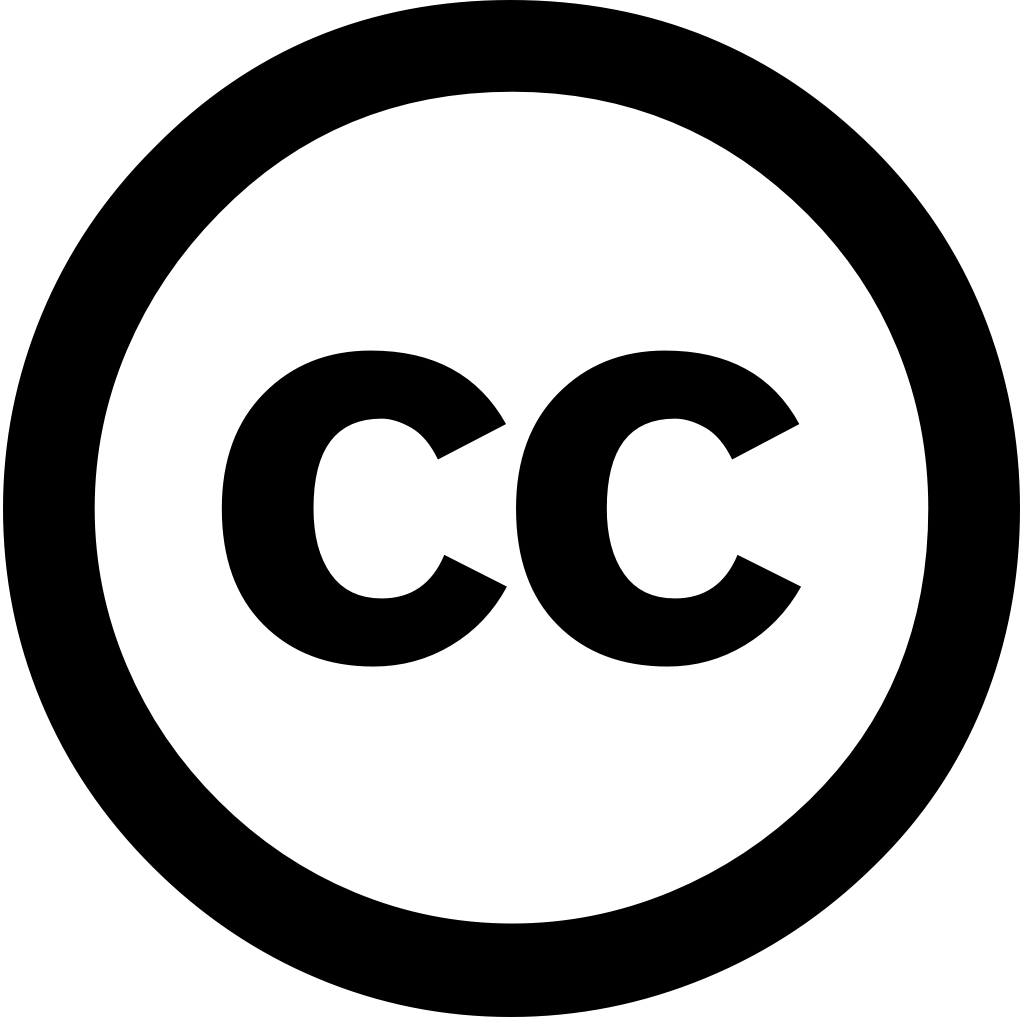
Frontiers in Marine Science, Journal Year: 2023, Volume and Issue: 10
Published: Nov. 30, 2023
Zooplankton play a crucial role in the ocean’s ecology, as they form foundational component food chain by consuming phytoplankton or other zooplankton, supporting various marine species and influencing nutrient cycling. The vertical distribution of zooplankton ocean is patchy, its relation to hydrographical conditions cannot be fully deciphered using traditional net casts due large depth intervals sampled. Lightframe On-sight Keyspecies Investigation (LOKI) concentrates with that leads flow-through chamber camera taking images. These high-resolution images allow for determination taxa, often even genus level, and, case copepods, developmental stages. Each cruise produces substantial volume images, ideally requiring onboard analysis, which presently consumes significant amount time necessitates internet connectivity access EcoTaxa Web service. To enhance analyses, we developed an AI-based software framework named DeepLOKI, utilizing Deep Transfer Learning Convolution Neural Network Backbone. Our DeepLOKI can applied directly on board. We trained validated model pre-labeled from four cruises, while fifth were used testing. best-performing model, self-supervised pre-trained ResNet18 Backbone, achieved notable average classification accuracy 83.9%, surpassing regularly frequently method (default) this field factor two. In summary, tool pre-sorting black white high accuracy, will simplify quicken final annotation process. addition, provide user-friendly graphical interface efficient concise processes leading up stage. Moreover, performing latent space analysis Backbone could prove advantageous identifying anomalies such deviations image parameter settings. This, turn, enhances quality control data. methodology remains agnostic specific imaging end system used, Loki, UVP, ZooScan, long there sufficient appropriately labeled data available enable effective task performance our algorithms.
Language: Английский