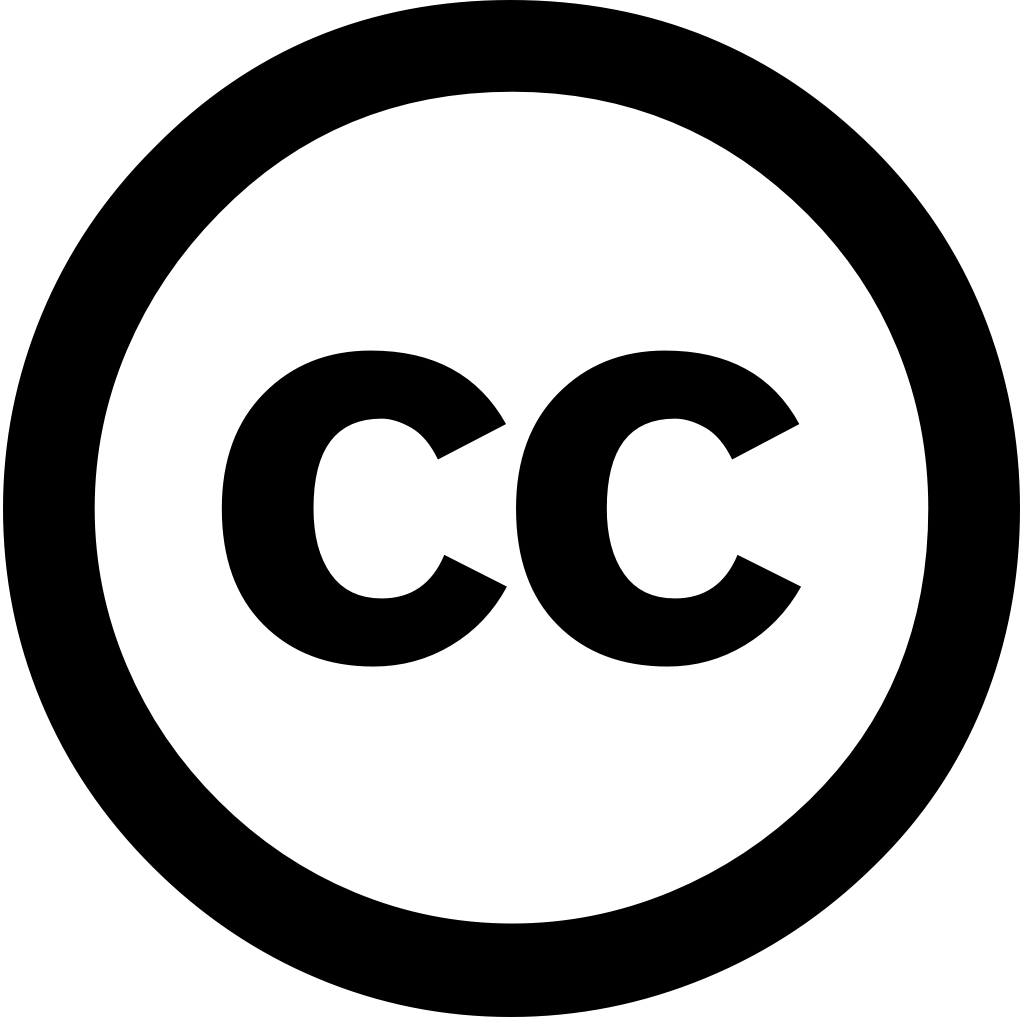
Research Square (Research Square), Journal Year: 2024, Volume and Issue: unknown
Published: Dec. 25, 2024
Language: Английский
Research Square (Research Square), Journal Year: 2024, Volume and Issue: unknown
Published: Dec. 25, 2024
Language: Английский
Scientific Reports, Journal Year: 2024, Volume and Issue: 14(1)
Published: Nov. 22, 2024
The use of time lapse systems (TLS) in In Vitro Fertilization (IVF) labs to record developing embryos has paved the way for deep-learning based computer vision algorithms assist embryologists their morphokinetic evaluation. Today, most literature characterized that predict pregnancy, ploidy or blastocyst quality, leaving side task identifying key events. Using a dataset N = 1909 collected from multiple clinics equipped with EMBRYOSCOPE/EMBRYOSCOPE+ (Vitrolife), GERI (Genea Biomedx) MIRI (Esco Medical), this study proposes novel architecture automatically detect 11 kinetic events (from 1-cell blastocyst). First, Transformer video backbone was trained custom metric inspired by reverse cross-entropy which enables model learn ordinal structure Second, embeddings were extracted and passed into Gated Recurrent Unit (GRU) sequence account dependencies. A weighted average 66.0%, 67.6% 66.3% timing precision, recall F1-score respectively reached on test set 278 embryos, applicable TLS.
Language: Английский
Citations
4Obstetrics Gynecology and Reproduction, Journal Year: 2025, Volume and Issue: unknown
Published: March 14, 2025
Given the increasing problem of infertility in Russian Federation, assisted reproductive technologies (ART) have proven to be one most effective treatments for this condition. Notably, introduction ART methods, particularly vitro fertilization (IVF), has led markedly increased birth rates over past two decades. Studies show that machine learning algorithms can process images embryos assess their quality, thus facilitating selection viable among them transfer. There are ethical and technical barriers hindering widespread adoption artificial intelligence (AI) clinical practice, including concerns data privacy as well a need train specialists deal with new technologies. AI analyze vast amounts data, medical histories research results, more accurately predict pregnancy outcomes. This enables doctors make justified decisions. In future, will able patient efficiently, helping identify causes at earlier stages.
Language: Английский
Citations
0Biomedical Signal Processing and Control, Journal Year: 2025, Volume and Issue: 107, P. 107841 - 107841
Published: March 28, 2025
Language: Английский
Citations
0Journal of Assisted Reproduction and Genetics, Journal Year: 2024, Volume and Issue: 42(1), P. 3 - 14
Published: Oct. 14, 2024
Language: Английский
Citations
2Archives of Medical Research, Journal Year: 2024, Volume and Issue: 55(8), P. 103131 - 103131
Published: Nov. 29, 2024
Language: Английский
Citations
1Mayo Clinic Proceedings Digital Health, Journal Year: 2024, Volume and Issue: 2(4), P. 518 - 532
Published: Aug. 27, 2024
The aim of this systematic review was to identify clinical decision support algorithms (CDSAs) proposed for assisted reproductive technologies (ARTs) and evaluate their effectiveness in improving ART cycles at every stage vs traditional methods, thereby providing an evidence-based guidance use practice. A literature search on PubMed Embase articles published between 1 January 2013 31 2024 performed relevant articles. Prospective retrospective studies English the CDSA were included. Out 1746 screened, 116 met inclusion criteria. selected categorized into 3 areas: prognosis patient counseling, management, embryo assessment. After screening, 11 CDSAs identified as potentially valuable management laboratory practices. Our findings highlight potential automated aids improve vitro fertilization outcomes. However, main limitation lack standardization validation methods across studies. Further trials are needed establish these tools setting.
Language: Английский
Citations
0Research Square (Research Square), Journal Year: 2024, Volume and Issue: unknown
Published: Dec. 25, 2024
Language: Английский
Citations
0