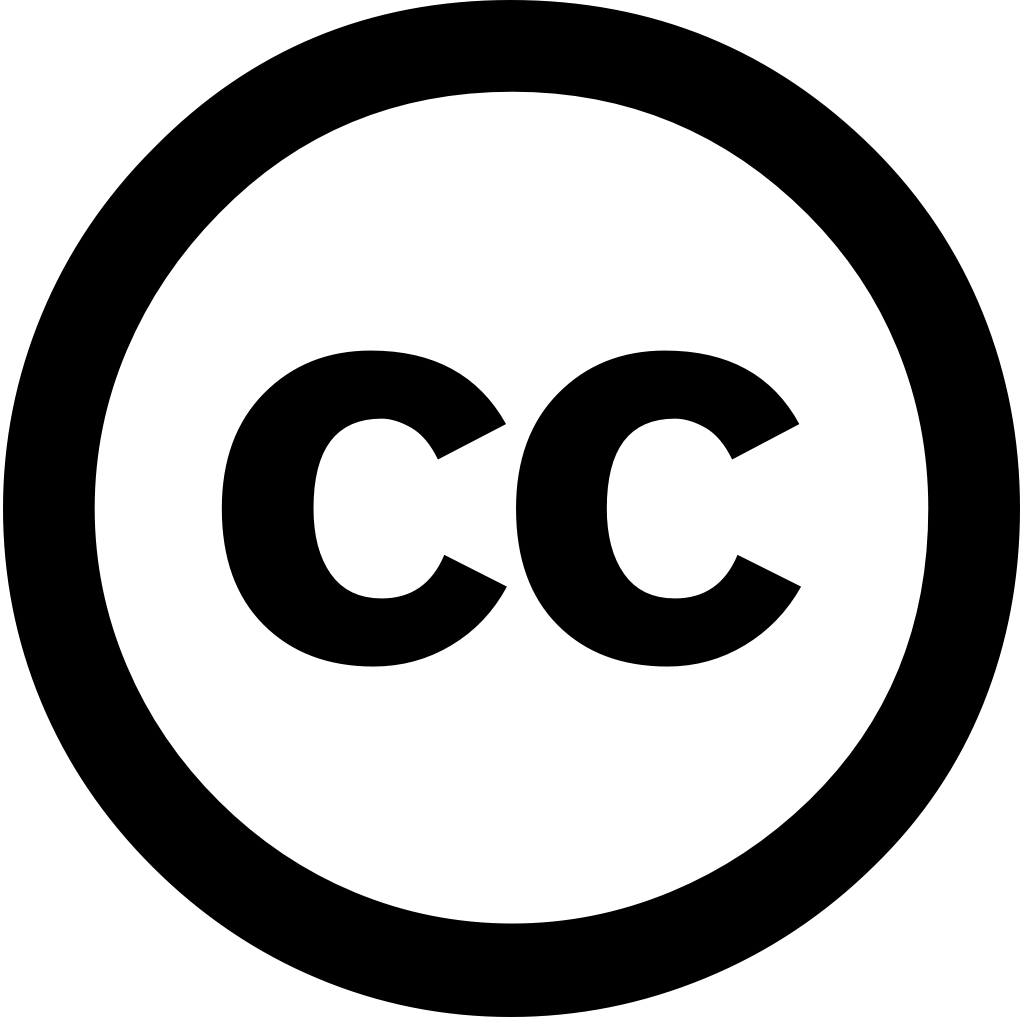
Carbon Capture Science & Technology, Journal Year: 2025, Volume and Issue: 14, P. 100374 - 100374
Published: Jan. 30, 2025
Language: Английский
Carbon Capture Science & Technology, Journal Year: 2025, Volume and Issue: 14, P. 100374 - 100374
Published: Jan. 30, 2025
Language: Английский
ACS Polymers Au, Journal Year: 2023, Volume and Issue: 3(3), P. 239 - 258
Published: Jan. 18, 2023
In the last five years, there has been tremendous growth in machine learning and artificial intelligence as applied to polymer science. Here, we highlight unique challenges presented by polymers how field is addressing them. We focus on emerging trends with an emphasis topics that have received less attention review literature. Finally, provide outlook for field, outline important areas science discuss advances from greater material community.
Language: Английский
Citations
85Desalination, Journal Year: 2023, Volume and Issue: 573, P. 117200 - 117200
Published: Dec. 4, 2023
Language: Английский
Citations
82Separation and Purification Technology, Journal Year: 2023, Volume and Issue: 335, P. 126022 - 126022
Published: Dec. 14, 2023
Language: Английский
Citations
68Exploration, Journal Year: 2023, Volume and Issue: 3(4)
Published: July 6, 2023
Flame retardants are currently used in a wide range of industry sectors for saving lives and property by mitigating fire hazards. The growing safety requirements materials boost an escalating demand consumption retardants. This has significantly driven both the scientific community to pursue sustainable retardants, but what makes flame retardant? Here overview recent advances is offered, their renewable raw materials, green synthesis life cycle assessments highlighted. A discussion on key challenges that hinder innovation design principles creating truly yet cost-effective also presented. short work expected help drive development sustainable, expedite creation more safer society.
Language: Английский
Citations
48Nature Reviews Materials, Journal Year: 2024, Volume and Issue: 9(12), P. 866 - 886
Published: Aug. 19, 2024
Language: Английский
Citations
26Advanced Materials, Journal Year: 2024, Volume and Issue: 36(18)
Published: Jan. 19, 2024
Abstract Machine learning holds significant research potential in the field of nanotechnology, enabling nanomaterial structure and property predictions, facilitating materials design discovery, reducing need for time‐consuming labor‐intensive experiments simulations. In contrast to their achiral counterparts, application machine chiral nanomaterials is still its infancy, with a limited number publications date. This despite great advance development new sustainable high values optical activity, circularly polarized luminescence, enantioselectivity, as well analysis structural chirality by electron microscopy. this review, an methods used studying provided, subsequently offering guidance on adapting extending work nanomaterials. An overview within framework synthesis–structure–property–application relationships presented insights how leverage study these highly complex are provided. Some key recent reviewed discussed Finally, review captures achievements, ongoing challenges, prospective outlook very important field.
Language: Английский
Citations
24Nano Letters, Journal Year: 2024, Volume and Issue: 24(10), P. 2953 - 2960
Published: March 4, 2024
Porous membranes, either polymeric or two-dimensional materials, have been extensively studied because of their outstanding performance in many applications such as water filtration. Recently, inspired by the significant success machine learning (ML) areas scientific discovery, researchers started to tackle problem field membrane design using data-driven ML tools. In this Mini Review, we summarize research efforts on three types design, including (1) property prediction ML, (2) gaining physical insight and drawing quantitative relationships between properties explainable artificial intelligence, (3) ML-guided optimization, virtual screening membranes. On top review previous research, discuss challenges associated with applying for potential future directions.
Language: Английский
Citations
17Progress in Materials Science, Journal Year: 2025, Volume and Issue: unknown, P. 101432 - 101432
Published: Jan. 1, 2025
Citations
3SmartMat, Journal Year: 2025, Volume and Issue: 6(1)
Published: Jan. 9, 2025
ABSTRACT Machine learning (ML), material genome, and big data approaches are highly overlapped in their strategies, algorithms, models. They can target various definitions, distributions, correlations of concerned physical parameters given polymer systems, have expanding applications as a new paradigm indispensable to conventional ones. Their inherent advantages building quantitative multivariate largely enhanced the capability scientific understanding discoveries, thus facilitating mechanism exploration, prediction, high‐throughput screening, optimization, rational inverse designs. This article summarizes representative progress recent two decades focusing on design, preparation, application, sustainable development materials based exploration key composition–process–structure–property–performance relationship. The integration both data‐driven insights through ML deepen fundamental discover novel is categorically presented. Despite construction application robust models, strategies algorithms deal with variant tasks science still rapid growth. challenges prospects then We believe that innovation will thrive along approaches, from efficient design applications.
Language: Английский
Citations
2Journal of Membrane Science, Journal Year: 2022, Volume and Issue: 665, P. 121131 - 121131
Published: Oct. 27, 2022
Language: Английский
Citations
45