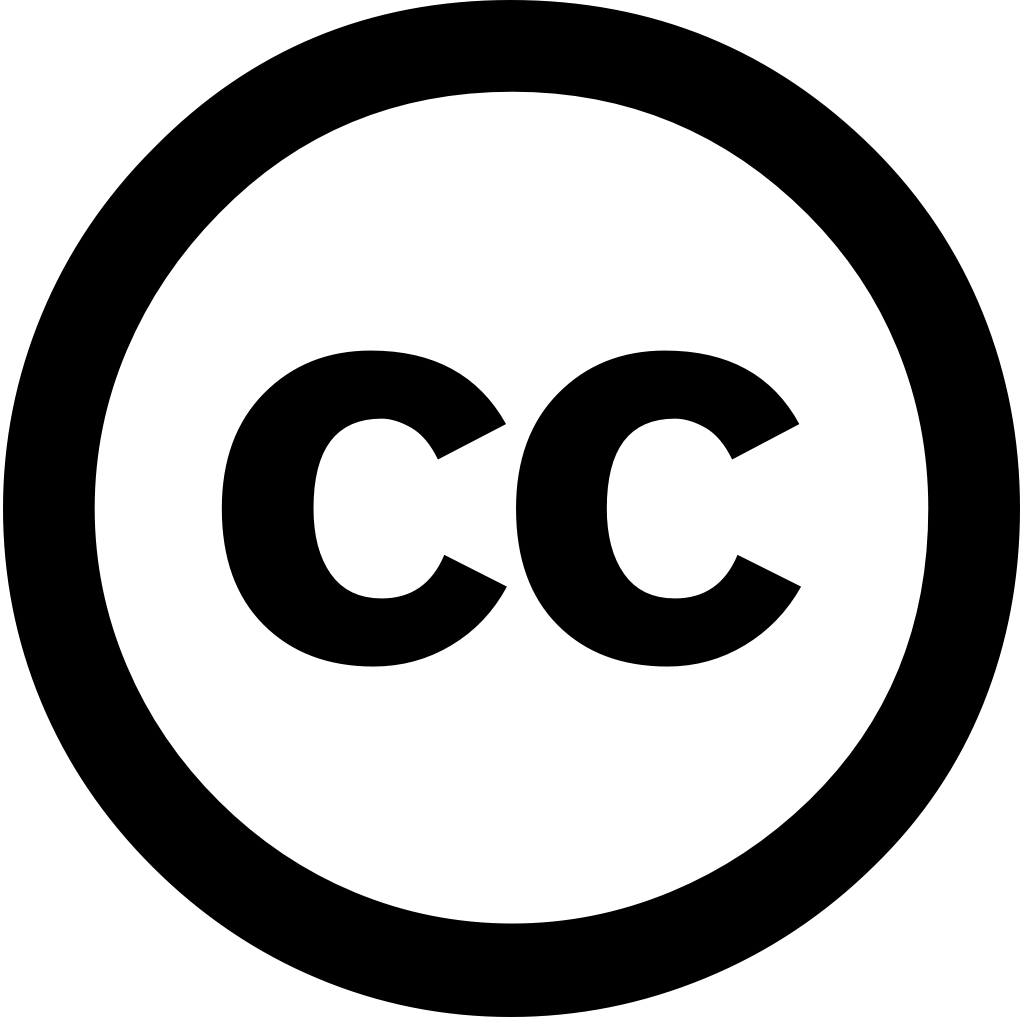
Technical Innovations & Patient Support in Radiation Oncology, Journal Year: 2022, Volume and Issue: 25, P. 100200 - 100200
Published: Dec. 30, 2022
Language: Английский
Technical Innovations & Patient Support in Radiation Oncology, Journal Year: 2022, Volume and Issue: 25, P. 100200 - 100200
Published: Dec. 30, 2022
Language: Английский
Diagnostics, Journal Year: 2023, Volume and Issue: 13(4), P. 667 - 667
Published: Feb. 10, 2023
Developers and users of artificial-intelligence-based tools for automatic contouring treatment planning in radiotherapy are expected to assess clinical acceptability these tools. However, what is ‘clinical acceptability’? Quantitative qualitative approaches have been used this ill-defined concept, all which advantages disadvantages or limitations. The approach chosen may depend on the goal study as well available resources. In paper, we discuss various aspects acceptability’ how they can move us toward a standard defining new autocontouring
Language: Английский
Citations
55Advances in Radiation Oncology, Journal Year: 2024, Volume and Issue: 9(5), P. 101470 - 101470
Published: Feb. 8, 2024
PurposeManual contour work for radiation treatment planning takes significant time to ensure volumes are accurately delineated. The use of artificial intelligence with deep learning based autosegmentation (DLAS) models has made itself known in recent years alleviate this workload. It is used organs at risk (OAR) contouring consistency performance and saving. purpose study was evaluate the current published data DLAS clinical target volume (CTV) contours, identify areas improvement, discuss future directions.MethodologyA literature review performed by utilizing key words "Deep Learning" AND ("Segmentation" OR "Delineation") "Clinical Target Volume" an indexed search into PubMed. A total 154 articles on criteria were reviewed. considered model used, disease site, targets contoured, guidelines utilized, overall performance.ResultsOf 53 investigating CTV, only 6 before 2020. Publications have increased years, 46 between 2020-2023. cervix (n=19) prostate (n=12) studied most frequently. Most studies (n=43) involved a single institution. Median sample size 130 patients (range: 5-1,052). common metrics utilized measure Dice similarity coefficient (DSC) followed Hausdorff distance. Dosimetric seldom reported (n=11). There also variability specific (RTOG, ESTRO, others). had good CTV multiple sites, showing DSC values >0.7. delineated faster compared manual contouring. However, some contours still required least minor edits, require improvement.ConclusionsDLAS demonstrates capability completing plans efficiency accuracy. developed validated institutions using developing institutions. about years. Future need include larger datasets different patient demographics, stages, validation multi-institutional settings, inclusion dosimetric performance. Manual directions. Of improvement.
Language: Английский
Citations
12Radiotherapy and Oncology, Journal Year: 2025, Volume and Issue: unknown, P. 110740 - 110740
Published: Jan. 1, 2025
Language: Английский
Citations
1Radiotherapy and Oncology, Journal Year: 2023, Volume and Issue: 182, P. 109555 - 109555
Published: Feb. 21, 2023
Language: Английский
Citations
22Radiotherapy and Oncology, Journal Year: 2023, Volume and Issue: 180, P. 109457 - 109457
Published: Jan. 3, 2023
Language: Английский
Citations
19Medical Physics, Journal Year: 2023, Volume and Issue: 50(5), P. 2625 - 2636
Published: Feb. 22, 2023
Abstract Background Stereotactic body radiation therapy (SBRT) of central lung tumors with photon or proton has a risk increased toxicity. Treatment planning studies comparing accumulated doses for state‐of‐the‐art treatment techniques, such as MR‐guided radiotherapy (MRgRT) and intensity modulated (IMPT), are currently lacking. Purpose We conducted comparison MRgRT, robustly optimized non‐adaptive IMPT, online adaptive IMPT tumors. A special focus was set on analyzing the to bronchial tree, parameter linked high‐grade toxicities. Methods Data 18 early‐stage tumor patients, treated at 0.35 T MR‐linac in eight five fractions, were analyzed. Three gated scenarios compared: (S1) (S2) (S3) IMPT. The plans recalculated reoptimized daily imaging data acquired during over all fractions. Accumulated dose‐volume histogram (DVH) parameters gross volume (GTV), lung, heart, organs‐at‐risk (OARs) within 2 cm target (PTV) extracted each scenario compared Wilcoxon signed‐rank tests between S1 & S2, S3. Results GTV D 98% above prescribed dose patients scenarios. Significant reductions ( p < 0.05) mean ipsilateral (S2: –8%; S3: –23%) heart –79%; –83%) observed both S1. tree 0.1cc significantly lower S3 (S1: 48.1 Gy; 39.2 = 0.005), but not different S2 45.0 0.094), smaller OARs 1–2 PTV 30.2 S2: 24.6 23.1 Gy), 1 PTV. Conclusions significant sparing potential MRgRT close, direct proximity identified. near‐maximum Online achieved MRgRT.
Language: Английский
Citations
13La radiologia medica, Journal Year: 2023, Volume and Issue: 128(10), P. 1250 - 1261
Published: Aug. 19, 2023
Language: Английский
Citations
11Journal of Applied Clinical Medical Physics, Journal Year: 2023, Volume and Issue: 24(7)
Published: March 15, 2023
Recently, target auto-segmentation techniques based on deep learning (DL) have shown promising results. However, inaccurate delineation will directly affect the treatment planning dose distribution and effect of subsequent radiotherapy work. Evaluation geometric metrics alone may not be sufficient for accuracy assessment. The purpose this paper is to validate performance automatic segmentation with dosimetric try construct new evaluation comprehensively understand dose-response relationship from perspective clinical application.A DL-based model was developed by using 186 manual modified radical mastectomy breast cancer cases. resulting DL were used generate alternative contours in a set 48 patients. Auto-plan reoptimized ensure same optimized parameters as reference Manual-plan. To assess impact auto-segmentation, only common but also spatial distance relative volume ( RV${R}_V$ ) used. Correlations performed Spearman's correlation between changes.Only strong (|R2 | > 0.6, p < 0.01) or moderate 0.4, Pearson established traditional metric three indices (conformity index, homogeneity mean dose). For organs at risk (OARs), inferior no significant found differences. Furthermore, we that OARs affected boundary error instead target.Current could reflect certain degree variation. find contour variations do lead dosimetry changes, clinically oriented more accurately how quality affects should constructed.
Language: Английский
Citations
10Physics and Imaging in Radiation Oncology, Journal Year: 2023, Volume and Issue: 27, P. 100454 - 100454
Published: June 6, 2023
Normal tissue sparing in radiotherapy relies on proper delineation. While manual contouring is time consuming and subject to inter-observer variability, auto-contouring could optimize workflows harmonize practice. We assessed the accuracy of a commercial, deep-learning, MRI-based tool for brain organs-at-risk delineation.Thirty adult tumor patients were retrospectively manually recontoured. Two additional structure sets obtained: AI (artificial intelligence) AIedit (manually corrected auto-contours). For 15 selected cases, identical plans optimized each set. used Dice Similarity Coefficient (DSC) mean surface-distance (MSD) geometric comparison gamma analysis dose-volume-histogram dose metrics evaluation. Wilcoxon signed-ranks test was paired data, Spearman coefficient(ρ) correlations Bland-Altman plots assess level agreement.Auto-contouring significantly faster than (1.1/20 min, p < 0.01). Median DSC MSD 0.7/0.9 mm 0.8/0.5 AIedit. correlated with size (ρ = 0.76, 0.01), higher large structures. pass rate 74% (71-81%) Plan_AI 82% (75-86%) Plan_AIedit, no correlation or MSD. Differences between Dmean_AI Dmean_Ref ≤ 0.2 Gy (p 0.05). The difference moderately DSC. Bland Altman plot showed minimal discrepancy (0.1/0) reference Dmean/Dmax.The AI-model good structures, but developments are required smaller ones. Auto-segmentation faster, minor differences distribution caused by variations.
Language: Английский
Citations
10Physica Medica, Journal Year: 2025, Volume and Issue: 130, P. 104896 - 104896
Published: Jan. 7, 2025
Language: Английский
Citations
0