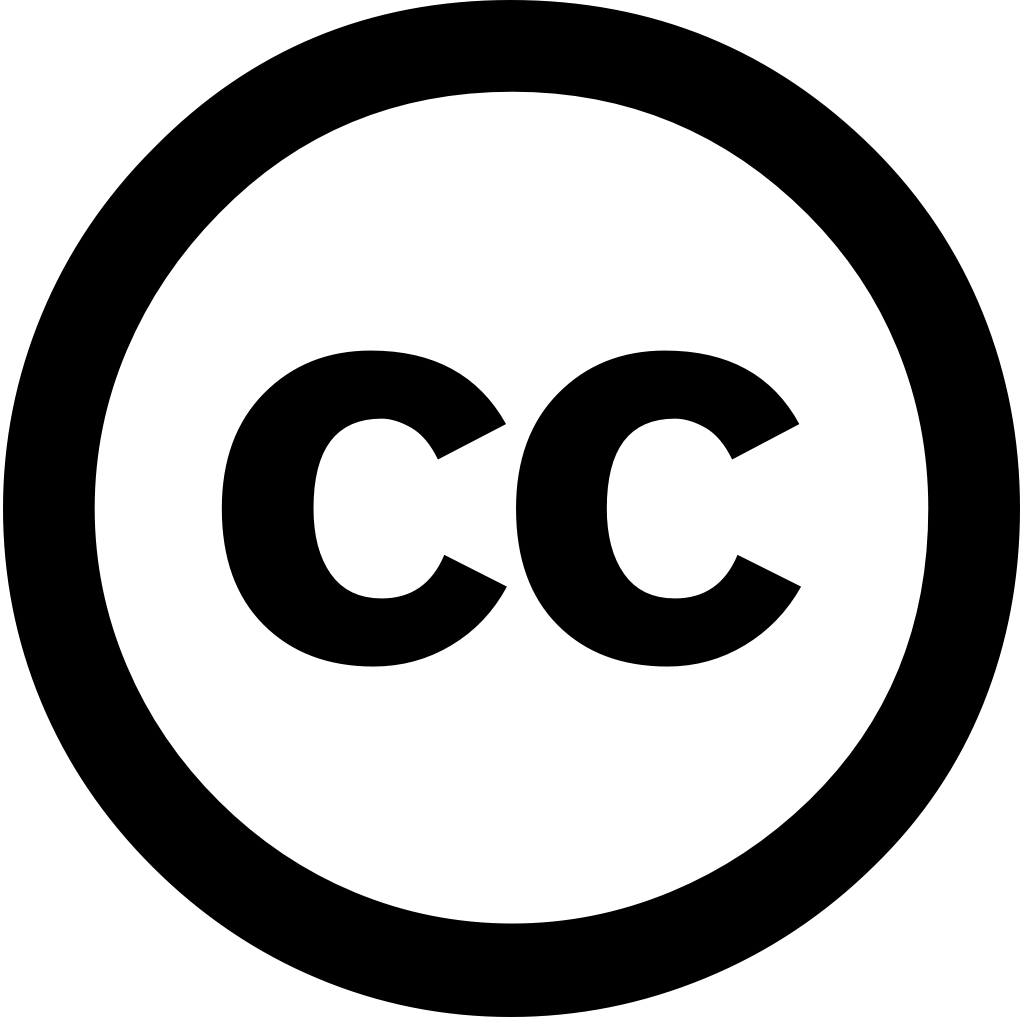
Published: May 9, 2024
Language: Английский
Published: May 9, 2024
Language: Английский
JAMA Network Open, Journal Year: 2024, Volume and Issue: 7(5), P. e2413213 - e2413213
Published: May 28, 2024
Importance The ratio of red blood cell distribution width (RDW) to albumin concentration (RAR) has emerged as a reliable prognostic marker for mortality in patients with various diseases. However, whether RAR is associated the general population remains unknown. Objectives To explore all-cause and cause-specific elucidate their dose-response association. Design, Setting, Participants This population-based prospective cohort study used data from participants 1998-2018 US National Health Nutrition Examination Survey (NHANES) UK Biobank baseline information provided 2006 2010. Included had complete on serum concentration, RDW, cause death. NHANES were linked Death Index records through December 31, 2019. For Biobank, dates causes death obtained Service Information Centre (England Wales) Central Register Scotland (Scotland) November 30, 2022. Main Outcomes Measures Potential associations between risk evaluated using Cox proportional hazards regression models. Restricted cubic spline regressions applied estimate possible nonlinear associations. Results In NHANES, 50 622 18 years age or older included (mean [SD] age, 48.6 [18.7] years; 26 136 [51.6%] female), mean (SD) was 3.15 (0.51). 418 950 37 [SD], 56.6 [8.1] 225 038 [53.7%] female) included, 2.99 (0.31). documented 7590 deaths over median (IQR) follow-up 9.4 (5.1-14.2) years, 36 793 13.8 (13.0-14.5) years. According multivariate analysis, elevated significantly greater (NHANES: hazard [HR], 1.83 [95% CI, 1.76-1.90]; Biobank: HR, 2.08 2.03-2.13]), well due malignant neoplasm 1.89 1.73-2.07]; 1.93 1.86-2.00]), heart disease 1.88 1.74-2.03]; 2.42 2.29-2.57]), cerebrovascular 1.35 1.07-1.69]; 2.15 1.91-2.42]), respiratory 1.99 1.68-2.35]; 2.96 2.78-3.15]), diabetes 1.55 1.27-1.90]; 2.83 2.35-3.40]), other 1.97 1.86-2.08]; 2.40 2.30-2.50]) both cohorts. Additionally, association observed levels Conclusions Relevance this study, higher an increased population. These findings suggest that may be simple, reliable, inexpensive indicator identifying individuals at high clinical practice.
Language: Английский
Citations
23Aging Cell, Journal Year: 2023, Volume and Issue: 22(8)
Published: June 10, 2023
Abstract Attaining personalized healthy aging requires accurate monitoring of physiological changes and identifying subclinical markers that predict accelerated or delayed aging. Classic biostatistical methods most rely on supervised variables to estimate do not capture the full complexity inter‐parameter interactions. Machine learning (ML) is promising, but its black box nature eludes direct understanding, substantially limiting physician confidence clinical usage. Using a broad population dataset from National Health Nutrition Examination Survey (NHANES) study including routine biological after selection XGBoost as appropriate algorithm, we created an innovative explainable ML framework determine Personalized age (PPA). PPA predicted both chronic disease mortality independently chronological age. Twenty‐six were sufficient PPA. SHapley Additive exPlanations (SHAP), implemented precise quantitative associated metric for each variable explaining (i.e., delayed) deviations age‐specific normative data. Among variables, glycated hemoglobin (HbA1c) displays major relative weight in estimation Finally, clustering profiles identical contextualized explanations reveal different trajectories opening opportunities specific follow‐up. These data show robust, ML‐based monitors health status. Our approach also provides complete applicable datasets allowing precision estimation.
Language: Английский
Citations
29Pflügers Archiv - European Journal of Physiology, Journal Year: 2025, Volume and Issue: unknown
Published: Feb. 25, 2025
Abstract Explainable artificial intelligence (XAI) is gaining importance in physiological research, where now used as an analytical and predictive tool for many medical research questions. The primary goal of XAI to make AI models understandable human decision-makers. This can be achieved particular through providing inherently interpretable methods or by making opaque their outputs transparent using post hoc explanations. review introduces core topics provides a selective overview current physiology. It further illustrates solved discusses open challenges existing practical examples from the field. article gives outlook on two possible future prospects: (1) provide trustworthy integrative (2) integrating expertise about explanation into method development useful beneficial human-AI partnerships.
Language: Английский
Citations
1Ageing Research Reviews, Journal Year: 2023, Volume and Issue: 93, P. 102144 - 102144
Published: Nov. 28, 2023
Language: Английский
Citations
18Nutrition & Metabolism, Journal Year: 2024, Volume and Issue: 21(1)
Published: May 14, 2024
Abstract Background Gout prediction is essential for the development of individualized prevention and treatment plans. Our objective was to develop an efficient interpretable machine learning (ML) model using SHapley Additive exPlanation (SHAP) link dietary fiber triglyceride-glucose (TyG) index predict gout. Methods Using datasets from National Health Nutrition Examination Survey (NHANES) (2005–2018) population study fiber, TyG used After evaluating performance six ML models selecting Light Gradient Boosting Machine (LGBM) as optimal algorithm, we interpret LGBM predicting gout SHAP reveal decision-making process model. Results An initial survey 70,190 participants conducted, after a gradual exclusion process, 12,645 cases were finally included in study. Selection best performing associated with (Area under ROC curve (AUC): 0.823, 95% confidence interval (CI): 0.798–0.848, Accuracy: 95.3%, Brier score: 0.077). The feature importance values indicated that age most important affecting output, followed by uric acid (UA). showed lower had more pronounced effect on positive model, while higher Conclusion high accuracy, efficiency, robustness Increasing intake lowering are beneficial reducing potential risk
Language: Английский
Citations
7Frontiers in Endocrinology, Journal Year: 2024, Volume and Issue: 15
Published: March 8, 2024
Background Obesity and metabolic syndrome pose significant health challenges in the United States (US), with connections to disruptions sex hormone regulation. The increasing prevalence of obesity might be associated exposure phthalates (PAEs). Further exploration impact PAEs on is crucial, particularly from a perspective. Methods A total 7780 adult participants National Health Nutrition Examination Survey (NHANES) 2013 2016 were included study. Principal component analysis (PCA) coupled multinomial logistic regression was employed elucidate association between urinary metabolite concentrations likelihood obesity. Weighted quartiles sum (WQS) utilized consolidate mixed levels (total testosterone (TT), estradiol hormone-binding globulin (SHBG)). We also delved into machine learning models accurately discern status identify key variables contributing most these models. Results Component 1 (PC1), characterized by mono(2-ethyl-5-carboxypentyl) phthalate (MECPP), mono(2-ethyl-5-hydroxyhexyl) (MEHHP), mono(2-ethyl-5-oxohexyl) (MEOHP) as major contributors, exhibited negative Conversely, PC2, monocarboxyononyl (MCNP), monocarboxyoctyl (MCOP), mono(3-carboxypropyl) (MCPP) showed positive Mixed decreased TT increased SHBG. During interrelations among obesity, hormones, PAEs, based Random Forest (RF) eXtreme Gradient Boosting (XGBoost) algorithms demonstrated best classification efficacy. In both models, hormones highest variable importance, certain metabolites made contributions model’s performance. Conclusions Individuals exhibit lower SHBG, accompanied elevated levels. Exposure disrupts levels, an risk US adults. interrelationships three factors, RF XGBoost algorithm superior performance, displaying higher importance.
Language: Английский
Citations
6Cell Metabolism, Journal Year: 2024, Volume and Issue: unknown
Published: Dec. 1, 2024
Language: Английский
Citations
4Frontiers in Neurology, Journal Year: 2025, Volume and Issue: 15
Published: Jan. 10, 2025
Spontaneous intracerebral hemorrhage (SICH) is the second most common cause of cerebrovascular disease after ischemic stroke, with high mortality and disability rates, imposing a significant economic burden on families society. This retrospective study aimed to develop evaluate an interpretable machine learning model predict functional outcomes 3 months SICH. A analysis was conducted clinical data from 380 patients SICH who were hospitalized at three different centers between June 2020 2023. Seventy percent samples randomly selected as training set, while remaining 30% used validation set. Univariate analysis, Least Absolute Shrinkage Selection Operator (LASSO) regression, Pearson correlation screen variables. The variables then incorporated into five models: complementary naive bayes (CNB), support vector (SVM), gaussian (GNB), multilayer perceptron (MLP), extreme gradient boosting (XGB), assess their performance. Additionally, area under curve (AUC) values evaluated compare performance each algorithmic model, global individual interpretive analyses using importance ranking Shapley additive explanations (SHAP). Among patients, 95 ultimately had poor prognostic outcomes. In AUC for CNB, SVM, GNB, MLP, XGB models 0.899 (0.816-0.979), 0.916 (0.847-0.982), 0.730 (0.602-0.857), 0.913 (0.834-0.986), 0.969 (0.937-0.998), respectively. Therefore, performed best among algorithms. SHAP revealed that GCS score, hematoma volume, blood pressure changes, platelets, age, bleeding location, glucose levels important prognosis. developed in this can effectively risk prognosis SICH, helping clinicians make personalized rational decisions. Prognostic closely associated levels.
Language: Английский
Citations
0European Radiology, Journal Year: 2025, Volume and Issue: unknown
Published: Jan. 24, 2025
Language: Английский
Citations
0Journal of Gastrointestinal Surgery, Journal Year: 2025, Volume and Issue: unknown, P. 101997 - 101997
Published: Feb. 1, 2025
Language: Английский
Citations
0