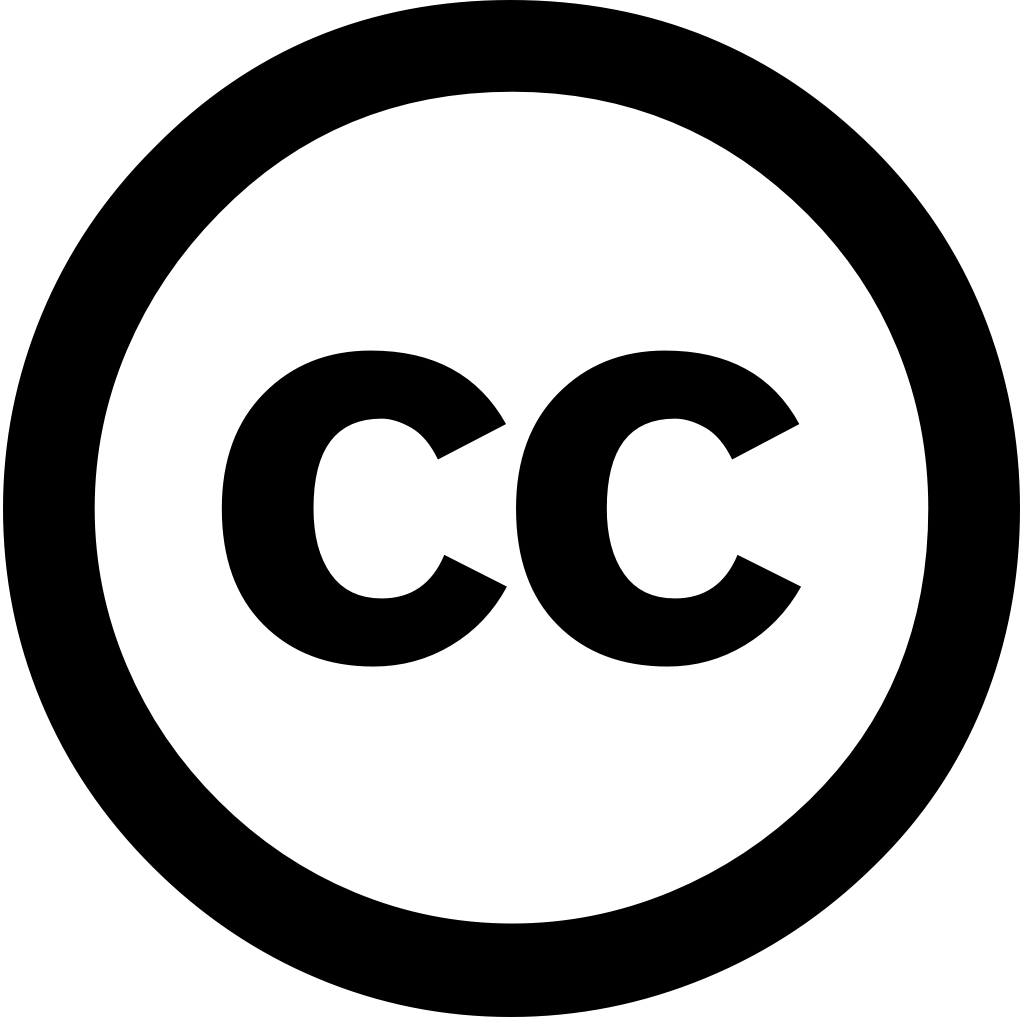
Fuel, Journal Year: 2024, Volume and Issue: 384, P. 133953 - 133953
Published: Dec. 6, 2024
Language: Английский
Fuel, Journal Year: 2024, Volume and Issue: 384, P. 133953 - 133953
Published: Dec. 6, 2024
Language: Английский
Forests, Journal Year: 2024, Volume and Issue: 15(3), P. 456 - 456
Published: Feb. 29, 2024
The estimation of forest above-ground biomass (AGB) can be significantly improved by leveraging remote sensing (RS) and deep learning (DL) techniques. In this process, it is crucial to obtain appropriate RS features develop a suitable model. However, traditional methods such as random (RF) feature selection often fail adequately consider the complex relationships within high-dimensional spaces. Moreover, challenges related parameter overfitting inherent in DL models may compromise accuracy AGB estimation. Therefore, study proposes novel framework based on freely available Sentinel-1 synthetic aperture radar (SAR) Sentinel-2 optical data. Firstly, we designed new indices through formula analogous with vegetation index calculation integrate multidimensional spectral structural information. Then, simplicity computational principles, pigeon-inspired optimization algorithm (PIO) was introduced into bi-directional long short-term memory neural network (PIO-BiLSTM), which achieved set objective function repeated iteration validation optimal model parameters. Finally, verify framework’s effect, conducted experiments two different tree species compared another seven classical algorithms machine models. results indicated that inversion all both categories, PIO-BiLSTM highest (Category-1: R2 = 0.8055, MAE 8.8475 Mg·ha−1, RMSE 12.2876 relative 18.1715%; Category-2: 0.7956, 1.7103 2.2887 9.3000%). Compared existing methods, proposed greatly reduced labor costs selection, its potential uncertainty also decreased up 9.0%. Furthermore, method has strong generalization ability independent species, indicating great for future wider regions diverse types.
Language: Английский
Citations
3Industrial Crops and Products, Journal Year: 2024, Volume and Issue: 215, P. 118627 - 118627
Published: May 9, 2024
Language: Английский
Citations
3Ecological Informatics, Journal Year: 2024, Volume and Issue: 82, P. 102684 - 102684
Published: June 13, 2024
The species Pinus radiata is highly invasive in native forests Chile, drastically affecting the functioning and structure of ecosystems. Hence, it imperative to develop robust approaches detect P. invasions at different scales. Models based on convolutional neural networks (CNN) have proven be a promising alternative plant high-resolution remote sensing data, such as those obtained by drones. However, studies been limited their spatial variability assessments transferability or transfer learning new sectors, hindering ability use these models real-world setting. We train CNN architectures using unpiloted aerial vehicle data evaluate outside training domain regression approaches. compared trained with low (mono-site) high (multi-site). further sought maximize transference searching among models, maximizing evaluation an independent set. results showed that better when multi-site higher are used for training, obtaining coefficient determination R2 between 60% 87%. On contrary, mono-site present wide performance attributed dissimilarity information sites, limiting possibilities extrapolations model generalizations. also significant difference within-domain generalization test versus domain, showing testing alone cannot depict discrepancy without data. Finally, best domains often do not agree selected standard training/validation/testing scheme. Our findings pave way deeper discussions investigations into limitations applied imagery.
Language: Английский
Citations
3Alexandria Engineering Journal, Journal Year: 2024, Volume and Issue: 105, P. 423 - 436
Published: Aug. 9, 2024
Overheating of power transformers, low-voltage panels, and medium-voltage components in Electric Transformer Rooms (ETRs) can result from various factors, such as contact issues, irregular loads, other similar problems. Thermal imaging shows significant potential for detecting faults equipment. However, its effectiveness is hindered by the complex thermal patterns variability equipment environmental conditions, making accurate fault detection challenging. This paper aims to study transfer learning architectures automating classification ETRs. work applies four architectures: AlexNet, SqueezeNet, VGG19, GoogLeNet. The findings testing phase demonstrated that use fine-tuning pre-trained convolutional neural networks was highly effective images captured ETRs, with models achieving accuracy rates between 86.98% 100%, F 1 -Scores 86.79% 100%. • Transfer applied automate A dataset set up augmented improve generalization abilities proposed models. Establish standard comparisons among architectures.
Language: Английский
Citations
3Remote Sensing, Journal Year: 2024, Volume and Issue: 16(16), P. 2929 - 2929
Published: Aug. 9, 2024
Accurate livestock counts are essential for effective pastureland management. High spatial resolution remote sensing, coupled with deep learning, has shown promising results in detection. However, challenges persist, particularly when the targets small and a heterogeneous environment, such as those African rangelands. This study evaluated nine state-of-the-art object detection models, four variants each from YOLOv5 YOLOv8, Faster R-CNN, detecting cattle 10 cm aerial RGB imagery Kenya. The experiment involved 1039 images 9641 labels training sites varying land cover characteristics. trained models were on 277 2642 test dataset, their performance was compared using Precision, Recall, Average Precision (AP0.5–0.95). indicated that reduced resolution, dense shrub cover, shadows diminish model’s ability to distinguish background. YOLOv8m architecture achieved best AP0.5–0.95 accuracy of 39.6% Recall 91.0% 83.4%, respectively. Despite its superior performance, had highest counting error −8%. By contrast, YOLOv5m 39.3% attained most accurate count RMSE 1.3 R2 0.98 variable herd densities. These highlight model high may struggle accurately. Nevertheless, these findings suggest potential upscale aerial-imagery-trained satellite conducting censuses over large areas. In addition, will support sustainable management by ensuring stock numbers do not exceed forage available grazing, thereby mitigating overgrazing.
Language: Английский
Citations
3Geoderma, Journal Year: 2024, Volume and Issue: 450, P. 117056 - 117056
Published: Oct. 1, 2024
Language: Английский
Citations
1Science of Remote Sensing, Journal Year: 2024, Volume and Issue: 11, P. 100185 - 100185
Published: Dec. 12, 2024
Language: Английский
Citations
1Journal of Marine Science and Engineering, Journal Year: 2024, Volume and Issue: 12(11), P. 1917 - 1917
Published: Oct. 27, 2024
Efficient and accurate fishery forecasting is of great significance in ensuring the efficiency operations. This paper proposes a method using brightness temperature (TB) time series spatial feature extraction fusion model. Using Indian Ocean bigeye tuna data from 2009 to 2021 as reference, this discusses feasibility FY-3 Microwave Radiation Imager (MWRI) Level 1 TB data. For paper, we designed deep learning network model for radiometer (TimeTB-FishNet) based on Convolutional Neural Network (CNN), Gated Recurrent Unit (GRU), Attention mechanism. After expanding dimensions features, uses them together with spatiotemporal factors (year, month, longitude, latitude) features. By adding GRU CNN, CNN-GRU-Attention architecture established can extract features achieve best results. In validation experiments, TimeTB-FishNet performed optimally, coefficient determination (R2) 0.6643. generalization R2 also reached 0.6261, root mean square error (RMSE) 46.6031 kg/1000 hook. When sea surface height (SSH) was introduced, further 0.6463, lower RMSE 45.1318 The experimental results show that proposed are feasible effective. directly use enhanced without relying lagging ocean environmental product data, performing temporal forecasting. provide reference fishing Ocean.
Language: Английский
Citations
0Smart Agricultural Technology, Journal Year: 2024, Volume and Issue: 9, P. 100643 - 100643
Published: Nov. 12, 2024
Language: Английский
Citations
0Ecological Indicators, Journal Year: 2024, Volume and Issue: 169, P. 112932 - 112932
Published: Dec. 1, 2024
Language: Английский
Citations
0