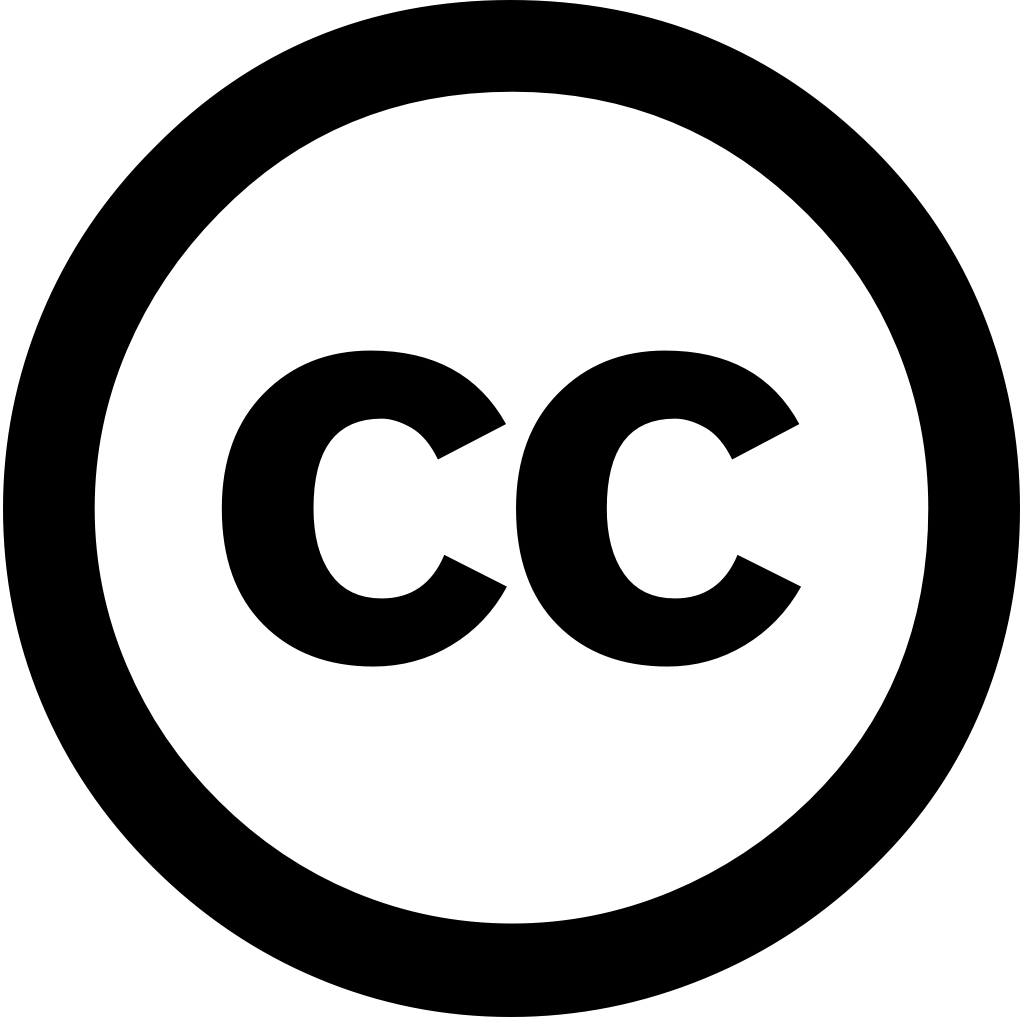
International Journal of Computational Intelligence Systems, Journal Year: 2023, Volume and Issue: 16(1)
Published: Nov. 27, 2023
Language: Английский
International Journal of Computational Intelligence Systems, Journal Year: 2023, Volume and Issue: 16(1)
Published: Nov. 27, 2023
Language: Английский
Journal of Medical Systems, Journal Year: 2023, Volume and Issue: 47(1)
Published: Feb. 1, 2023
Nowadays, Artificial Intelligence (AI) and machine learning (ML) have successfully provided automated solutions to numerous real-world problems. Healthcare is one of the most important research areas for ML researchers, with aim developing disease prediction systems. One detection problems that AI researchers focused on dementia using methods. Numerous diagnostic systems based techniques early been proposed in literature. Few systematic literature reviews (SLR) conducted past. However, these SLR a single type data modality dementia. Hence, purpose this study conduct comprehensive evaluation ML-based considering different types modalities such as images, clinical-features, voice data. We collected articles from 2011 2022 keywords dementia, learning, feature selection, modalities, The selected were critically analyzed discussed. It was observed image driven models yields promising results terms compared other i.e., clinical feature-based Furthermore, highlighted limitations previously methods presented future directions overcome limitations.
Language: Английский
Citations
87Journal of Hazardous Materials, Journal Year: 2023, Volume and Issue: 457, P. 131680 - 131680
Published: May 23, 2023
Being an essential component in the plastics industry, phthalates are ubiquitous environment and everyday life. They considered environmental contaminants that have been classified as endocrine-disrupting compounds. Despite di-2-ethylhexyl phthalate (DEHP) being most common plasticizer studied to date, there many others that, addition widely used plastic, also applied medical pharmaceutical industries cosmetics. Due their wide use, easily absorbed by human body where they can disrupt endocrine system binding molecular targets interfering with hormonal homeostasis. Thus, exposure has implicated development of several diseases different age groups. Collecting information from recent available literature, this review aims relate phthalates’ cardiovascular throughout all ages. Overall, studies presented demonstrated association between diseases, either prenatal or postnatal exposure, affecting foetuses, infants, children, young older adults. However, mechanisms underlying these effects remain poorly explored. considering incidence worldwide constant phthalates, topic should be extensively understand involved.
Language: Английский
Citations
69International Journal of Computational and Experimental Science and Engineering, Journal Year: 2025, Volume and Issue: 11(1)
Published: Feb. 16, 2025
During the past few years, Frequent Pattern Mining (FPM) has received interest of several researchers that necessitate extracting items from transactions, and sequences datasets, clarifying heart disease diagnosis materializes commonly, recognizing specific arrangements. In this era with healthcare involving significant evolutions, unforeseeable movement enormous amount data concerning classification lead way to new issues in FPM, such as space time complexity. However, most research work concentrates on identifying patterns relating transpires frequently, where within every transaction were known a priori. To address present scenario, selecting predominant or frequent is essential using relevant FPM models. The primary objective enhance mining results reduce misclassification rate Cardiovascular Disease (CVD) dataset samples. This proposes novel method called Renyi Entropy Homogenized Weighted Xavier-based Deep Neural Classifier (REHWX-DNC) for prediction. tackle first challenge, Entropy-based (RE-FPM) algorithm proposed, which filters low-quality features function. handle second issue, HWX-DNC model designed assist minimizing by employing Swish activation A CVD synthesis can be analyzed obtain accuracy study, REGEX-DNC improved compared state-of-the-art methods. Some indicators, including prediction accuracy, time, level, F1-total, are considered calculate predictor, checking REHWX-DNC proposed efficient trustworthy predicting disease.
Language: Английский
Citations
3Expert Systems with Applications, Journal Year: 2022, Volume and Issue: 213, P. 118933 - 118933
Published: Sept. 29, 2022
Language: Английский
Citations
45The Journal of Supercomputing, Journal Year: 2023, Volume and Issue: 79(11), P. 11797 - 11826
Published: March 3, 2023
Language: Английский
Citations
40Journal Of Big Data, Journal Year: 2023, Volume and Issue: 10(1)
Published: Sept. 17, 2023
Abstract The identification and prognosis of the potential for developing Cardiovascular Diseases (CVD) in healthy individuals is a vital aspect disease management. Accessing comprehensive health data on CVD currently available within hospital databases holds significant early detection diagnosis CVD, thereby positively impacting outcomes. Therefore, incorporation machine learning methods promise advancement clinical practice management (CVDs). By providing means to develop evidence-based guidelines algorithms, these techniques can eliminate need costly extensive laboratory investigations, reducing associated financial burden patients healthcare system. In order optimize prediction intervention CVDs, this study proposes development novel, robust, effective, efficient specifically designed automatic selection key features early-stage heart disease. proposed Catboost model yields an F1-score about 92.3% average accuracy 90.94%. Compared many other existing state-of-art approaches, it successfully achieved maximized classification performance with higher percentages precision.
Language: Английский
Citations
40Published: Feb. 9, 2023
Language: Английский
Citations
38Advances in medical education, research, and ethics (AMERE) book series, Journal Year: 2023, Volume and Issue: unknown, P. 331 - 363
Published: March 13, 2023
A visual analysis may accurately predict cardiac arrest, making it a potent educational tool for raising public awareness of health issues. By predicting arrest earlier, preventative steps can be taken to save lives, and the dissemination such knowledge dramatically lower world mortality rate. heart attack, also known as encompasses various heart-related disorders has been leading cause death worldwide in recent decades. Several medical data mining machine learning technologies are being applied gather helpful regarding disease prediction. The accuracy intended outcomes, however, is insufficient. This chapter aims likelihood patients having solve issue. Specifically, compared alternative models identification appropriately categorize forecast attack instances with compact features. use ensemble algorithms over classifier gives maximum 96.5%, which examined this investigation.
Language: Английский
Citations
24Scientific Reports, Journal Year: 2023, Volume and Issue: 13(1)
Published: May 3, 2023
Heart disease remains the major cause of death, despite recent improvements in prediction and prevention. Risk factor identification is main step diagnosing preventing heart disease. Automatically detecting risk factors for clinical notes can help with progression modeling decision-making. Many studies have attempted to detect disease, but none identified all factors. These proposed hybrid systems that combine knowledge-driven data-driven techniques, based on dictionaries, rules, machine learning methods require significant human effort. The National Center Informatics Integrating Biology Beyond (i2b2) a natural language processing (NLP) challenge 2014, track (track2) focused over time. Clinical narratives provide wealth information be extracted using NLP Deep Learning techniques. objective this paper improve previous work area as part 2014 i2b2 by identifying tags attributes relevant diagnosis, factors, medications providing advanced techniques stacked word embeddings. dataset has shown improvement approach stacking embeddings, which combines various Our model achieved an F1 score 93.66% BERT character embeddings (CHARACTER-BERT Embedding) stacking. results compared other models we developed challenge.
Language: Английский
Citations
23Artificial Intelligence Review, Journal Year: 2024, Volume and Issue: 57(10)
Published: Aug. 19, 2024
Heart disease (HD) is one of the leading causes death in humans, posing a heavy burden on society, families, and patients. Real-time prediction HD can reduce mortality rates crucial for timely intervention treatment HD. Deep learning (DL)-related methods have higher accuracy real-time performance predicting In this study, we comprehensively compared evaluated contributions limitations DL algorithms, extended deep (ETDL) integrated (integrated DL) algorithms that combine with other technologies The articles considered span period from 2018 to 2023, after rigorous screening, 64 were selected preliminary research. A systematic literature review HDP will provide future researchers comprehensive understanding existing related healthcare industry. Furthermore, it discusses popular datasets employed deploying numerous models. Additionally, reveals open problems or challenges encountered by previous researchers. Notably, most prevalent challenge scarcity large discrete datasets, followed need further improvement
Language: Английский
Citations
9