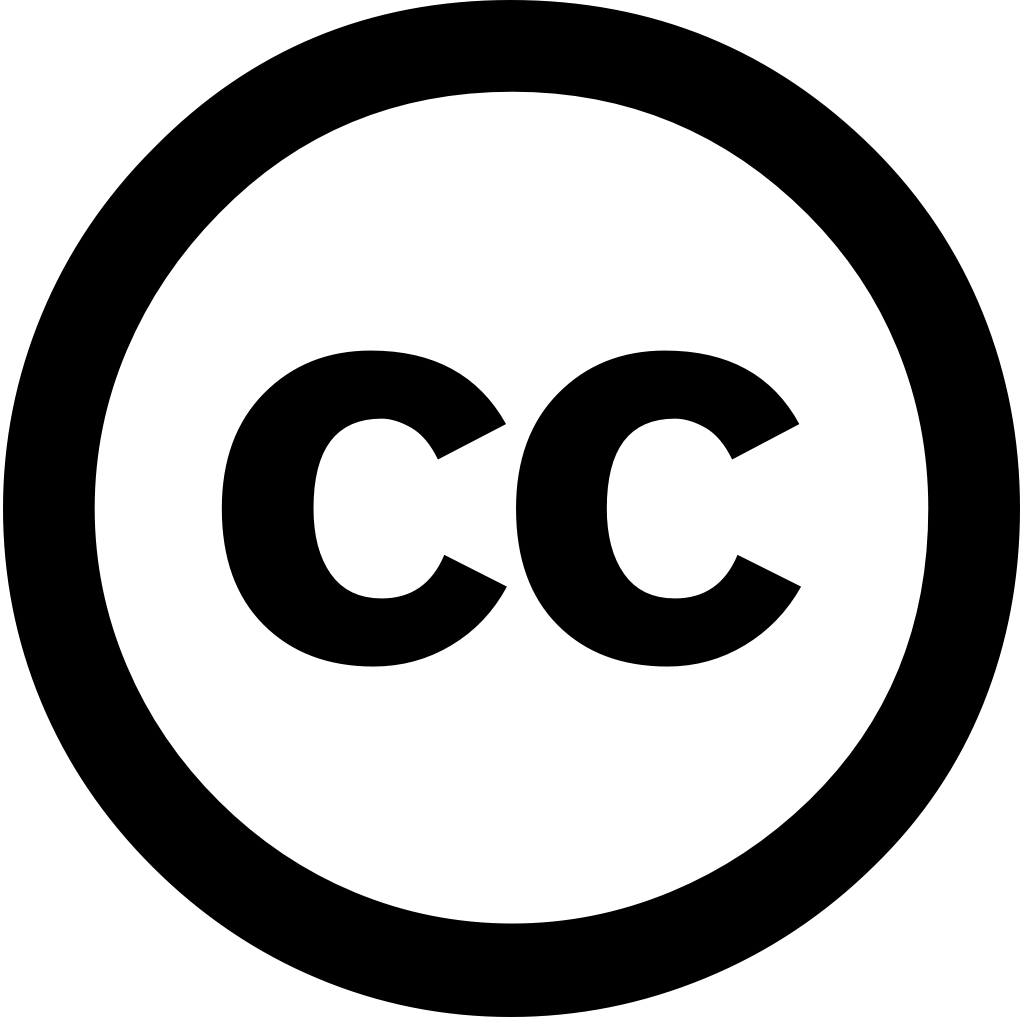
Current Biology, Journal Year: 2021, Volume and Issue: 32(1), P. 210 - 219.e4
Published: Nov. 3, 2021
Language: Английский
Current Biology, Journal Year: 2021, Volume and Issue: 32(1), P. 210 - 219.e4
Published: Nov. 3, 2021
Language: Английский
Journal of Wood Science, Journal Year: 2023, Volume and Issue: 69(1)
Published: Jan. 5, 2023
Abstract This study investigated the feature importance of near-infrared spectra from random forest regression models constructed to predict carbonization characteristics hydrochars produced by hydrothermal kraft lignin. The model achieved high coefficients determination 0.989, 0.988, and 0.985 with root mean square errors 0.254, 0.003, 0.008 when predicting carbon content, atomic O/C ratio, H/C respectively. outperformed multilayer perceptron for all predictions. In analysis, spectral regions at 1600–1800 nm, first overtone C–H stretching vibrations, 2000–2300 combination bands, were highly important content predictions, whereas region 1250–1711 nm contributed H/C. trained high-importance better prediction performances than those entire range, demonstrating usefulness yielded feasibility selective application data.
Language: Английский
Citations
31Metals, Journal Year: 2024, Volume and Issue: 14(2), P. 235 - 235
Published: Feb. 15, 2024
High-entropy alloys (HEAs) have attracted worldwide interest due to their excellent properties and vast compositional space for design. However, obtaining HEAs with low density high through experimental trial-and-error methods results in efficiency costs. Although high-throughput calculation (HTC) improves the design of HEAs, accuracy prediction is limited owing indirect correlation between theoretical values performances. Recently, machine learning (ML) from real data has increasing attention assist material design, which closely related performance. This review introduces common advanced ML models algorithms are used current HEA The advantages limitations these analyzed potential weaknesses corresponding optimization strategies discussed as well. suggests that acquisition, utilization, generation effective key issues development future
Language: Английский
Citations
16Molecular Ecology Resources, Journal Year: 2020, Volume and Issue: 20(6), P. 1526 - 1541
Published: June 20, 2020
Abstract As species extinction rates increase, genomics provides a powerful tool to support intensive management of threatened species. We use the Tasmanian devil ( Sarcophilus harrisii ) demonstrate how conservation can be implemented in management. conducted whole genome sequencing (WGS) 25 individuals from captive breeding programme and reduced‐representation (RRS) 98 founders same programme. A subset WGS samples was also sequenced by RRS, allowing us directly compare genome‐wide heterozygosity with estimates RRS data. found good congruence interindividual variation gene‐ontology classifications between two data sets, indicating that our reflect well. attempted association studies both sets (regarding success), but genomic suffered small sample size, while lack precision, highlighting key trade‐off design research. Nevertheless, we identified number candidate genes may associated success. Individual heterozygosity, as measured or not success captivity negatively litter sizes females set. Our findings enable managers have confidence understanding its limitations, provide avenues for further investigation into which processes underlie devils. caution, however, deep functional insights using impaired especially when marker density is low.
Language: Английский
Citations
70Journal of Dairy Science, Journal Year: 2019, Volume and Issue: 102(10), P. 9409 - 9421
Published: Aug. 22, 2019
Language: Английский
Citations
66Ecological Informatics, Journal Year: 2019, Volume and Issue: 52, P. 46 - 56
Published: May 9, 2019
Language: Английский
Citations
58Proceedings of the Royal Society B Biological Sciences, Journal Year: 2021, Volume and Issue: 288(1946), P. 20210177 - 20210177
Published: March 3, 2021
Climate-driven reef decline has prompted the development of next-generation coral conservation strategies, many which hinge on movement adaptive variation across genetic and environmental gradients. This process is limited by our understanding how genotypic drivers bleaching will manifest in different conditions. We reciprocally transplanted 10 genotypes Acropora cervicornis eight sites along a 60 km span Florida Reef Tract documented significant genotype × environment interactions response during severe 2015 event. Performance relative to site mean was significantly between can be mostly explained ensemble models correlations with markers. The high explanatory power driven enrichment loci associated DNA repair, cell signalling apoptosis. No performed above (or below) average at all sites, so genomic predictors provide practitioners ‘confidence intervals' about chance success novel habitats. These data have important implications for assisted gene flow managed relocation, their integration traditional active restoration.
Language: Английский
Citations
45Methods in Ecology and Evolution, Journal Year: 2021, Volume and Issue: 12(11), P. 2117 - 2128
Published: July 28, 2021
The ecological and environmental science communities have embraced machine learning (ML) for empirical modelling prediction. However, going beyond prediction to draw insights into underlying functional relationships between response variables 'drivers' is less straightforward. Deriving from fitted ML models requires techniques extract the 'learning' hidden in models.We revisit theoretical background effectiveness of four approaches deriving ML: ranking independent variable importance (Gini importance, GI; permutation PI; split SI; conditional CPI), two inference bivariate (partial dependence plots, PDP; accumulated local effect ALE). We also explore use a surrogate model visualization interpretation complex multi-variate drivers. examine challenges opportunities extracting with these approaches. Specifically, we aim improve by investigating how relates (a) algorithm, (b) sample size (c) presence spurious explanatory variables.We base analysis on simulations known predictor variables, added white noise correlated but non-influential variables. results indicate that insight strongly affected algorithm moderately impacted size. Removing improves models. Meanwhile, increasing has limited value does performance once are omitted. Among methods, SI slightly more effective than other methods while GI yield higher accuracy when removed. PDP retrieving ALE, its reliability declines sharply Visualization interactive effects predictors can be enhanced using models, including three-dimensional visualizations loess planes represent interactions.Machine analysts should aware no clear causal relationship interfere inference. When important, constructed While interpreting remains challenging, show careful choice exclusion adequate provide better 'learn learning'.机器学习已经被广泛应用于生态和环境科学中进行经验建模和预测, 然而我们并不能直接有效地从机器学习模型中获取重要的生态机制关系。因此从机器学习模型中获取生态机制关系亟需有效的方法提取模型中的潜在关系。在本研究中, 我们主要考察了以下相关方法的理论背景和有效性。其中四种方法用来估计环境解释变量的重要性, 包括: 基尼系数, 排列特征重要性, 基于增长回归树模型分裂树的特征重要性, 以及条件排列重要性。两种方法来获取解释变量与目标变量之间的功能关系: 部份依赖图和累积局部效应。此外, 我们采用一个代理模型来可视化并解释复杂的多变量关系。本文旨在检验应用以上方法获取生态解释的机遇和挑战, 尤其是他们受样本大小和冗余特征变量的影响。本研究基于一组已知潜在生态机制的全球物种丰富度的模拟数据, 并添加白噪声和相关但没有直接因果关系的冗余特征。研究结果显示从机器学习中获取生态机制最主要受解释方法以及冗余特征的影响, 其次是样本大小。剔除冗余变量可以显著提高模型的生态解释能力; 并且当冗余变量去除后, 增大样本大小可以提高解释效果。在四个特征重要性排列的方法中, 当模型中存在冗余特征时, 分裂特征重要性比其他方法稍显优势; 当冗余特征去除后, 基尼系数和分裂特征重要性都能获得更准确的结果。在任何情况下, 部份依赖图比都比累计局部效应更有效提取功能关系; 冗余特征的存在同样影响了部份依赖图的有效性。应用三维可视化和局部多项式回归的组合作为代理模型可以有效表达多个环境特征的交互作用。本研究结果显示我们需要关注冗余变量对获取生态机制的影响。如果我们需要从机器学习模型中获取潜在的生态机制, 机器学习模型中最好只包括与反应变量有清晰因果关系的特征变量。从机器学习中获取生态机制解释一直是一个关键的挑战, 本研究显示应用合适的解释方法, 剔除冗余变量以及应用充足的样本量可以显著提高从机器学习中“学习”的机会。.
Language: Английский
Citations
43Scientific Reports, Journal Year: 2022, Volume and Issue: 12(1)
Published: March 7, 2022
Abstract Determining the solubility of non-hydrocarbon gases such as carbon dioxide (CO 2 ) and nitrogen (N in water brine is one most controversial challenges oil chemical industries. Although many researches have been conducted on water, very few investigated power plant flue –N mixtures) aqueous solutions. In this study, using six intelligent models, including Random Forest, Decision Tree (DT), Gradient Boosting-Decision (GB-DT), Adaptive (AdaBoost-DT), Boosting-Support Vector Regression (AdaBoost-SVR), (GB-SVR), CO mixtures solutions was predicted, results were compared with four equations state (EOSs), Peng–Robinson (PR), Soave–Redlich–Kwong (SRK), Valderrama–Patel–Teja (VPT), Perturbed-Chain Statistical Associating Fluid Theory (PC-SAFT). The indicate that Forest model an average absolute percent relative error (AAPRE) value 2.8% has best predictions. GB-SVR DT models also good precision AAPRE values 6.43% 7.41%, respectively. For present gaseous systems, PC-SAFT model, for N , VPT EOS had among EOSs. Also, sensitivity analysis input parameters showed increasing mole phase, temperature, pressure, decreasing ionic strength increase mixture Another significant issue salinity a subtractive effect mixture. Finally, Leverage method proved actual data are excellent quality approach quite reliable determining gas systems.
Language: Английский
Citations
38Frontiers in Plant Science, Journal Year: 2022, Volume and Issue: 13
Published: Dec. 6, 2022
Climate change across the globe has an impact on occurrence, prevalence, and severity of plant diseases. About 30% yield losses in major crops are due to diseases; emerging diseases likely worsen sustainable production coming years. Plant have led increased hunger mass migration human populations past, thus a serious threat global food security. Equipping modern varieties/hybrids with enhanced genetic resistance is most economic, environmentally friendly solution. geneticists done tremendous work identifying stable primary genepools many times other than breed resistant varieties different crops. Over last two decades, availability crop pathogen genomes advances next generation sequencing technologies improved our understanding trait genetics using approaches. Genome-wide association studies been effectively used identify candidate genes map loci associated plants. In this review, we highlight successful examples for discovery important addition, developments studies, statistical models bioinformatic tools that improve power, resolution efficiency marker-trait associations. Overall review provides comprehensive insights into decades GWAS discusses challenges opportunities research area breeding varieties.
Language: Английский
Citations
35Journal of Dairy Science, Journal Year: 2023, Volume and Issue: 106(5), P. 3321 - 3344
Published: April 6, 2023
The adoption of preventive management decisions is crucial to dealing with metabolic impairments in dairy cattle. Various serum metabolites are known be useful indicators the health status cows. In this study, we used milk Fourier-transform mid-infrared (FTIR) spectra and various machine learning (ML) algorithms develop prediction equations for a panel 29 blood metabolites, including those related energy metabolism, liver function/hepatic damage, oxidative stress, inflammation/innate immunity, minerals. For most traits, data set comprised observations from 1,204 Holstein-Friesian cows belonging 5 herds. An exception was represented by β-hydroxybutyrate prediction, which contained 2,701 multibreed pertaining 33 best predictive model developed using an automatic ML algorithm that tested methods, elastic net, distributed random forest, gradient boosting machine, artificial neural network, stacking ensemble. These predictions were compared partial least squares regression, commonly method FTIR traits. Performance each evaluated 2 cross-validation (CV) scenarios: 5-fold (CVr) herd-out (CVh). We also model's ability classify values precisely extreme tails, namely, 25th (Q25) 75th (Q75) percentiles (true-positive scenario). Compared achieved more accurate performance. Specifically, net increased R2 value 5% 75% CVr 2% 139% CVh, whereas ensemble 4% 70% 150% CVh. Considering model, scenario, good accuracies obtained glucose (R2 = 0.81), urea 0.73), albumin 0.75), total reactive oxygen 0.79), thiol groups 0.76), ceruloplasmin 0.74), proteins globulins 0.87), Na 0.72). Good accuracy classifying (Q25 70.8%, Q75 69.9%), 72.3%), 75.1%, 74%), (Q75 70.4%), 72.4%, 77.2.%), 74.8%, 81.5%), haptoglobin 74.4%). conclusion, our study shows can predict relatively accuracy, depending on trait, promising tool large-scale monitoring.
Language: Английский
Citations
22